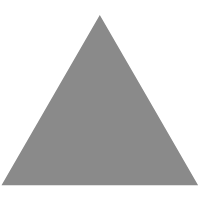
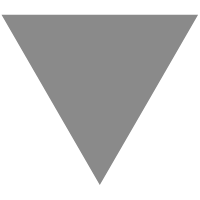
Shooting on the Street: Measuring the Spatial Influence of Physical Features on...
source link: https://link.springer.com/article/10.1007/s10940-016-9292-y
Go to the source link to view the article. You can view the picture content, updated content and better typesetting reading experience. If the link is broken, please click the button below to view the snapshot at that time.
- Original Paper
- Published: 30 March 2016
Shooting on the Street: Measuring the Spatial Influence of Physical Features on Gun Violence in a Bounded Street Network
Journal of Quantitative Criminology
volume 33, pages237–253(2017)Cite this article
-
931 Accesses
-
9 Citations
-
6 Altmetric
Abstract
Objectives
Accurately estimate the strength and extent (distance) of the spatial influence of physical features on gun violence using a street network measurement strategy.
Methods
Treating disaggregated point locations as the unit-of-analysis, the spatial influence of various physical features of place on all 2012 incidents of gun violence in Newark, NJ is estimated along a street network plane rather than a planar plane, and using a continuous operationalization of street network distances as opposed to Euclidean or Grid distances. Network-based computation methods clarify the path distances over which physical features of place, or shooting attractors, exert a significant spatial influence on gun violence. Segmented regression models estimate feature-specific distance decay patterns by demarcating the exact network distances at which the strength of attraction weakens or dissipates entirely.
Results
Findings show that liquor stores, grocery stores, bus stops, and residential foreclosures are shooting attractors in Newark, NJ. The magnitude of spatial influence is strongest in the immediate vicinity of each physical feature, and declines precipitously thereafter; yet the nature and strength of the decay varies by feature. A comparison of results analyzed on a street network plane to those based on an unbounded plane illustrates the potential biases in traditional approaches.
Conclusions
Determining whether and how strongly physical features operate as crime attractors requires constraining the analyses to the street network plane and accurately measuring continuous distances along the street network. The methodology articulated in this study can be used to more precisely estimate the spatial influence and distance decay of various physical features of place on crime density.
Introduction
The spatial array of physical features, travel routes, and behavior settings that characterize the urban landscape both influence and are constituted by the daily routines of offenders and targets. Physical features, like liquor stores, can operate as crime generators or crime attractors that draw potential victims and offenders together in time and space and elevate crime risks around them (Brantingham and Brantingham 1995; Cohen and Felson 1979; Kennedy et al. 2015). Indeed, certain types of places are routinely found to dramatically increase the risk of crime, even when accounting for local levels of socioeconomic disadvantage (Bernasco and Block 2011; Groff and Lockwood 2014; Smith et al. 2000; Stucky and Ottensmann 2009); these include bars and liquor stores (Gorman et al. 2001; Groff 2014; Lipton and Gruenewald 2002; Ratcliffe 2012; Roncek and Maier 1991; Scribner et al. 1995), public housing (Griffiths and Tita 2009; Roncek and Francik 1981), transit stops (Levine et al. 1986), and public schools (LaGrange 1999; Roncek and Faggiani 1985), among others.
While a large body of literature has firmly established the attractive effects of various physical features on crime, the precise strength and extent (distance) of their spatial influence has received much less attention. To date, scholarship has tended to focus on the degree to which criminal events cluster within specific threshold distance bands of certain types of physical features of place (Fagan and Davies 2000; Groff 2014; McNulty and Holloway 2000; Ratcliffe 2012). In these cases, distance bands encompass the entire geographic space within 400, 800, or 1500 ft, for example, of the feature under consideration. Yet a majority of the geography included in these bands may be unlikely or unable to host crime, as criminal incidents are located along the streets, paths, and other travel routes of prospective victims and would-be offenders.
