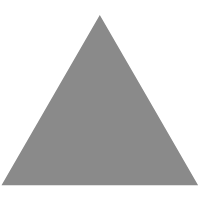
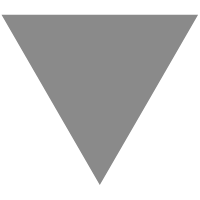
Snowflake for Data Science | Slalom Technology
source link: https://medium.com/slalom-technology/architecting-data-science-applications-on-snowflake-dce906f1e387
Go to the source link to view the article. You can view the picture content, updated content and better typesetting reading experience. If the link is broken, please click the button below to view the snapshot at that time.
Architecting Data Science Applications on Snowflake
An approach to addressing the challenges of the machine learning lifecycle and designing enterprise-scale solutions using the Snowflake and AWS platforms.

As machine learning (ML) adoption continues to grow, delivering more sophisticated insights across industries, it has become increasingly important for companies to develop capabilities that maximize the value of data within their organizations. Unlocking value in this modern analytics paradigm requires an adaptive platform with the ability to leverage data in a way that supports evolving business use cases.
The Snowflake Data Cloud offers a range of native platform tools and extensibility features to meet this growing need. Throughout the course of this article, we’ll explore how these features can address common challenges within the ML lifecycle and create enterprise scale data science applications on the Snowflake platform.
Using example reference architectures, design patterns, and best practices based on real-world experience and conversations with Snowflake, we’ll dive deeper into:
- Data collection (secure data sharing): Snowflake’s zero-copy data share offering allows organizations to instantly share data with other accounts, eliminating the need for a traditional ingestion process.
- Feature engineering (Snowpark): Snowpark allows users to write commands to Snowflake’s elastic query engine using languages including Java, Scala, and Python (private preview).
- Feature engineering (streams, tasks, and stored procedures): When used in conjunction, these built-in features of the Snowflake platform allow users to develop CI/CD pipelines and automate various tasks within the ML lifecycle.
- Modeling and deployment (external stages, functions, and tables): These features allow Snowflake users to access a rich partner ecosystem and develop custom applications, bringing artificial intelligence (AI) and ML capabilities to the platform.
- Performance evaluation (Snowsight dashboards): As a visualization interface within the Snowflake console, this tool allows users to create query-based charts and graphs for monitoring and reporting.
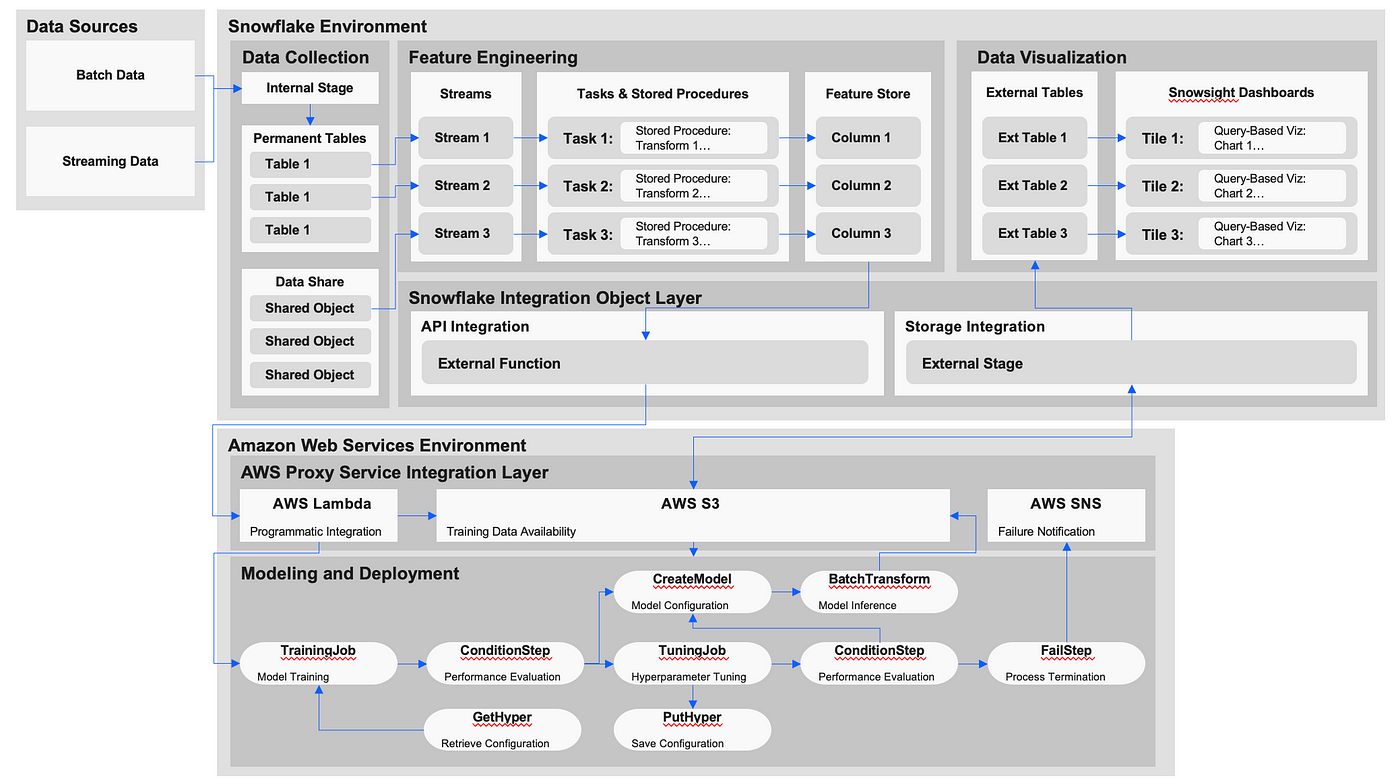
Snowflake data science reference diagram
Data collection
As data platforms expand to meet the increasing volume and velocity of data generated by relevant producers, the complexity of the data ingestion process tends to grow as well. Mounting dependencies create pressure on both the platform and organization, often constraining capabilities and limiting realized value.
