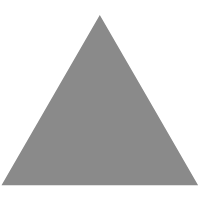
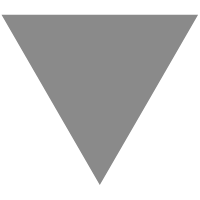
Tensorflow2 深度学习十必知 - cpuimage
source link: https://www.cnblogs.com/cpuimage/p/16427268.html
Go to the source link to view the article. You can view the picture content, updated content and better typesetting reading experience. If the link is broken, please click the button below to view the snapshot at that time.
Tensorflow2 深度学习十必知
博主根据自身多年的深度学习算法研发经验,整理分享以下十条必知。
含参考资料链接,部分附上相关代码实现。
独乐乐不如众乐乐,希望对各位看客有所帮助。
待回头有时间再展开细节说一说深度学习里的那些道道。
有什么技术需求需要有偿解决的也可以邮件或者QQ联系博主。
邮箱QQ同ID:[email protected]
当然除了这十条,肯定还有其他“必知”,
欢迎评论分享更多,这里只是暂时拟定的十条,别较真哈。
主要学习其中的思路,切记,以下思路在个别场景并不适用 。
1.数据回流
[1907.05550] Faster Neural Network Training with Data Echoing
def data_echoing(factor): return lambda image, label: tf.data.Dataset.from_tensors((image, label)).repeat(factor) |
数据集加载后,在数据增广前后重复当前批次进模型的次数,减少数据的加载耗时。
等价于让模型看n次当前的数据,或者看n个增广后的数据样本。
2.AMP 自动精度混合
在bert4keras中使用混合精度和XLA加速训练 - 科学空间|Scientific Spaces
tf.config.optimizer.set_experimental_options({ "auto_mixed_precision" : True }) |
降低显存占用,加速训练,将部分网络计算转为等价的低精度计算,以此降低计算量。
3.优化器节省显存
3.1 [1804.04235]Adafactor: Adaptive Learning Rates with Sublinear Memory Cost
mesh/optimize.py at master · tensorflow/mesh · GitHub
3.2 [1901.11150] Memory-Efficient Adaptive Optimization
google-research/sm3 at master · google-research/google-research (github.com)
节省显存,加速训练,
主要是对二阶动量进行特例化解构,减少显存存储。
4.权重标准化(归一化)
[2102.06171] High-Performance Large-Scale Image Recognition Without Normalization
deepmind-research/nfnets at master · deepmind/deepmind-research · GitHub
class WSConv2D(tf.keras.layers.Conv2D): def __init__( self , * args, * * kwargs): super (WSConv2D, self ).__init__( kernel_initializer = tf.keras.initializers.VarianceScaling( scale = 1.0 , mode = 'fan_in' , distribution = 'untruncated_normal' , ), use_bias = False , kernel_regularizer = tf.keras.regularizers.l2( 1e - 4 ), * args, * * kwargs ) self .gain = self .add_weight( name = 'gain' , shape = ( self .filters,), initializer = "ones" , trainable = True , dtype = self .dtype ) def standardize_weight( self , eps): mean, var = tf.nn.moments( self .kernel, axes = [ 0 , 1 , 2 ], keepdims = True ) fan_in = np.prod( self .kernel.shape[: - 1 ]) # Manually fused normalization, eq. to (w - mean) * gain / sqrt(N * var) scale = tf.math.rsqrt( tf.math.maximum( var * fan_in, tf.convert_to_tensor(eps, dtype = self .dtype) ) ) * self .gain shift = mean * scale return self .kernel * scale - shift def call( self , inputs): eps = 1e - 4 weight = self .standardize_weight(eps) return tf.nn.conv2d( inputs, weight, strides = self .strides, padding = self .padding.upper(), dilations = self .dilation_rate ) if self .bias is None else tf.nn.bias_add( tf.nn.conv2d( inputs, weight, strides = self .strides, padding = self .padding.upper(), dilations = self .dilation_rate ), self .bias) |
通过对kernel进行标准化或归一化,相当于对kernel做一个先验约束,以此加速模型训练收敛。
5.自适应梯度裁剪
deepmind-research/agc_optax.py at master · deepmind/deepmind-research · GitHub
def unitwise_norm(x): if len (tf.squeeze(x).shape) < = 1 : # Scalars and vectors axis = None keepdims = False elif len (x.shape) in [ 2 , 3 ]: # Linear layers of shape IO axis = 0 keepdims = True elif len (x.shape) = = 4 : # Conv kernels of shape HWIO axis = [ 0 , 1 , 2 , ] keepdims = True else : raise ValueError(f 'Got a parameter with shape not in [1, 2, 3, 4]! {x}' ) square_sum = tf.reduce_sum(tf.square(x), axis, keepdims = keepdims) return tf.sqrt(square_sum) def gradient_clipping(grad, var): clipping = 0.01 max_norm = tf.maximum(unitwise_norm(var), 1e - 3 ) * clipping grad_norm = unitwise_norm(grad) trigger = (grad_norm > max_norm) clipped_grad = (max_norm / tf.maximum(grad_norm, 1e - 6 )) return grad * tf.where(trigger, clipped_grad, tf.ones_like(clipped_grad)) |