A handful of recent studies published in this Journal have begun to consider street networks, rather than continuous planes, as appropriate for analyzing the geography of crime (Groff 2014; Davies and Johnson 2015). In each instance, however, it is the street segment—not the object of attraction (i.e. the specific physical feature of place)—that is treated as the unit-of-analysis. We contend that to precisely calculate the distances at which physical features exert a statistically significant attraction to crime, three conditions must be met: first, distance must be operationalized according to the travel patterns of prospective offenders along street networks rather than according to an unbounded continuous plane, as both physical features (e.g. liquor stores) and crimes (e.g. shootings) are spatial events constrained to occur in or along streets; second, precisely delineating the extent of influence over which the attraction operates requires continuous measures of distance, rather than the testing of a handful of threshold distance bands which are themselves set arbitrarily; and third, the original point locations, specifically the physical features and crimes, rather than the street segments on which they are located or proximate, should serve as the unit-of-analysis from which spatial influence is estimated. The methodology detailed below capitalizes on an approach that meets each of these conditions.
In this study, we constrain the spatial analysis plane to the specific urban street network of Newark, New Jersey, as opposed to utilizing a planar plane and calculating distance with Euclidean or grid methods, and we measure distances according to the shortest routes following street networks. In doing so, we are able to: (1) exploit network spatial measures to examine the geographic relationship between physical features and gun violence within a street network; (2) measure network distances as the shortest paths that follow streets; (3) accurately estimate the cumulative spatial influence of high risk physical features on gun violence; and (4) compute the precise spatial extent at which each physical feature operates to attract or repel gun violence, without assuming the extent of distance decay a priori. Moreover, we validate the street network approach by showing how it improves upon traditional methods—wherein Euclidean distance is typically measured on a continuous study plane—used to estimate spatial influence.
Literature Review
Spatial Influence Through Street Networks
A majority of research on crime pattern analysis has analyzed spatial data using an unbounded continuous plane, such as a whole city plane, without explicitly operationalizing the barriers (e.g. rivers) and inaccessible locations (e.g. closed highways) within the geography. Yet human activities, such as traveling, shopping, eating, and even sleeping are generally restricted to bounded street networks (Grannis 1998). Physical features of place, like grocery stores, are also not scattered randomly on the plane; instead, they are found along street networks. This means that treating largely street-network-bounded events, such as crimes, as potentially unbounded, or able to occur at any location within a whole city plane, can introduce substantial measurement error and reduce the accuracy of estimation (Okabe et al. 1995).
A handful of past studies have shown that analyses of network events in an unbounded plane produces inaccurate results. For example, Yamada and Thill (2004) compared traffic accident clusters on a network plane with those on a continuous planar surface. Not surprisingly, they found that using a continuous surface significantly raised the risk of over-detecting clustered patterns. Lu and Chen (2007) likewise concluded that crime pattern analysis on a continuous planar surface can produce both false positive and false negative results, whereas analyses on a network plane produces more reliable and accurate findings.
Measuring Distance
There are three main approaches to calculating distance to crime in the extant literature: Euclidean distance (Levine et al. 1986; Townsley and Sidebottom 2010), Manhattan distance (Levine 2005; Ratcliffe and Rengert 2008), and network distance (Lu and Chen 2007). Euclidean geometry assumes an infinitely continuous planar space in which distances are measured as straight-line paths ‘as the crow flies.’ This planar space assumption is problematic in street crime analysis because (1) the actual travel distance between two locations in a city is rarely a simple straight line, and (2) crime events are constrained by the street network (Yamada and Thill 2004). By contrast, Manhattan distance, also known as grid distance, is calculated as the sum of the horizontal (x) and vertical (y) distances between two locations on a grid (Krause 1975). Unfortunately, not all cities have a grid-style street system and, even among those that do, the use of the Manhattan distance is often inaccurate. This is because the walking distance between two points on neighboring parallel streets, for example, is actually much longer than that calculated using Manhattan geometry (Lu and Chen 2007). Therefore, the most promising method to calculate distances between network events is network distance, developed by Okabe et al. (1995). Network distance constrains analytical computations within street networks and reflects the actual shortest travel distance between two events or locations, otherwise known as the shortest path distance.