Snowflake’s data sharing offering provides a solution to this by allowing two or more Snowflake customers to leverage the platform’s multi-layer architecture. Consumers are granted access to data in a decoupled storage layer while owners maintain full control of governance through access policy definitions made in the metadata services layer. While the platform still has data load options, including the CLI-based SnowSQL and a connector ecosystem with support for Kafka, Spark, and Python, this option effectively eliminates the traditional data ingestion process, streamlining the workflows of various roles within the development team.
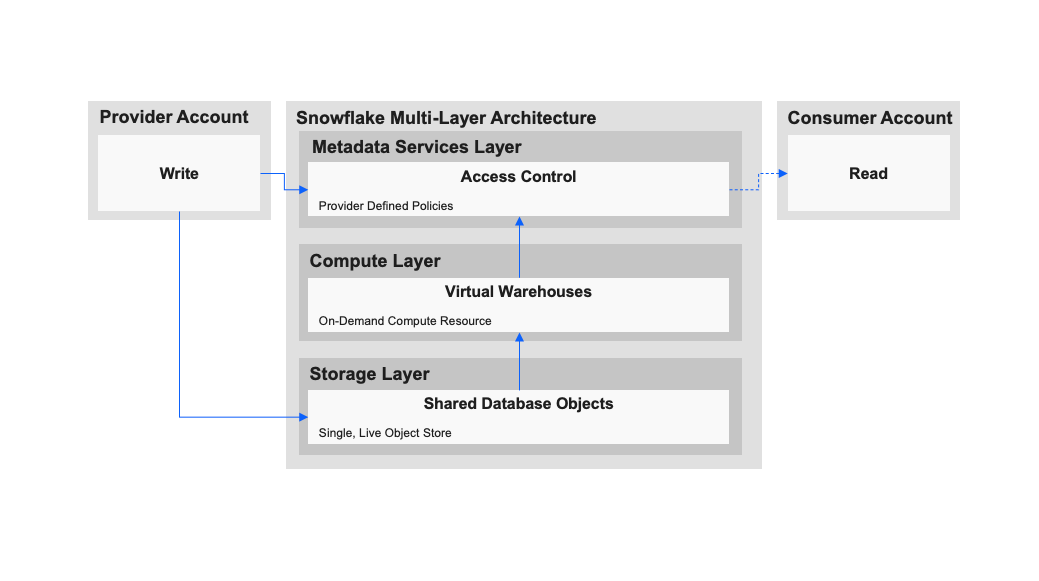
Example of data sharing on Snowflake
Feature engineering
Data rarely lands within the platform in a format suitable to be processed by an ML application. Instead, raw data commonly finds itself in an extract, transform, load (ETL) or extract, load, transform (ELT) pipeline where it is enriched prior to predictive modeling. In practice, these pipelines can often grow beyond manageable scope, warranting a workflow orchestration tool or a form of modularization. Even then, processes can still suffer from inefficiencies and inconsistencies related to repetitive computation.
One method of addressing these concerns is by implementing a feature store: a consistent, curated collection of transformed data created for shared use across multiple teams and ML models. These reusable features reduce the amount of repeated processing required in ML applications, accelerating and standardizing development. When designing a feature store, organizations should ask themselves:
- Who are and how will downstream consumers interact with this data?
- What do the frequency and usage patterns look like for this data?
- What underlying source dependencies exist and how does this affect feature design?
- What are the appropriate data structures and storage options for this data?
- What relationships and what reference strategies are most appropriate for this data?
To illustrate how this architecture can be implemented using the Snowflake platform, a reference diagram and sample code have been included. This pattern leverages Snowflake’s native streams, tasks, and stored procedures in combination with Snowpark to prepare data for use in ML.
- Stream: A row-level change data capture object that can be created on top of another database object, such as a table or view. These can be used to track and store changes in underlying dependencies for consumption by tasks in downstream transformation processes.
- Task: A scheduling tool that can be used to create process hierarchies and execute predefined statements. Snowflake users can nest stored procedures within these to create automated data pipelines.
- Stored procedure: A logical execution statement that can be written using tools including SQL, Javascript, and Snowpark.
- Snowpark: an application programming interface (API) library allows users to create DataFrame abstractions over Snowflake objects, facilitating dynamic transformations.
