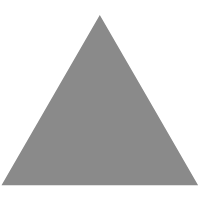
6
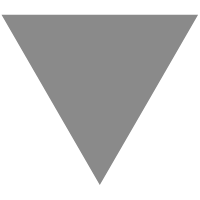
【丢弃】【NLP2】word2vec
source link: https://www.guofei.site/2018/09/21/nlp2.html
Go to the source link to view the article. You can view the picture content, updated content and better typesetting reading experience. If the link is broken, please click the button below to view the snapshot at that time.
版权声明:本文作者是郭飞。转载随意,但需要标明原文链接,并通知本人
原文链接:https://www.guofei.site/2018/09/21/nlp2.html
回收原因,这是之前仿照网络写的 word2vec,后来自己重写了一遍,新的更好读
word2vec
import tensorflow as tf
import numpy as np
import string
import collections
from tensorflow.python.framework import ops
# %%
ops.reset_default_graph()
# os.chdir(os.path.dirname(os.path.realpath(__file__)))
# Start a graph session
sess = tf.Session()
# Declare model parameters
batch_size = 100
embedding_size = 200
vocabulary_size = 10000
generations = 100000
print_loss_every = 2000
num_sampled = int(batch_size / 2) # Number of negative examples to sample.
window_size = 2 # How many words to consider left and right.
# Declare stop words
# import nltk
# nltk.download() # 需要先执行这个来下载数据,否则报错
# nltk.download('stopwords')
from nltk.corpus import stopwords
stops = stopwords.words('english')
# We pick five test words. We are expecting synonyms to appear
print_valid_every = 5000
valid_words = ['cliche', 'love', 'hate', 'silly', 'sad']
# %%
import pandas as pd
url_neg = 'http://www.guofei.site/datasets_for_ml/rt-polaritydata/rt-polaritydata/rt-polarity.neg'
url_pos = 'http://www.guofei.site/datasets_for_ml/rt-polaritydata/rt-polaritydata/rt-polarity.pos'
df_neg = pd.read_csv(url_neg, sep='\t', header=None, names=['text'])
df_pos = pd.read_csv(url_pos, sep='\t', header=None, names=['text'])
texts = list(df_pos.text) + list(df_neg.text)
target = [1] * df_pos.shape[0] + [0] * df_neg.shape[0]
# %%
# Normalize text
def normalize_text(texts, stops):
# Lower case
texts = [x.lower() for x in texts]
# Remove punctuation
texts = [''.join(c for c in x if c not in string.punctuation) for x in texts]
# Remove numbers
texts = [''.join(c for c in x if c not in '0123456789') for x in texts]
# Remove stopwords
texts = [' '.join([word for word in x.split() if word not in stops]) for x in texts]
# Trim extra whitespace
texts = [' '.join(x.split()) for x in texts]
return texts
texts = normalize_text(texts, stops)
# 影评要3个字以上才是有效的分析对象
# Texts must contain at least 3 words
target = [target[ix] for ix, x in enumerate(texts) if len(x.split()) > 2]
texts = [x for x in texts if len(x.split()) > 2]
# !!! 这里有个大问题:单个字母的单词也保留了
# %%
# 6. 创建词汇表
# Build dictionary of words
def build_dictionary(sentences, vocabulary_size):
# Turn sentences (list of strings) into lists of words
split_sentences = [s.split() for s in sentences]
words = [x for sublist in split_sentences for x in sublist]
# Initialize list of [word, word_count] for each word, starting with unknown
count = [['RARE', -1]]
# Now add most frequent words, limited to the N-most frequent (N=vocabulary size)
count.extend(collections.Counter(words).most_common(vocabulary_size - 1))
# Now create the dictionary
word_dict = {}
# For each word, that we want in the dictionary, add it, then make it
# the value of the prior dictionary length
for word, word_count in count:
word_dict[word] = len(word_dict)
return word_dict
# Build our data set and dictionaries
word_dictionary = build_dictionary(texts, vocabulary_size)
# %%
# Turn text data into lists of integers from dictionary
def text_to_numbers(sentences, word_dict):
# Initialize the returned data
data = []
for sentence in sentences:
sentence_data = []
# For each word, either use selected index or rare word index
for word in sentence.split(' '):
if word in word_dict:
word_ix = word_dict[word]
else:
word_ix = 0
sentence_data.append(word_ix)
data.append(sentence_data)
return data
word_dictionary_rev = dict(zip(word_dictionary.values(), word_dictionary.keys()))
text_data = text_to_numbers(texts, word_dictionary)
# !text_data是 序号列表,序号是在vocabulary中的index
# %%
# Get validation word keys
# 待评估的单词在vocabulary中的index
valid_examples = [word_dictionary[x] for x in valid_words]
# %%
# 返回 skip-gram 模型数据
# Generate data randomly (N words behind, target, N words ahead)
def generate_batch_data(sentences, batch_size, window_size, method='skip_gram'):
# Fill up data batch
batch_data = []
label_data = []
while len(batch_data) < batch_size:
# select random sentence to start
rand_sentence = np.random.choice(sentences)
# Generate consecutive windows to look at
window_sequences = [rand_sentence[max((ix - window_size), 0):(ix + window_size + 1)] for ix, x in
enumerate(rand_sentence)]
