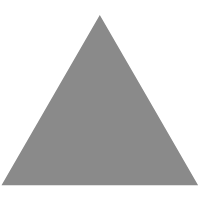
0
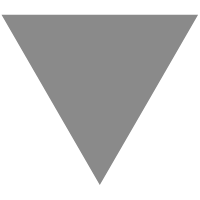
【TensorFlow案例2】用tf实现svm
source link: https://www.guofei.site/2018/11/09/tfcase2.html
Go to the source link to view the article. You can view the picture content, updated content and better typesetting reading experience. If the link is broken, please click the button below to view the snapshot at that time.
【TensorFlow案例2】用tf实现svm
2018年11月09日Author: Guofei
文章归类: 2-3-神经网络与TF ,文章编号: 292
版权声明:本文作者是郭飞。转载随意,但需要标明原文链接,并通知本人
原文链接:https://www.guofei.site/2018/11/09/tfcase2.html
线性svm用于分类
理论见于这里
这里不用对偶性,而是针对原问题做优化:
minw,b∑i=1N[1−yi(wxi+b)]++λ∣∣w∣∣2minw,b∑i=1N[1−yi(wxi+b)]++λ∣∣w∣∣2
step1:导入数据和包
import matplotlib.pyplot as plt
import numpy as np
import tensorflow as tf
from sklearn import datasets
import sklearn.model_selection as model_selection
iris = datasets.load_iris()
X = np.array([[x[0], x[3]] for x in iris.data])
Y = np.array([1 if y == 0 else -1 for y in iris.target])
X_train, X_test, Y_train, Y_test = model_selection.train_test_split(X, Y, test_size=0.1)
step2:构建网络
x_data = tf.placeholder(shape=[None, 2], dtype=tf.float32)
y_target = tf.placeholder(shape=[None, 1], dtype=tf.float32)
A = tf.Variable(tf.random_normal(shape=[2, 1]))
b = tf.Variable(tf.random_normal(shape=[1, 1]))
model_output = tf.matmul(x_data, A) + b
# Declare vector L2 'norm' function squared
l2_norm = tf.reduce_sum(tf.square(A))
# Declare loss function
# Loss = max(0, 1-pred*actual) + alpha * L2_norm(A)^2
alpha = tf.constant([0.1])
classification_term = tf.reduce_sum(tf.maximum(0., 1. - model_output * y_target))
loss = classification_term + tf.multiply(alpha, l2_norm)
# Declare prediction function
prediction = tf.sign(model_output)
accuracy = tf.reduce_mean(tf.cast(tf.equal(prediction, y_target), tf.float32))
# Declare optimizer
my_opt = tf.train.GradientDescentOptimizer(0.001)
train_step = my_opt.minimize(loss)
step3:训练
batch_size = 135
sess = tf.Session()
init = tf.global_variables_initializer()
sess.run(init)
loss_vec = []
train_accuracy = []
test_accuracy = []
for i in range(500):
rand_index = np.random.choice(len(X_train), size=batch_size)
rand_x = X_train[rand_index]
rand_y = np.transpose([Y_train[rand_index]])
sess.run(train_step, feed_dict={x_data: rand_x, y_target: rand_y})
temp_loss = sess.run(loss, feed_dict={x_data: rand_x, y_target: rand_y})
loss_vec.append(temp_loss)
train_acc_temp = sess.run(accuracy, feed_dict={
x_data: X_train,
y_target: np.transpose([Y_train])})
train_accuracy.append(train_acc_temp)
test_acc_temp = sess.run(accuracy, feed_dict={
x_data: X_test,
y_target: np.transpose([Y_test])})
test_accuracy.append(test_acc_temp)
if (i + 1) % 100 == 0:
print('Step #{} A = {}, b = {}'.format(
str(i + 1),
str(sess.run(A)),
str(sess.run(b))
))
print('Loss = ' + str(temp_loss))
step4:画图
# %%
setosa = X[Y == 1]
not_setosa = X[Y == -1]
plt.plot(setosa[:, 0], setosa[:, 1], 'o', label='setosa')
plt.plot(not_setosa[:, 0], not_setosa[:, 1], 'x', label='not-setosa')
[[a1], [a2]] = sess.run(A)
[[b]] = sess.run(b)
plt.plot(X[:, 0], (-b - a1 * X[:, 0]) / a2)