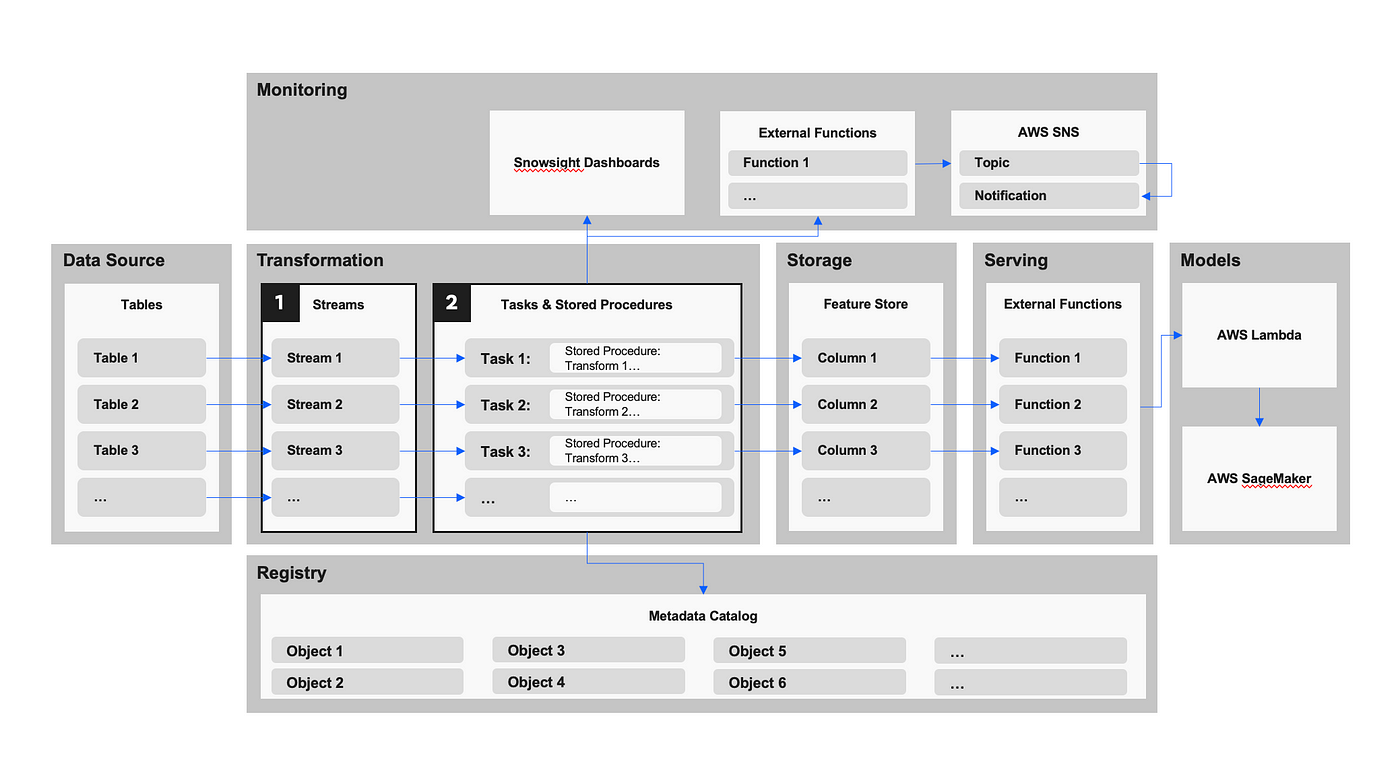
Feature store reference diagram.
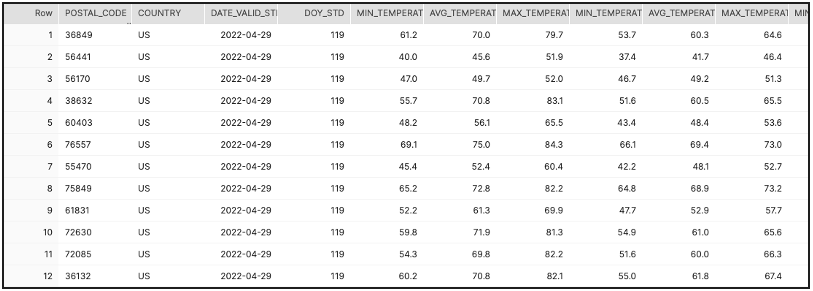
Raw weather data accessed via a data share stored in traditional tabular format

Defines a stream object for change data capture
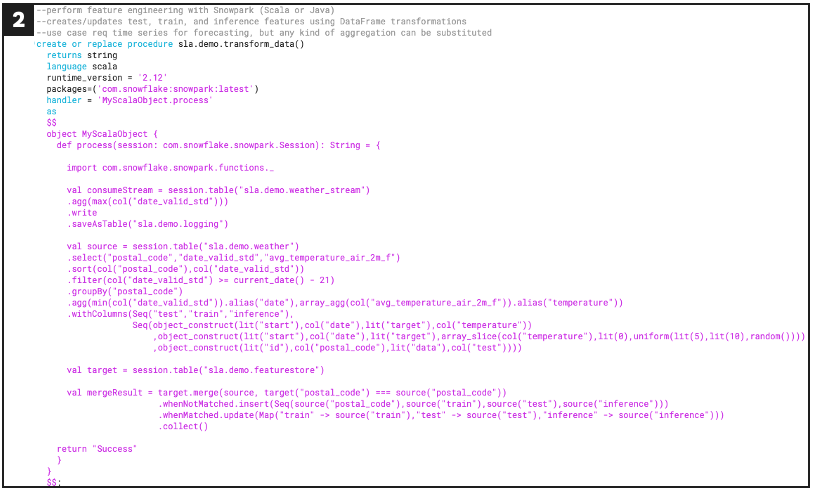
Defines a Snowpark stored procedure for data transformation

Defines a task for scheduling execution
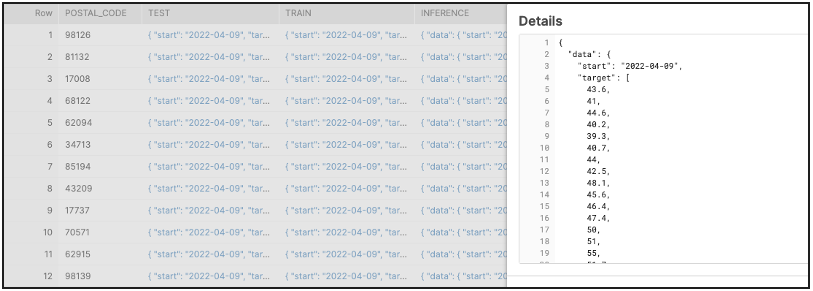
Data transformed by the stored procedure above is now prepared for consumption by an ML application
Modeling and deployment
Even with a reusable semantic data layer in place, organizations still have a long way to go between inception and value delivery in ML applications. There is a significant cost of ownership to building, deploying, and maintaining models that is shared by data scientists, ML engineers, DevOps engineers, and others across the organization. As applications mature, development and maintenance requirements become more difficult to fulfill. Without proper management, this often leads to the creation of technical debt within the organization.