The Network Cross K Function for Stochastic Spatial Events
The Network Cross K Function is a stochastic point process modeling strategy to identify the spatial interdependence of deterministic (e.g. physical features) and stochastic points (e.g., crimes) when both sets of points are constrained to street networks (Okabe et al. 1995; Okabe and Yamada 2001). It tests the cumulative distribution function of the entire set of street crime points around the entire set of physical features of place within a range of continuous distances, and it compares the observed pattern of events with a random pattern at each distance. When the accumulated number of observed crime events at a specific distance to the physical feature is significantly greater than the accumulated number of crime events in a random distribution at the same distance, then the observed crime events are said to be attracted to the physical feature. When the accumulated number of observed crime points is significantly less than would be expected in a random situation, crime is repelled by the feature. If there is no difference between the observed and the random pattern, crime points and the locations of physical features of place can be said to be unrelated.
The Network Cross K-Function tests the Complete Spatial Randomness (CSR) hypothesis, given two sets of points—a set of type A points (e.g., bus stops) and a set of type B points (e.g., crimes) on a street network, L. The CSR hypothesis proposes that points are independently and identically distributed according to a uniform distribution over the street network, implying that the configuration of type A points does not affect the distribution of type B points. The set of type A points (deterministic spatial events, like bus stops) are deterministically placed on street network, L, with a fixed number, n a . The set of type B points (stochastic spatial events, like crimes) are generated according to a stochastic point process on street network, L, with a fixed number, n b .
The Network Cross K Function is defined as:
where n a is the number of bus stops, and n b is the number of crime points, L is the total street network, l T represents the total length of all the streets in the defined network, and t denotes network distance. Network distance is defined as the shortest path between two points on L.
Monte Carlo simulations are performed to generate a series of random distributions of points restricted to the street network resulting in an expected network K value, K ab(t). In a random distribution of n points in a street network, each point has an equal opportunity to occur at any location in the network; the presence of one point at a certain location does not impact the probability of other points occurring at any location in the network. The Network Cross K-Function involves extensive computation to simulate the expected number of points under CSR.
After a series of K ab(t) estimates are obtained, they are ranked from high to low and then compared to the observed Network Cross K Function, K ab(t). By comparing the simulated (K ab(t)) and observed (K^ ab(t)) Network Cross K Functions, we can assess whether the deterministic points (e.g., bus stops) exert a statistically identifiable influence on the distribution of stochastic points (e.g., crime events). If the observed K^ ab(t) is greater than the simulated K ab(t), we can infer that the crime points are clustered around, and thus attracted to bus stops; if K^ ab(t) is less than K ab(t), then the crime points are repelled by bus stop locations.
Data and Measures
Below we examine data on all incidents of gun violence in the city of Newark, NJ (excluding heavy industrial areas, the Port of Newark, and the Newark International Airport) during 2012. Newark is the largest city in the state of New Jersey with a population of more than 250,000 (United States Census Bureau 2012). It is a city that has experienced considerable problems of drugs, gangs and violence (Fried 2008; Murr and Noonoo 2007). Concerted efforts to tackle these problems have been undertaken through various civic administrations, yet violent crime remains markedly high (FBI 2010; New Jersey State Police, n.d.). In total, we distinguish eight types of physical features in operation during 2012 that may serve as environmental risk factors for gun shootings due to their known crime generating properties: foreclosures, liquor stores, middle and high schools, bus stops, grocery stores, take out eateries and fast food restaurants, gas stations, and laundry and dry cleaning stores.
Spatial location (point) data on each physical feature were collected from three sources: the City of Newark, New Jersey Transit Corporation, and the Newark Police Department. New Jersey Transit provided the locations for all 895 bus stops. According to data from the City of Newark, there were 774 foreclosed properties, 87 liquor stores, 68 middle and high schools, 223 grocery stores, 43 take out eateries and fast food restaurants, 36 gas stations, and 37 laundry and dry cleaning stores located in the city in 2012. Each of the addresses for these physical features were geocoded prior to analysis.Footnote 1 In addition, gun violence (shooting) incident locations (including street addresses and ZIP codes) were obtained from the Newark Police Department. The data include both fatal and nonfatal shootings: gun homicides, aggravated assaults with guns, and armed robberies.Footnote 2 All address-level data were geocoded to street centerline shapefiles obtained from the ESRI 2012 TIGER Files.
