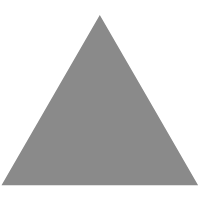
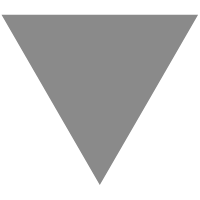
【丢弃】【NLP1】词袋
source link: https://www.guofei.site/2018/09/20/nlp1.html
Go to the source link to view the article. You can view the picture content, updated content and better typesetting reading experience. If the link is broken, please click the button below to view the snapshot at that time.
【丢弃】【NLP1】词袋
2018年09月20日Author: Guofei
文章归类: ,文章编号: 301
版权声明:本文作者是郭飞。转载随意,但需要标明原文链接,并通知本人
原文链接:https://www.guofei.site/2018/09/20/nlp1.html
回收原因:tensorflow相关的包将被移除,有些功能用sklearn更加好读
词向量转序号向量的功能,sklearn也没有提供,我在另一篇博客写了一个类取完成这样的功能
词袋
词袋(Bag of Words)
先有一个常用词组成单词向量,非常用词的序号统一设定为0
对于每一个句子,每个单词对应的索引设定为1
例如,我们的词袋为
['others','TensorFlow','makes','machine','learning','easy']
词袋的计算关系为
sentence1='TensorFlow makes machine learning easy'
sentence1_list=[0,1,1,1,1,1]
sentence2='Machine learning is easy'
sentence2=[1,0,0,1,1,1]
# 这里,'is'没有出现在单词向量中,为0号
step1获取数据
import pandas as pd
df=pd.read_csv('http://www.guofei.site/datasets_for_ml/SMSSpamCollection/SMSSpamCollection.csv',sep='\t',header=None,names=['label','sentences'])
Y=(df.label=='spam')*1
step2初步清洗
import re
regex=re.compile('[a-zA-Z]+')
X1=[regex.findall(sentence) for sentence in df.sentences] # 只挑出单词,去除标点符号和数字
X2=[[i.lower() for i in j if len(i)>1] for j in X1] # 改成小写,并剔除单个字母
X=[' '.join(i) for i in X2] # 重新整合成句子
from sklearn.model_selection import train_test_split
X_train, X_test, Y_train, Y_test = train_test_split(X, Y, test_size=0.2)
step3:使用TensorFlow做词袋
import tensorflow as tf
from tensorflow.contrib import learn
vocab_processor=learn.preprocessing.VocabularyProcessor(max_document_length=25,min_frequency=3) # max_document_length 是每个句子最大的长度,短的补空值,长的截取
vocab_processor.fit(X)
embedding_size=len(vocab_processor.vocabulary_) # 词典向量的长度
# 使用案例:
vocab_processor.transform(['hello world','good morning']) # 返回一个generator,每个next对应入参中的一项(入参也必须是<iterable>)
# 每项是一个与入参对应项大小相同的向量,向量的值是每个word在vocab_processor.vocabulary_中的序号
# 例如,案例返回[237 327 0 0 0 0 0 0 0 0 0 0 0 0 0 0 0 0 0 0 0 0 0 0 0]
# 前两个数字分别是'hello', 'world' 在 vocab_processor.vocabulary_中的位置,后面的0是用'其它'补到25
# vocab_processor.fit_transfrom(X)
step4:定义占位符
import tensorflow as tf
sess = tf.Session()
identity_mat = tf.diag(tf.ones(shape=[embedding_size]))
A = tf.Variable(tf.random_normal(shape=(embedding_size, 1)))
b = tf.Variable(tf.random_normal(shape=[1, 1]))
x = tf.placeholder(shape=[25], dtype=tf.int32) # 序号组成的向量,将来用vocab_processor.transform()来feed
y = tf.placeholder(shape=[1, 1], dtype=tf.float32)
x_embed = tf.nn.embedding_lookup(identity_mat, x)
# 用法解释:
# sess.run(x_embed,feed_dict={x:next(vocab_processor.transform(['hello world']))})
# 一个shape=(max_document_length,vocab_processor.vocabulary_)的向量,第i行是第i个单词的OneHot编码
x_col_sum=tf.reduce_sum(x_embed,axis=0) # 上一步是每个单词的OneHot编码,现在加总成句子的OneHot编码
x_col_sum_2D=tf.expand_dims(x_col_sum,axis=0)
# 下面做softmax
a=tf.matmul(x_col_sums_2D,A)+b
y_hat=tf.nn.sigmoid(a)
cross_entropy=tf.reduce_mean(tf.nn.sigmoid_cross_entropy_with_logits(logits=a, labels=y))
# 等价写法:
# y_hat=tf.nn.sigmoid(tf.matmul(x_col_2D,A)+b)
# cross_entropy = tf.reduce_mean(-tf.reduce_sum(y * tf.log(y_hat), axis=1))
train_step = tf.train.GradientDescentOptimizer(learning_rate=0.001).minimize(cross_entropy)
step5:训练阶段
sess.run(tf.global_variables_initializer())
for i,j in enumerate(vocab_processor.transform(X)):
sess.run(train_step,feed_dict={x:j,y:[[Y[i]]]})
# 预测阶段
Y_predict=[]
for i,j in enumerate(vocab_processor.transform(X)):
if i % 100 == 0: print(i)
Y_predict.append(sess.run(y_hat,feed_dict={x:j})[0][0])
# 评价阶段
from sklearn.metrics import roc_auc_score
roc_auc_score(Y,Y_predict)
注:上面的案例改编自Nick McClure:《TensorFlow机器学习实战指南》
有如下问题:
- 每次训练只送入一个样本,对于随机梯度下降比较糟糕,并且工程上迭代也慢。
- 样本偏斜严重
- 4000多个样本,2000多个维度
- tensorflow.contrib.learn 将会DEPRECATED
改进
下面把处理OneHot后存储为csv,然后批量读取csv去训练模型(很占内存,慎重!)