Snowflake’s external functions provide the platform-agnostic feature extensibility organizations need to access a rich ecosystem of ML solutions. Organizations may also benefit from the optionality provided by this architecture; as the operational context changes, so too can the components of their technical infrastructure.
In this section, we’ll focus on connecting to the Amazon Web Services (AWS) platform and the SageMaker ML service using Snowflake integration objects and external stages, tables, and functions. The following reference diagram and code examples illustrate an approach toward integrating the two platforms, allowing users to leverage a variety of resources to generate ML predictions:
- Integration objects: These Snowflake objects store the information required to enable external platform connections including access keys, identity access management configurations, and Amazon resource identifiers.
- External stage: A file-based Snowflake abstraction created over a storage location on another platform, creating a shared repository on AWS S3.
- External function: A Snowflake abstraction created over an API hosted on another platform, allowing users to communicate with the SageMaker platform through AWS API Gateway and Lambda.
- External table: A table-based Snowflake abstraction created over a storage location on another platform, creating a tabular representation of inference data hosted on AWS S3.
- SageMaker pipeline: A CI/CD tool for orchestrating ML workflows that facilitates the automation of tasks — including model training, tuning, evaluation, and inference — helping developers manage ML solutions at scale.
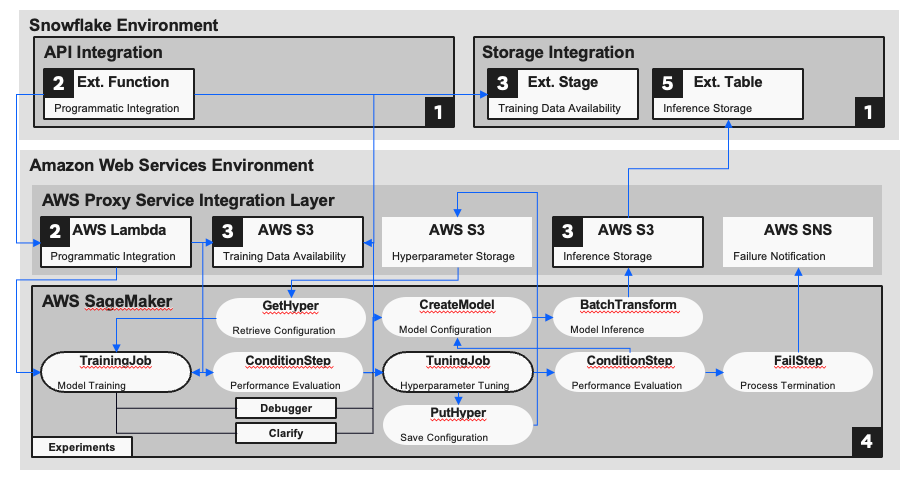
Integrated ML reference diagram
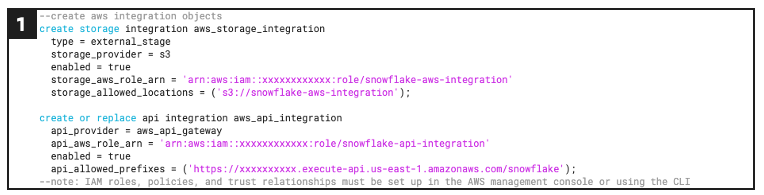
Defines storage and API integration objects

Defines AWS Lambda external function using API integration
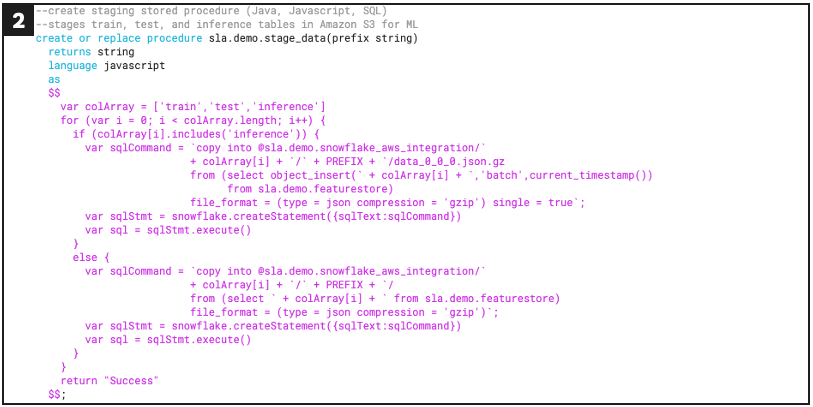
Defines stored procedure for staging data
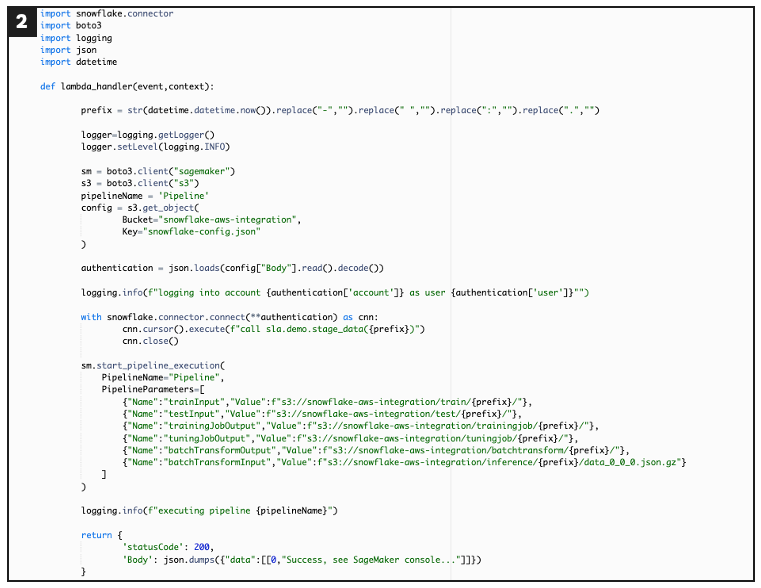
Defines AWS Lambda function for staging data and starting pipeline execution

Defines AWS S3 external stage using storage integration
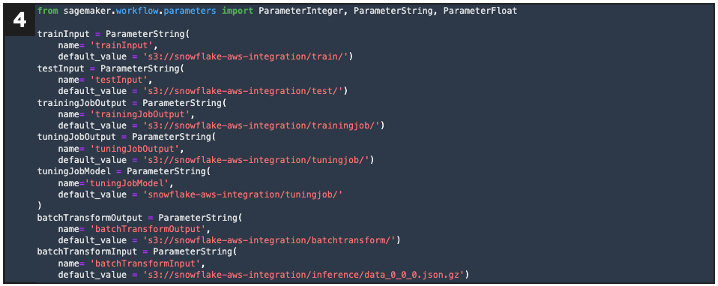
Defines SageMaker pipeline execution parameters

Defines a step to get saved hyperparameters using AWS Lambda
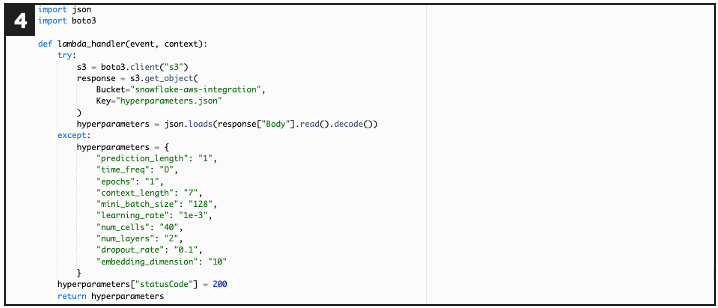
Defines AWS Lambda to get saved hyperparameters from AWS S3 storage
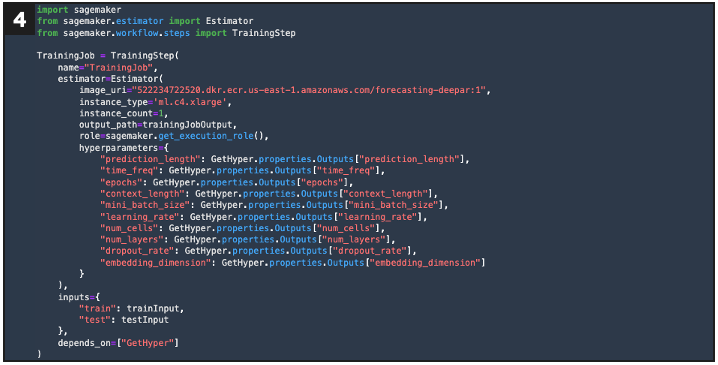
Defines a step for model training
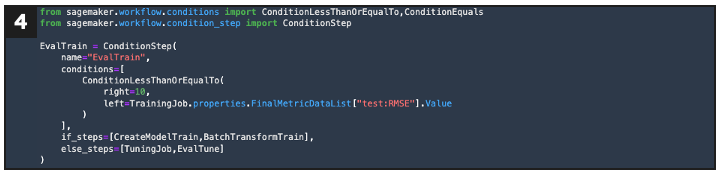