防止梯度爆炸,稳定训练。通过梯度和参数的关系,对梯度进行裁剪,约束学习率。
6.recompute_grad
[1604.06174] Training Deep Nets with Sublinear Memory Cost
google-research/recompute_grad.py at master · google-research/google-research (github.com)
bojone/keras_recompute: saving memory by recomputing for keras (github.com)
通过梯度重计算,节省显存。
7.归一化
[2003.05569] Extended Batch Normalization (arxiv.org)
from keras.layers.normalization.batch_normalization import BatchNormalizationBase class ExtendedBatchNormalization(BatchNormalizationBase): def __init__( self , axis = - 1 , momentum = 0.99 , epsilon = 1e - 3 , center = True , scale = True , beta_initializer = 'zeros' , gamma_initializer = 'ones' , moving_mean_initializer = 'zeros' , moving_variance_initializer = 'ones' , beta_regularizer = None , gamma_regularizer = None , beta_constraint = None , gamma_constraint = None , renorm = False , renorm_clipping = None , renorm_momentum = 0.99 , trainable = True , name = None , * * kwargs): # Currently we only support aggregating over the global batch size. super (ExtendedBatchNormalization, self ).__init__( axis = axis, momentum = momentum, epsilon = epsilon, center = center, scale = scale, beta_initializer = beta_initializer, gamma_initializer = gamma_initializer, moving_mean_initializer = moving_mean_initializer, moving_variance_initializer = moving_variance_initializer, beta_regularizer = beta_regularizer, gamma_regularizer = gamma_regularizer, beta_constraint = beta_constraint, gamma_constraint = gamma_constraint, renorm = renorm, renorm_clipping = renorm_clipping, renorm_momentum = renorm_momentum, fused = False , trainable = trainable, virtual_batch_size = None , name = name, * * kwargs) def _calculate_mean_and_var( self , x, axes, keep_dims): with tf.keras.backend.name_scope( 'moments' ): y = tf.cast(x, tf.float32) if x.dtype = = tf.float16 else x replica_ctx = tf.distribute.get_replica_context() if replica_ctx: local_sum = tf.math.reduce_sum(y, axis = axes, keepdims = True ) local_squared_sum = tf.math.reduce_sum(tf.math.square(y), axis = axes, keepdims = True ) batch_size = tf.cast(tf.shape(y)[ 0 ], tf.float32) y_sum = replica_ctx.all_reduce(tf.distribute.ReduceOp. SUM , local_sum) y_squared_sum = replica_ctx.all_reduce(tf.distribute.ReduceOp. SUM , local_squared_sum) global_batch_size = replica_ctx.all_reduce(tf.distribute.ReduceOp. SUM , batch_size) axes_vals = [(tf.shape(y))[i] for i in range ( 1 , len (axes))] multiplier = tf.cast(tf.reduce_prod(axes_vals), tf.float32) multiplier = multiplier * global_batch_size mean = y_sum / multiplier y_squared_mean = y_squared_sum / multiplier # var = E(x^2) - E(x)^2 variance = y_squared_mean - tf.math.square(mean) else : # Compute true mean while keeping the dims for proper broadcasting. mean = tf.math.reduce_mean(y, axes, keepdims = True , name = 'mean' ) variance = tf.math.reduce_mean( tf.math.squared_difference(y, tf.stop_gradient(mean)), axes, keepdims = True , name = 'variance' ) if not keep_dims: mean = tf.squeeze(mean, axes) variance = tf.squeeze(variance, axes) variance = tf.math.reduce_mean(variance) if x.dtype = = tf.float16: return (tf.cast(mean, tf.float16), tf.cast(variance, tf.float16)) else : return mean, variance |
一个简易改进版的Batch Normalization,思路简单有效。
8.学习率策略
[1506.01186] Cyclical Learning Rates for Training Neural Networks (arxiv.org)
一个推荐的学习率策略方案,特定情况下可以取得更好的泛化。
9.重参数化
https://zhuanlan.zhihu.com/p/361090497
通过同时训练多份参数,合并权重的思路来提升模型泛化性。
10.长尾学习
[2110.04596] Deep Long-Tailed Learning: A Survey (arxiv.org)
Jorwnpay/A-Long-Tailed-Survey: 本项目是 Deep Long-Tailed Learning: A Survey 文章的中译版 (github.com)
解决长尾问题,可以加速收敛,提升模型泛化,稳定训练。
Recommend
About Joyk
Aggregate valuable and interesting links.
Joyk means Joy of geeK