step1:处理OneHot,并存储为csv
import pandas as pd
df=pd.read_csv('SMSSpamCollection.csv',sep='\t',header=None,names=['label','sentences'])
Y=(df.label=='spam')*1
import re
regex=re.compile('[a-zA-Z]+')
X1=[regex.findall(sentence) for sentence in df.sentences] # 只挑出单词,去除标点符号和数字
X2=[[i.lower() for i in j if len(i)>1] for j in X1] # 改成小写,并剔除单个字母
X=[' '.join(i) for i in X2] # 重新整合成句子
from tensorflow.contrib import learn
vocab_processor=learn.preprocessing.VocabularyProcessor(max_document_length=25,min_frequency=3) # max_document_length 是每个句子最大的长度,短的补空值,长的截取
vocab_processor.fit(X)
embedding_size=len(vocab_processor.vocabulary_) # 词典向量的长度
import tensorflow as tf
sess = tf.Session()
identity_mat = tf.diag(tf.ones(shape=[embedding_size]))
A = tf.Variable(tf.random_normal(shape=(embedding_size, 1)))
b = tf.Variable(tf.random_normal(shape=[1, 1]))
x = tf.placeholder(shape=[25], dtype=tf.int32) # 序号组成的向量,将来用vocab_processor.transform()来feed
y = tf.placeholder(shape=[1, 1], dtype=tf.float32)
x_embed = tf.nn.embedding_lookup(identity_mat, x)
x_col_sum=tf.reduce_sum(x_embed,axis=0) # 上一步是每个单词的OneHot编码,现在加总成句子的OneHot编码
x_col_sum_2D=tf.expand_dims(x_col_sum,axis=0)
f_X=open('X_embed.csv','w')
for i in vocab_processor.transform(X):
x_col_sums_2D_values=sess.run(x_col_sum_2D,feed_dict={x:i})[0]
f_X.writelines(','.join([str(j) for j in x_col_sums_2D_values])+'\n')
f_X.close()
f_Y=open('Y.csv','w')
f_Y.writelines(','.join([str(i) for i in Y]))
f_Y.close()
下面是读数据和普通的逻辑回归
import pandas as pd
X_embed=pd.read_csv('X_embed.csv',header=None).values
Y=pd.read_csv('Y.csv',header=None).values[0].reshape(-1,1)
num_sample,num_features=X_embed.shape
# 定义变量
import tensorflow as tf
x_embed=tf.placeholder(shape=(None,num_features),dtype=tf.float32)
y=tf.placeholder(shape=(None,1),dtype=tf.float32)
A=tf.Variable(tf.truncated_normal(shape=(num_features,1),dtype=tf.float32))
b=tf.Variable(tf.truncated_normal(shape=(1,1),dtype=tf.float32))
a=tf.matmul(x_embed,A)+b
y_hat=tf.nn.sigmoid(a)
cross_entropy=tf.reduce_mean(tf.nn.sigmoid_cross_entropy_with_logits(logits=a, labels=y))
train_step=tf.train.GradientDescentOptimizer(learning_rate=0.001).minimize(cross_entropy)
sess=tf.Session()
sess.run(tf.global_variables_initializer())
a_list=[]
import numpy as np
batch_size=30
for i in range(200):
choice_index=np.random.choice(num_sample,size=batch_size)
sess.run(train_step,feed_dict={x_embed:X_embed[choice_index],y:Y[choice_index]})
参考资料
Nick McClure:《TensorFlow机器学习实战指南》 机械工业出版社
lan Goodfellow:《深度学习》 人民邮电出版社
王琛等:《深度学习原理与TensorFlow实战》 电子工业出版社
李嘉璇:《TensorFlow技术解析与实战》 人民邮电出版社
黄文坚:《TensorFlow实战》 电子工业出版社
郑泽宇等:《TensorFlow实战Google深度学习框架》 电子工业出版社
您的支持将鼓励我继续创作!
Recommend
About Joyk
Aggregate valuable and interesting links.
Joyk means Joy of geeK