Defines a step that evaluates model performance against a specified threshold to determine next actions
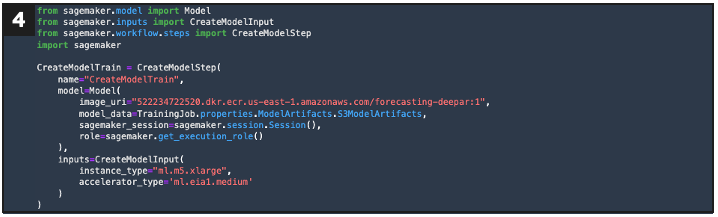
Defines a step that creates a model configuration
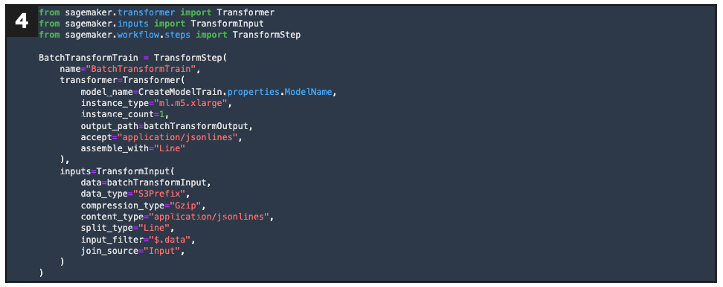
If evaluation = pass, this defines an inference step using the training model configuration
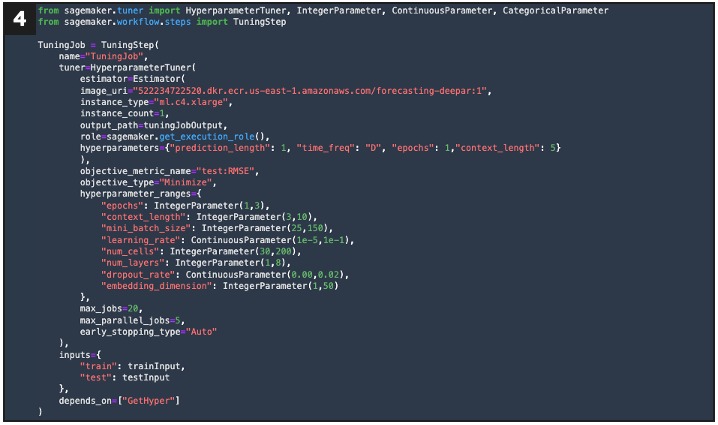
If evaluation = fail, this defines a retraining step that creates a new hyperparameter tuning configuration

If evaluation = fail, this defines a second performance evaluation step that determines next actions
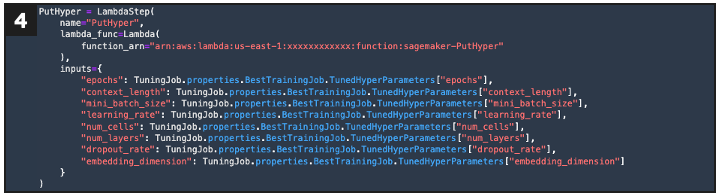
If new evaluation = pass, this defines a step that puts the new hyperparameter configuration to S3 using Lambda
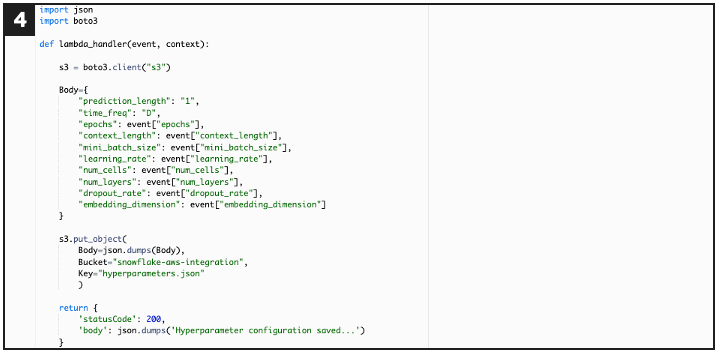
Defines AWS Lambda to save new hyperparameter configuration to S3
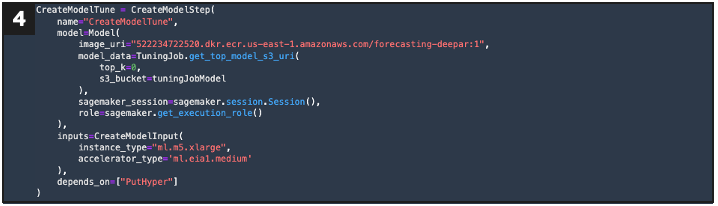
If new evaluation = pass, this defines a step that creates a model configuration
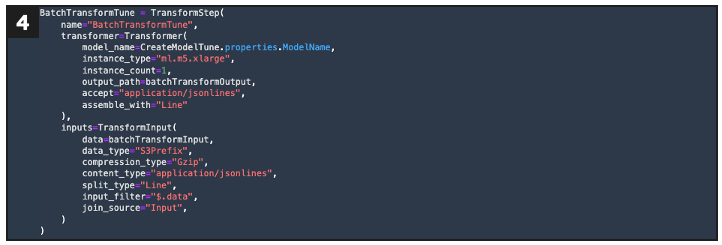
If new evaluation = pass, this defines an inference step using the tuning model configuration

If new evaluation = fail, this defines a step that notifies developers of a failure using AWS SNS via Lambda
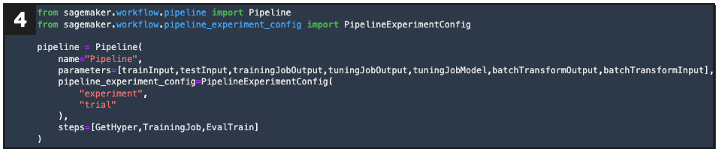