# Denote which element of each window is the center word of interest
label_indices = [ix if ix < window_size else window_size for ix, x in enumerate(window_sequences)]
# Pull out center word of interest for each window and create a tuple for each window
if method == 'skip_gram':
batch_and_labels = [(x[y], x[:y] + x[(y + 1):]) for x, y in zip(window_sequences, label_indices)]
# Make it in to a big list of tuples (target word, surrounding word)
tuple_data = [(x, y_) for x, y in batch_and_labels for y_ in y]
elif method == 'cbow':
batch_and_labels = [(x[:y] + x[(y + 1):], x[y]) for x, y in zip(window_sequences, label_indices)]
# Make it in to a big list of tuples (target word, surrounding word)
tuple_data = [(x_, y) for x, y in batch_and_labels for x_ in x]
else:
raise ValueError('Method {} not implemented yet.'.format(method))
# extract batch and labels
batch, labels = [list(x) for x in zip(*tuple_data)]
batch_data.extend(batch[:batch_size])
label_data.extend(labels[:batch_size])
# Trim batch and label at the end
batch_data = batch_data[:batch_size]
label_data = label_data[:batch_size]
# Convert to numpy array
batch_data = np.array(batch_data)
label_data = np.transpose(np.array([label_data]))
return batch_data, label_data
# 示例:
# batch_inputs, batch_labels = generate_batch_data(text_data, batch_size, window_size)
# 将来的预测输入:feed_dict = {x_inputs: batch_inputs, y_target: batch_labels}
# %%
# Define Embeddings:
embeddings = tf.Variable(tf.random_uniform([vocabulary_size, embedding_size], -1.0, 1.0))
# NCE loss parameters
nce_weights = tf.Variable(tf.truncated_normal([vocabulary_size, embedding_size], stddev=1.0 / np.sqrt(embedding_size)))
nce_biases = tf.Variable(tf.zeros([vocabulary_size]))
# Create data/target placeholders
x_inputs = tf.placeholder(tf.int32, shape=[batch_size])
y_target = tf.placeholder(tf.int32, shape=[batch_size, 1])
valid_dataset = tf.constant(valid_examples, dtype=tf.int32)
# Lookup the word embedding:
embed = tf.nn.embedding_lookup(embeddings, x_inputs)
# %%
# Define Embeddings:
embeddings = tf.Variable(tf.random_uniform([vocabulary_size, embedding_size], -1.0, 1.0))
# NCE loss parameters
nce_weights = tf.Variable(tf.truncated_normal([vocabulary_size, embedding_size], stddev=1.0 / np.sqrt(embedding_size)))
nce_biases = tf.Variable(tf.zeros([vocabulary_size]))
# Create data/target placeholders
x_inputs = tf.placeholder(tf.int32, shape=[batch_size])
y_target = tf.placeholder(tf.int32, shape=[batch_size, 1])
valid_dataset = tf.constant(valid_examples, dtype=tf.int32)
# Lookup the word embedding:
embed = tf.nn.embedding_lookup(embeddings, x_inputs)
# Get loss from prediction
loss = tf.reduce_mean(
tf.nn.nce_loss(weights=nce_weights, biases=nce_biases, labels=y_target, inputs=embed, num_sampled=num_sampled,
num_classes=vocabulary_size))
# Create optimizer
optimizer = tf.train.GradientDescentOptimizer(learning_rate=1.0).minimize(loss)
# Cosine similarity between words
norm = tf.sqrt(tf.reduce_sum(tf.square(embeddings), 1, keepdims=True))
normalized_embeddings = embeddings / norm
valid_embeddings = tf.nn.embedding_lookup(normalized_embeddings, valid_dataset)
similarity = tf.matmul(valid_embeddings, normalized_embeddings, transpose_b=True)
# Add variable initializer.
init = tf.global_variables_initializer()
sess.run(init)
# Run the skip gram model.
loss_vec = []
loss_x_vec = []
for i in range(generations):
batch_inputs, batch_labels = generate_batch_data(text_data, batch_size, window_size)
feed_dict = {x_inputs: batch_inputs, y_target: batch_labels}
# Run the train step
sess.run(optimizer, feed_dict=feed_dict)
# Return the loss
if (i + 1) % print_loss_every == 0:
loss_val = sess.run(loss, feed_dict=feed_dict)
loss_vec.append(loss_val)
loss_x_vec.append(i + 1)
print('Loss at step {} : {}'.format(i + 1, loss_val))
# Validation: Print some random words and top 5 related words
if (i + 1) % print_valid_every == 0:
sim = sess.run(similarity, feed_dict=feed_dict)
for j in range(len(valid_words)):
valid_word = valid_words[j]
# top_k = number of nearest neighbors
top_k = 5
nearest = (-sim[j, :]).argsort()[1:top_k + 1]
log_str = "Nearest to {}:".format(valid_word)
for k in range(top_k):
close_word = word_dictionary_rev[nearest[k]]
log_str = '{} {},'.format(log_str, close_word)
print(log_str)
参考资料
https://blog.csdn.net/pipisorry/article/details/41957763
Nick McClure:《TensorFlow机器学习实战指南》 机械工业出版社
lan Goodfellow:《深度学习》 人民邮电出版社
王琛等:《深度学习原理与TensorFlow实战》 电子工业出版社
李嘉璇:《TensorFlow技术解析与实战》 人民邮电出版社
黄文坚:《TensorFlow实战》 电子工业出版社
郑泽宇等:《TensorFlow实战Google深度学习框架》 电子工业出版社
您的支持将鼓励我继续创作!
Recommend
About Joyk
Aggregate valuable and interesting links.
Joyk means Joy of geeK