Analyses and Results
Identifying the Attractors of Gun Violence
The street network of Newark represents the actual travel space within the confines of the city, excluding impediments such as rivers and inaccessible land. To measure the distance between physical features and gun violence in this study, then, we calculate the street network distance (or the shortest path distance) along those street networks between events. The Network Cross K FunctionFootnote 3 identifies the specific physical features that serve as significant attractors of shooting by comparing the cumulative numbers of observed shootings within a continuous range of distances around physical features against the cumulative numbers of points in a random distribution within the same distances. The computational procedures are as follows:
-
1.
For observed data, compute the network distance between each liquor store, for example, and each shooting.
-
2.
Create buffers for each unit of distance (e.g., 1 foot through 3000 ft)Footnote 4 from liquor store locations and compute the number of shootings located within each buffer.
-
3.
Employ Eq. 1 to compute the observed Network Cross K Function.
-
4.
Use a Monte Carlo simulationFootnote 5 to simulate uniformly distributed shooting points within the street network of Newark.
-
5.
Compute the number of simulated shooting points within each distance buffer.
-
6.
Use Eq. 1 to calculate the simulated Network Cross K Function.
-
7.
Identify attractive, repellent, or random patterns in the locations of observed shootings vis-à-vis liquor store locations by comparing the observed points to the simulated random point pattern. If there are significantly more shootings in the observed pattern than in the simulated pattern at a specified distance band, then the presence of shootings is locationally dependent on (or attracted to) liquor stores at the specified distance; otherwise, the shootings are said to be random if the observed and simulated distributions are not statistically different, or dispersed if there are fewer shootings in the observed distribution than in the simulated distribution.
The procedure above is repeated for each physical feature of interest. Figures 1 and 2 present the results of the Network Cross K Function between shootings and eight types of physical features of place. Each graph depicts: (1) the observed curve (solid line) representing the clustering of shootings at continuous distances according to Eq. 1, (2) the mean expected results according to Eq. 1 within each distance band under the Complete Spatial Randomness (CSR) hypothesis (dashed line), and (3) the upper and lower envelop curves under the CSR hypothesis (dotted curves). The X-axis provides the continuous network distances ranging from 1 to 3000 ft and the Y-axis provides the average cumulative number of shootings observed as one moves further away, along the street network, from the locations of the physical features.
Network Cross K Function identifying attractors of gun violence in Newark, NJ 2012
Network Cross K Function identifying non-attractors of gun violence in Newark, NJ 2012
Four physical features can be described as statistically significant shooting attractors in Newark; these include liquor stores, grocery stores, bus stops, and foreclosures (see Fig. 1). As depicted in each graph, the observed curve falls outside and above the CRS hypothesis envelope, indicating a statistically significant clustering pattern of shootings around the associated physical feature. Said differently, these features attract shootings. When the observed curve falls within the CSR curve envelope, the observed and the simulated random shooting distributions do not vary. Thus, Fig. 2 shows that four physical features of place failed to attract shootings: these are gas stations, take out eateries and fast food restaurants, laundry or dry cleaning stores, and middle and high schools.
The graphs of shooting attractors (Fig. 1) also indicate that the distribution of shootings are locationally dependent on liquor stores, grocery stores, bus stops, and foreclosures, at all distances up to and including the cutoff distance of 3000 ft. That is, the observed curve (which is additive and thus always increases as the distance buffer gets larger) in each case falls outside and above the CSR curve throughout the full range of distance (from 1 to 3000 ft). Note that significant spatial attraction is actually apparent over much larger network distances. By way of illustration, Fig. 3 shows that it is not until upwards of 30,000 ft that the attractive effects of grocery stores on shootings dissipates entirely.