Defines pipeline object for programmatic reference
By automating traditionally slow, manual processes, these tools increase the velocity with which teams can deliver and scale ML products while reducing complexity. This pattern decomposes traditionally monolithic ML applications by introducing a loosely coupled micro-services architecture, simplifying both development and deployment. The customizable pipeline object defined above streamlines various responsibilities within the data science workflow and its execution results in one of three outcomes:
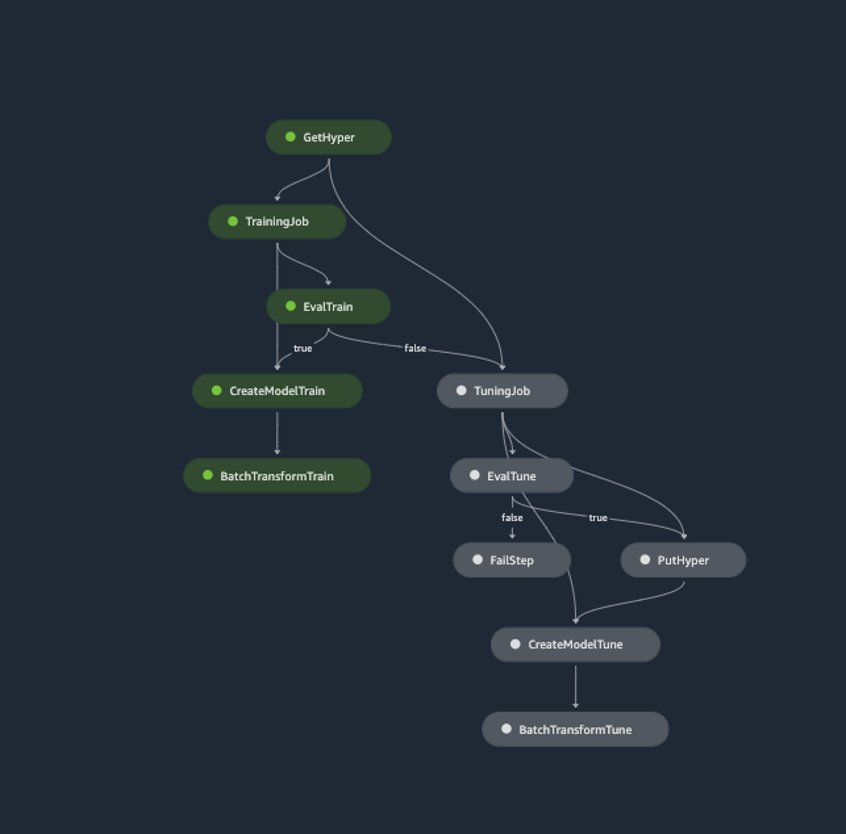
A training job passes evaluation and is used for inference
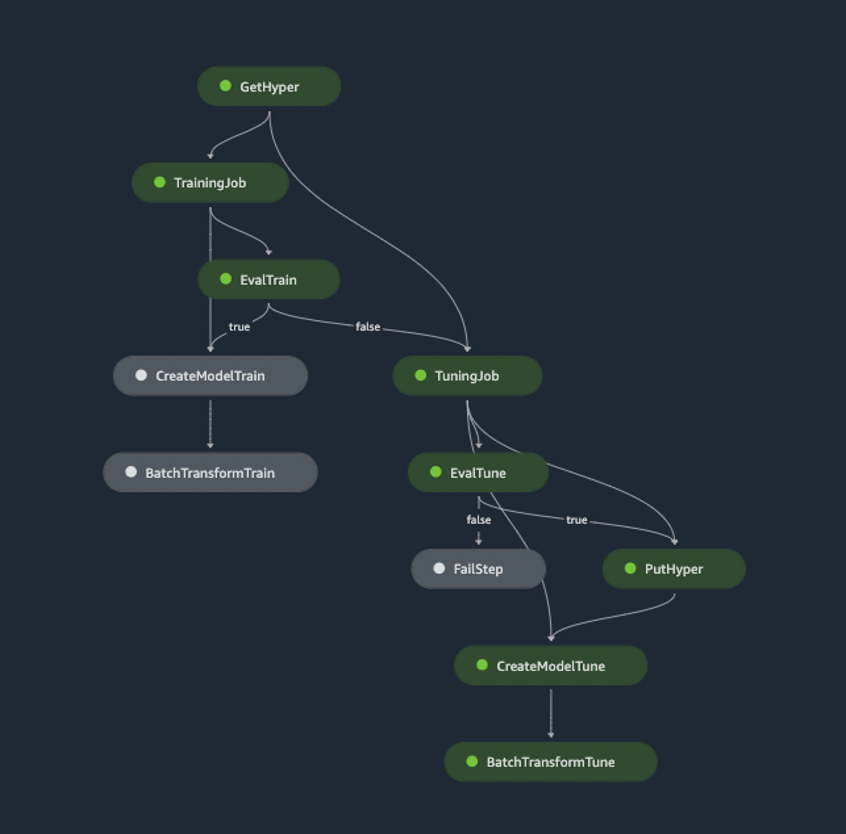
A training job fails evaluation, a tuning job passes evaluation and is used for inference
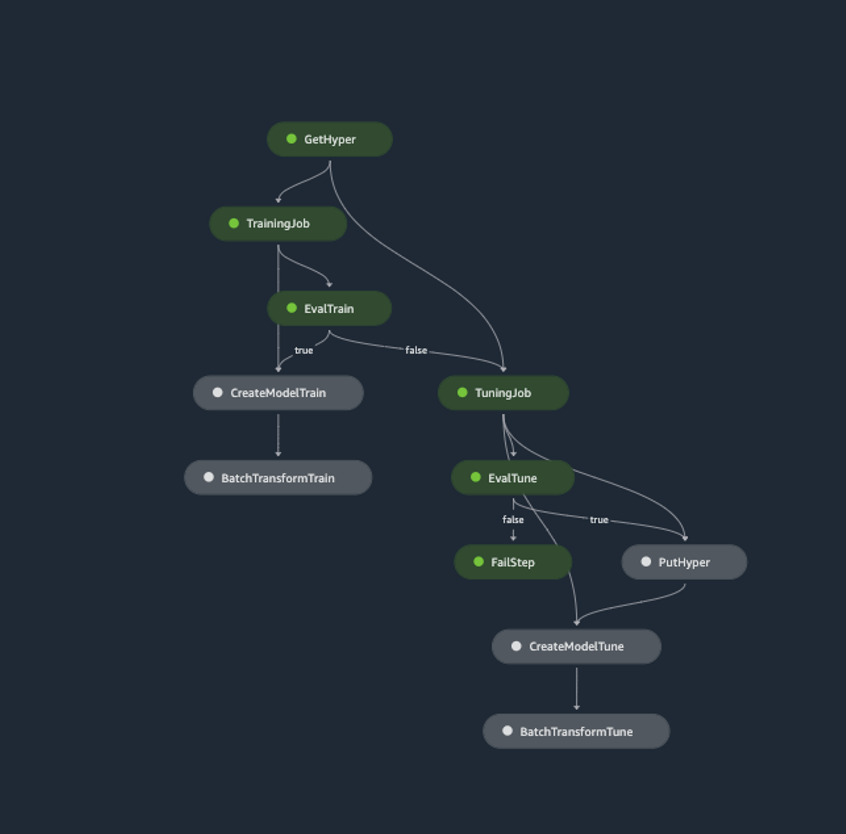
Both training and tuning jobs fail to pass evaluation and a failure notification is distributed via SNS
Depending on the deployment strategy, the inference output of a pipeline can be re-ingested in the following ways:
- Batch transform: The model output is stored in S3 as a flat file so a Snowflake external table can be used to provide a tabular abstraction over the file for further downstream processing.