plt.show()
# %%
# Plot train/test accuracies
plt.plot(train_accuracy, 'k-', label='Training Accuracy')
plt.plot(test_accuracy, 'r--', label='Test Accuracy')
plt.title('Train and Test Set Accuracies')
plt.xlabel('Generation')
plt.ylabel('Accuracy')
plt.legend(loc='lower right')
plt.show()
# Plot loss over time
plt.plot(loss_vec, 'k-')
plt.title('Loss per Generation')
plt.xlabel('Generation')
plt.ylabel('Loss')
plt.show()
线性svm用于回归
损失函数是max(0,∣yi−(Axi+b)∣−ε)max(0,∣yi−(Axi+b)∣−ε)
step1:导入数据和包
import matplotlib.pyplot as plt
import numpy as np
import tensorflow as tf
from sklearn import datasets
import sklearn.model_selection as model_selection
iris = datasets.load_iris()
X, Y = iris.data[:, 3], iris.data[:, 0]
X_train, X_test, Y_train, Y_test = model_selection.train_test_split(X, Y, test_size=0.2)
step2:构建模型
x_data = tf.placeholder(shape=[None, 1], dtype=tf.float32)
y_target = tf.placeholder(shape=[None, 1], dtype=tf.float32)
A = tf.Variable(tf.random_normal(shape=[1, 1]))
b = tf.Variable(tf.random_normal(shape=[1, 1]))
model_output = tf.matmul(x_data, A) + b
epsilon = tf.constant([0.5])
loss = tf.reduce_mean(tf.maximum(0., tf.abs(model_output - y_target) - epsilon))
my_opt = tf.train.GradientDescentOptimizer(0.075)
train_step = my_opt.minimize(loss)
step3:训练模型
sess = tf.Session()
sess.run(tf.global_variables_initializer())
# Training loop
batch_size = 50
train_loss = []
test_loss = []
for i in range(200):
rand_index = np.random.choice(len(X_train), size=batch_size)
rand_x = np.transpose([X_train[rand_index]])
rand_y = np.transpose([Y_train[rand_index]])
sess.run(train_step, feed_dict={x_data: rand_x, y_target: rand_y})
temp_train_loss = sess.run(loss,
feed_dict={x_data: np.transpose([X_train]), y_target: np.transpose([Y_train])})
train_loss.append(temp_train_loss)
temp_test_loss = sess.run(loss,
feed_dict={x_data: np.transpose([X_test]), y_target: np.transpose([Y_test])})
test_loss.append(temp_test_loss)
if (i + 1) % 50 == 0:
print('-----------')
print('Generation: ' + str(i + 1))
print('A = ' + str(sess.run(A)) + ' b = ' + str(sess.run(b)))
print('Train Loss = ' + str(temp_train_loss))
print('Test Loss = ' + str(temp_test_loss))
step4 作图
[[slope]] = sess.run(A)
[[y_intercept]] = sess.run(b)
width = sess.run(epsilon)
best_fit = slope * X + y_intercept
best_fit_upper = slope * X + y_intercept + width
best_fit_lower = slope * X + y_intercept - width
plt.plot(X, Y, 'o', label='Data Points')
plt.plot(X, best_fit, 'r-', label='SVM Regression Line', linewidth=3)
plt.plot(X, best_fit_upper, 'r--', linewidth=2)
plt.plot(X, best_fit_lower, 'r--', linewidth=2)
plt.ylim([0, 10])
plt.legend(loc='lower right')
plt.show()
# Plot loss over time
plt.plot(train_loss, 'k-', label='Train Set Loss')
plt.plot(test_loss, 'r--', label='Test Set Loss')
plt.title('L2 Loss per Generation')
plt.xlabel('Generation')
plt.ylabel('L2 Loss')
plt.legend(loc='upper right')
plt.show()
参考资料
Nick McClure:《TensorFlow机器学习实战指南》 机械工业出版社
您的支持将鼓励我继续创作!
Recommend
About Joyk
Aggregate valuable and interesting links.
Joyk means Joy of geeK