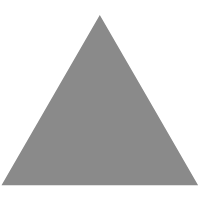
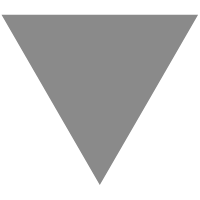
Google Cloud Industries VP shares the dos and don’ts of generative AI projects
source link: https://diginomica.com/google-cloud-industries-vp-shares-dos-and-donts-generative-ai-projects
Go to the source link to view the article. You can view the picture content, updated content and better typesetting reading experience. If the link is broken, please click the button below to view the snapshot at that time.
Google Cloud Industries VP shares the dos and don’ts of generative AI projects

At Google Cloud’s Next event in Las Vegas last week we heard from the vendor’s CEO, Thomas Kurian, about why he is prioritizing an ‘open’ AI platform, one that allows buyers to build AI applications that mean new and differentiated experiences. One of the key elements of Kurian’s pitch was the idea of ‘AI agents’, which are built through Google Cloud’s Vertex platform, and serve a number of roles, including customer agents, creative agents, employee agents, and data agents. We also heard from customers that had started deploying their own generative AI projects in production, which are already delivering efficiency gains.
However, it’s still very early days for generative AI and many customers will be looking on with apprehension as they assess their incomplete cloud and data modernization projects of the past few years. AI may be here today, but many buyers are still trying to figure out yesterday’s problem.
With this in mind, I sat down with Carrie Tharp, Google Cloud’s VP of Strategic Industries, to discuss what she is seeing on the ground with customers - to understand where they are experimenting, what their priorities are, where success is being realized, and what common mistakes are being made. Tharp works closely with buyers and has a keen eye for the common themes occurring across industries and generative AI projects, which may prove helpful information to those assessing their options.
Tharp said that across all industries, companies were somewhere between ‘25% to 40% along the AI journey’ - when looking at what we used to think about as ‘AI’ (read: automation), before generative AI came along and changed everything. These companies, according to Tharp, will have an advantage with future generative AI projects. She said:
Companies that have spent time building those data foundations, and holistically have different data sources enacted, are in the best position to move into the advanced use cases.
However, this doesn’t mean that organizations that are still early on in their data modernization efforts can’t take advantage of some early generative AI developments that are being built into Google Cloud platforms - such as the work that it’s been doing across Workspace. Tharp said:
But there's something for everybody. There's going to be low hanging fruit, productivity and efficiency type use cases, that whether or not you've built your data foundation, you can start doing proofs of concepts and pilots very quickly.
Workspace is an example where you can start to get your organization's hands dirty, playing with things, before you unleash it on actual corporate processes.
Test and learn
Tharp said that the path to adoption of generative AI will depend on a number of factors, including a company’s background, what industry it is in, what data it holds, what its profit levels are, and what ROI it is seeking. However, she added that the priority should be for organizations to get going and start testing and learning:
That’s more important than ever right now. There’s no downside to trying it, failing fast, and then moving on to the next use case, or the next technology.
One common approach across most companies and industries, however, is that organizations are starting to test out generative AI applications on internal users first - before promoting anything extenerally to customers. In the case of Discover Financial Services, a buyer we spoke to at Next, this was certainly the case and something it said was a priority. Tharp said:
I see a lot of folks starting internally first. You know, there's less risk doing something with employees, than it is to unleash it on a consumer, or a patient, etc. We see folks starting with things like knowledge agents; helping your call center reps have better access to all of your company data, your policy data, product information, so they can serve customers faster.
Or legal and HR teams having access to bots that are helping the person with all the different information that you could go look for, but it might take a long time.
Also, anything that's process oriented, where there's a lot of paperwork involved, or what I call ‘pre-work’, where the human has to sit and read a bunch of things. Where they have to discern, use their experience to understand something…now they use generative AI to short cycle this.
That could be a legal document review, where the AI summarizes something for me. Another example is marketing campaigns. For a marketing campaign you have to write a marketing brief. Well, the marketing brief is a version of everything you've ever done, plus specifics for this campaign. And so why don't you have AI draft it for you? And then you just are tweaking some elements. That lets you get to the actual AI campaign, faster.
These are certainly the types of examples diginomica is seeing in the market - take a look at WPP, another Google Cloud customer, for example. Beyond demos during keynotes, I can’t think of an actual buyer I’ve spoken to that is yet doing anything external (that’s not to say it’s not happening, but on an anecdotal level, it seems rarer).
Tharp’s experience is that it’s the largest companies that are experimenting with customer-facing generative AI experiences. She said:
The largest companies are doing consumer facing stuff, they want to break ground with new experiences. But they’re still learning all the different things that consumers are going to try and do with these experiences.
They don't want to get left behind, so you really have to start that learning journey now and be working on the different elements. If you think of retail, for example, you may start with an agent that generally helps with customer service first, and then it starts helping with conversational commerce and discovery. Getting the agent to that level, you might build that over time, but you want to get started.