- Endpoint deployment: The model output is returned as a query result in response to the execution of an external function. This can be persisted in a Snowflake internal table or other data structure appropriate for the use case.
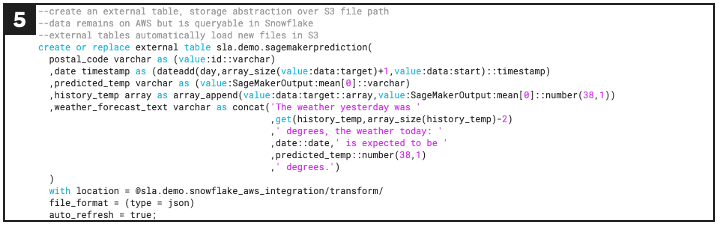
Defines an external table for ingesting batch inferences.
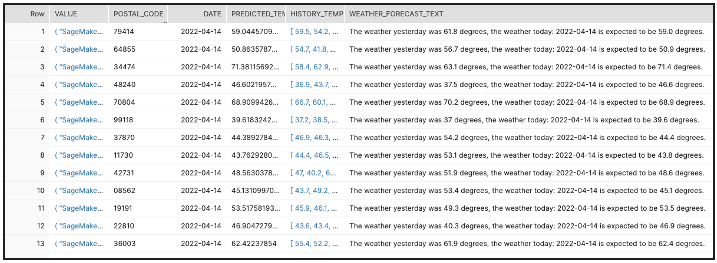
An external table with relevant information parsed from the inference response object
Pipelines can also be integrated with other features of the SageMaker platform that build on this architecture to further simplify the ML process, including:
- SageMaker Experiments: These provide a logical structure for tracking the performance components of the ML workflow including training jobs, tuning jobs, and inference requests over time.
- SageMaker Clarify: Clarify monitors data for bias and allows users to configure actions in response to the results of the evaluation.
- SageMaker Debugger: Debugger monitors model resource performance, helping developers identify bottlenecks and automatically perform actions in response.
- AWS Simple Notification Service (SNS): SNS is a pub-sub tool that can be used to send emails, texts, and other notifications in response to a change in the status of a pipeline.
Data visualization
With solution infrastructure in place, organizations must turn their attention toward performance evaluation. Monitoring and reporting impose challenges related to reliability and accessibility of information, and a failure to properly address these concerns poses a risk of rendering the organization rudderless. Without the ability to gauge and communicate the degree to which success criteria is met, product teams will have trouble quantifying ROI and capitalizing on opportunities for improvement. This hampers effectiveness and limits the ability of solutions to realize their full potential.
Snowsight Dashboards provide an on-platform option to meet the need for a reporting solution, enabling users to extract valuable insights using embedded data visualization capabilities. Query-based tiles include support for aggregation and filtering operations, allowing users to define custom logic behind visualizations. In this SQL-driven architecture, a dashboard consisting of many tiles acts as a visual representation of the individual queries made against the underlying source data, allowing users to consolidate tasks within the data visualization process. Support for dashboard sharing between Snowflake users simplifies distribution, encouraging process transparency and data democratization amongst stakeholders.
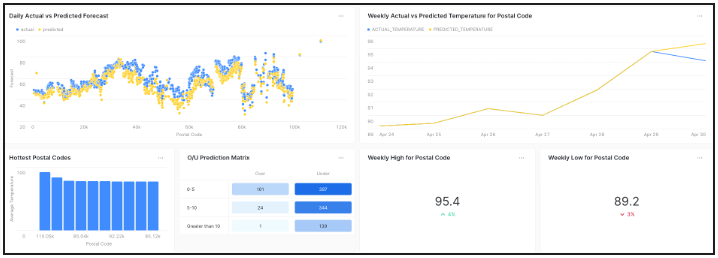
Snowsight dashboard for visualizing model inferences
Conclusion
Emerging trends in ML continue to redefine how organizations leverage data, shifting the basis of competition toward modern, data-driven applications. Activating these capabilities can pose a significant challenge to organizations. Platforms like Snowflake can help enterprises abstract away complexity and develop scalable data science platforms that enable digital transformation.
Recommend
About Joyk
Aggregate valuable and interesting links.
Joyk means Joy of geeK