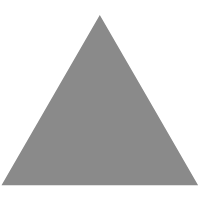
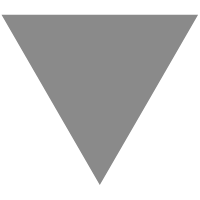
【机器学习】线性回归预测 - hjk-airl
source link: https://www.cnblogs.com/hjk-airl/p/16405474.html
Go to the source link to view the article. You can view the picture content, updated content and better typesetting reading experience. If the link is broken, please click the button below to view the snapshot at that time.
回归分析就是用于预测输入变量(自变量)和输出变量(因变量)之间的关系,特别当输入的值发生变化时,输出变量值也发生改变!回归简单来说就是对数据进行拟合。线性回归就是通过线性的函数对数据进行拟合。机器学习并不能实现预言,只能实现简单的预测。我们这次对房价关于其他因素的关系。
波士顿房价预测
下载相关数据集
- 数据集是506行14列的波士顿房价数据集,数据集是开源的。
wget.download(url='https://archive.ics.uci.edu/ml/machine-learning-databases/housing/housing.data',out= 'housing.data')
wget.download(url='https://archive.ics.uci.edu/ml/machine-learning-databases/housing/housing.names',out='housing.names')
wget.download(url='https://archive.ics.uci.edu/ml/machine-learning-databases/housing/Index',out='Index')
对数据集进行处理
feature_names = ['CRIM','ZN','INDUS','CHAS','NOX','RM','AGE','DIS','RAD','TAX','PTRATIO','B','LSTAT','MEDV']
feature_num = len(feature_names)
print(feature_num)
# 把7084 变为506*14
housing_data = housing_data.reshape(housing_data.shape[0]//feature_num,feature_num)
print(housing_data.shape[0])
# 打印第一行数据
print(housing_data[:1])
## 归一化
feature_max = housing_data.max(axis=0)
feature_min = housing_data.min(axis=0)
feature_avg = housing_data.sum(axis=0)/housing_data.shape[0]
## 实例化模型
def Model():
model = linear_model.LinearRegression()
return model
# 拟合模型
def train(model,x,y):
model.fit(x,y)
可视化模型效果
def draw_infer_result(groud_truths,infer_results):
title = 'Boston'
plt.title(title,fontsize=24)
x = np.arange(1,40)
y = x
plt.plot(x,y)
plt.xlabel('groud_truth')
plt.ylabel('infer_results')
plt.scatter(groud_truths,infer_results,edgecolors='green',label='training cost')
plt.grid()
plt.show()
## 基于线性回归实现房价预测
## 拟合函数模型
## 梯度下降方法
## 开源房价策略数据集
import wget
import numpy as np
import os
import matplotlib
import matplotlib.pyplot as plt
import pandas as pd
from sklearn import linear_model
## 下载之后注释掉
'''
wget.download(url='https://archive.ics.uci.edu/ml/machine-learning-databases/housing/housing.data',out= 'housing.data')
wget.download(url='https://archive.ics.uci.edu/ml/machine-learning-databases/housing/housing.names',out='housing.names')
wget.download(url='https://archive.ics.uci.edu/ml/machine-learning-databases/housing/Index',out='Index')
'''
'''
1. CRIM per capita crime rate by town
2. ZN proportion of residential land zoned for lots over
25,000 sq.ft.
3. INDUS proportion of non-retail business acres per town
4. CHAS Charles River dummy variable (= 1 if tract bounds
river; 0 otherwise)
5. NOX nitric oxides concentration (parts per 10 million)
6. RM average number of rooms per dwelling
7. AGE proportion of owner-occupied units built prior to 1940
8. DIS weighted distances to five Boston employment centres
9. RAD index of accessibility to radial highways
10. TAX full-value property-tax rate per $10,000
11. PTRATIO pupil-teacher ratio by town
12. B 1000(Bk - 0.63)^2 where Bk is the proportion of blacks
by town
13. LSTAT % lower status of the population
14. MEDV Median value of owner-occupied homes in $1000's
'''
## 数据加载
datafile = './housing.data'
housing_data = np.fromfile(datafile,sep=' ')
print(housing_data.shape)
feature_names = ['CRIM','ZN','INDUS','CHAS','NOX','RM','AGE','DIS','RAD','TAX','PTRATIO','B','LSTAT','MEDV']
feature_num = len(feature_names)
print(feature_num)
# 把7084 变为506*14
housing_data = housing_data.reshape(housing_data.shape[0]//feature_num,feature_num)
print(housing_data.shape[0])
# 打印第一行数据
print(housing_data[:1])
## 归一化
feature_max = housing_data.max(axis=0)
feature_min = housing_data.min(axis=0)
feature_avg = housing_data.sum(axis=0)/housing_data.shape[0]
def feature_norm(input):
f_size = input.shape
output_features = np.zeros(f_size,np.float32)
for batch_id in range(f_size[0]):
for index in range(13):
output_features[batch_id][index] = (input[batch_id][index]-feature_avg[index])/(feature_max[index]-feature_min[index])
return output_features
housing_features = feature_norm(housing_data[:,:13])
housing_data = np.c_[housing_features,housing_data[:,-1]].astype(np.float32)
## 划分数据集 8:2
ratio =0.8
offset = int(housing_data.shape[0]*ratio)
train_data = housing_data[:offset]
test_data = housing_data[offset:]
print(train_data[:2])
## 模型配置
## 线性回归
## 实例化模型
def Model():
model = linear_model.LinearRegression()
return model
# 拟合模型
def train(model,x,y):
model.fit(x,y)
## 模型训练
X, y = train_data[:,:13], train_data[:,-1:]
model = Model()
train(model,X,y)
x_test, y_test = test_data[:,:13], test_data[:,-1:]
prefict = model.predict(x_test)
## 模型评估
infer_results = []
groud_truths = []
def draw_infer_result(groud_truths,infer_results):
title = 'Boston'
plt.title(title,fontsize=24)
x = np.arange(1,40)
y = x
plt.plot(x,y)
plt.xlabel('groud_truth')
plt.ylabel('infer_results')
plt.scatter(groud_truths,infer_results,edgecolors='green',label='training cost')
plt.grid()
plt.show()
draw_infer_result(y_test,prefict)
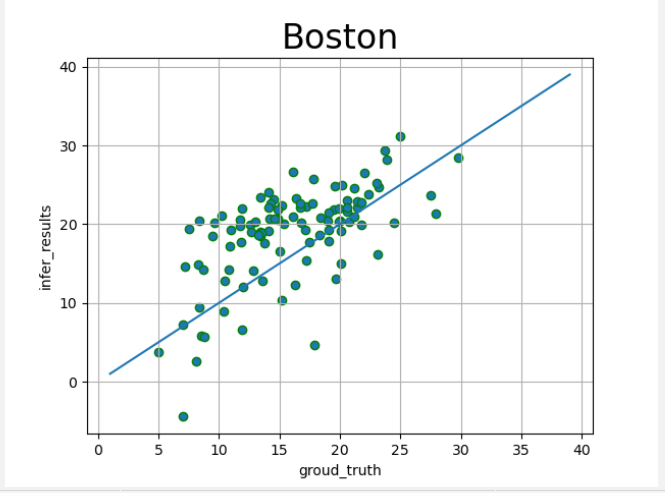
线性回归预测还是比较简单的,可以简单理解为函数拟合,数据集是使用的开源的波士顿房价的数据集,算法也是打包好的包,方便我们引用。
Recommend
About Joyk
Aggregate valuable and interesting links.
Joyk means Joy of geeK