However, key to starting internally is that buyers can try and ‘break the experiences’. Generative AI, as we’ve seen with the likes of ChatGPT, isn’t a traditional workflow or process that’s rigid and defined by an organization from the outset. It can be unpredictable and needs to be grounded with reliable data, as well as fine tuned to achieve excellence. As Tharp said:
Sometimes they're testing end consumer experiences on their associates first, so they understand all the different ways you could accidentally or intentionally break this experience. You can plan for that and make sure that the agent has the right response.
In retail, you're gonna see people try to game the system: violate return policy, stack promotions that shouldn't be stacked. You can see people ask questions that you shouldn't be answering, from a health and safety standpoint, in healthcare.
Understanding all those different versions of where humans can take an agent, and really making sure you're thinking through that, it's more complex than the old deterministic flows that we used to programme out.
Disrupt yourself
Tharp was keen to argue that even if organizations are grappling with legacy platforms, such as their ERP or e-commerce systems, AI can allow them to leapfrog to some better experiences. She said that you can ‘plug the AI into whatever your stack looks like today’, which is helping customers move faster to the experiences that they want to drive. This isn’t something that has been validated by diginomica in terms of many use cases, but in theory this is true - in that buyers could source, organize and store data in the cloud and then connect it to AI platforms. I wrote previously how AI does arguably provide a level of abstraction between end users and traditional backend processes, but this needs to be put into practice. Tharp said:
That’s part of the excitement and energy that we're getting from all the industries, where you don't have to be completely stuck in this world that’s going to take you five years to get all your databases modernized, you don't have to wait for that.
We see it as helping our customers move faster to experiences that they wanted to drive, that historically their legacy tech stack was inhibiting them from doing.
One point I raised with Tharp was something I’ve been putting to many vendors and buyers in the market, regarding generative AI, and how it could favor ‘legacy’ companies. So much of the past decade has been defined by digital disruptors forcing consolidation in the market and driving customers to new business models. It’s the classic Netflix vs Blockbuster example, but we’ve seen it in many areas, including finance, energy, transport, etc.
However, I have wondered whether or not those companies that have been around for decades (if they’re still active in the market) may hold some advantage with generative AI, compared to new entrants, because of the amount of data that they hold. Tharp agrees and said:
It’s something I've talked about quite a bit, this ability to disrupt yourself. These enterprises that are sitting on these very large corpuses of information, they have customer data, they have all of their historical transaction data. In healthcare they have all of your patient data.
So you're sitting on this data that is proprietary, and probably has untapped insights in it. You're in a better position at scale, with this time period of history that you have, with this latent asset that you can now use in a different way.
That’s part of the challenge or the opportunity in front of all these industries to say:‘now that I have these new tools I’m inherently advantaged to use this very large data source, how do I use that to grow revenue and reduce costs?’
I think that's one of the most exciting pieces.
Common pitfalls
Finally, Tharp was able to provide some examples of mistakes that she’s seeing organizations commonly make across all industries, when starting to adopt generative AI. Firstly, Tharp said that it’s wrong to assume that generative AI solves all unstructured data problems:
It's going to help you unlock that unstructured data unlike you could before, but it doesn't mean skipping traditional steps and preparing your data for the use cases. We see that if you prepare the proper data foundation…those are the experiences that we're seeing go live to production. Don’t think about a narrow single use case, think about it more holistically, the process end to end.
Secondly, organizations may be tempted to delegate ‘generative AI’ to the work of IT and technology teams, but Tharp argued that this would be a mistake. Not to say IT shouldn’t be involved, but that multi-disciplinary teams should be established, with leaders from different lines of business, to assess the impact of use cases. She provided an example of a customer that had adopted AI to generate images for marketing purposes, but that the technology team had been given the responsibility to create the tool and hadn’t considered the aims of the marketing department. Tharp said:
They ended up presenting an image that was kind of contrary to that brand's positioning at the time, so it was not well received by the end consumer because it was kind of night and day.
And when just the Chief Digital Officer and the IT teams were involved, they were simply looking at conversion. The more images you have, the more diversity that's shown, the better kind of conversion you're going to see. And so thinking about how to construct those teams, and using a broader type of teams [is helpful]
For example, we're seeing UX and UI resources are actually really good at prompt engineering, because they're used to thinking through: how would you interact with a person in this way and what do I need to think about in this flow?
And thirdly, whilst technology, people and process still applies as a set of principles for generative AI, organizations should also be thinking about including prioritization as part of that. Tharp said:
We also see a lot of folks coming in with spreadsheets of hundreds of use cases, super excited about all of them, and a huge range of different values they're going to see.
You don't want to get into a kind of paralysis around prioritizing for too long, not thinking through what the implications of the use cases are or the ones that have more trust implications.
My take
Lots to digest here, but for organizations just starting out, perhaps some helpful guiding thoughts for approaching generative AI.
Recommend
About Joyk
Aggregate valuable and interesting links.
Joyk means Joy of geeK