Network Cross K Function: the attraction of grocery stores to shootings through 40,000 ft
Comparison to Planar Analysis
The traditional approach to analyzing spatial relationships utilizes an unbounded continuous plane, rather than a network plane. To demonstrate the limitations of the traditional approach, the Planar Cross K Function was calculated on an unbounded plane to examine the spatial dependence of gun violence on each of the eight physical features. The Planar Cross K Function compares the accumulated number of actual shootings within a certain distance of liquor stores, for example, with that in simulated random patterns on the planar plane. This traditional strategy thus mirrors the network approach, with the exception that spatial data are analyzed on a continuous plane and the extent of influence is measured by Euclidean distance.
The planar analyses presented in Figs. 4 and 5 were conducted in R.Footnote 6 The “kcross” function in the R package spatstat was used to run the Planar Cross K Function (Baddeley and Turner 2005). Like the Network Cross K Function, the computational procedures for the Planar Cross K Function follow the same steps, with the exception that Euclidean distance is substituted for network distance and the Ripley’s Cross K Function is substituted for the Network Cross K Function. As these graphs illustrate, the use of planar methods leads to major differences in the results and the conclusions that can be drawn about the strength and extent of spatial influence that physical features have on gun violence. It is noteworthy that, according to the planar analyses, none of these features significantly attract gun violence, and many are even shown to repel it. The variance in results between our network and planar analyses echo the conclusions of Lu and Chen (2007), who find widely disparate effects in the performance of K-functions on street networks versus planar planes. Failure to analyze network-constrained events on a network plane using network distance will thus generate substantively inaccurate estimates of spatial influence.
Planar Cross K Function: the spatial influence of physical features on shootings, Newark NJ 2012
Planar Cross K Function: the spatial influence of physical features on shootings, Newark NJ 2012
Calculating Change Points in Spatial Influence
Returning to the results of the Network Cross K function, it is possible calculate shooting densities within specified network distances from each of the four physical features that serve as significant crime attractors to evaluate the decay in attraction over distance. To measure shooting density, we simply count the number of shootings within a specified distance buffer of each physical feature and divide by the total length of the streets encompassed within that buffer.Footnote 7 First we calculate shooting densities at network distances of 5 ft, followed by larger and larger buffers adding an additional 10 ft consecutively thereafter, through 1005 ft (i.e. 5, 15, 25, 35,…1005 ft).Footnote 8 Preliminary analyses showed that significant changes in spatial influence occurred only within shorter distances and failed to undergo modifications after about 400 ft. Thus, we set 1005 ft as the outside distance boundary to visually capture both specific shifts in, and the point at which a distinct flattening trend in the spatial influence of the feature becomes apparent. All of the computational programming for these procedures were conducted in MATLAB.
Figure 6 illustrates the spatial influence patterns for each identified shooting attractor (e.g., liquor stores, grocery stores, bus stops, and foreclosures). In all cases, shooting density decreases as network distance from the physical features increases. Consistent with past research, the strength of spatial influence is uniformly strongest at shorter distances, initially decreasing rapidly and then dissipating more slowly through 1005 ft. Importantly, however the magnitude of spatial influence and its pattern of decay vary by physical feature. In each case, the decay pattern approximates a nonlinear continuous function, which is observable from our computations of spatial influence at each ten-foot distance band on the continuous street network plane. To better specify the precise distances at which a statistical shift in the spatial influence of crime attractors is observed (that is, to gauge identifiable inflection points), we estimate a segmented regression model using the Joinpoint Regression Program (Kim et al. 2000; Ratcliffe 2012). Segmented regression is a nonlinear regression method that calculates identifiable change points in coefficient effects based on a regression equation of continuous segments.Footnote 9
Decay in density of shootings from attractors of gun violence, Newark NJ 2012
Table 1 presents the results of the change point models in tabular form for shooting attractors. The optimal model for liquor stores has two change points, one at 25 ft and one at 155 ft in network distance. Similarly, a 2-change point model is optimal for grocery stores, with change points of 25 and 255 ft. For bus stops, a 3-change point model is optimal (with change points at 25, 65, and 125 ft). By contrast, the optimal foreclosure model has only one change point at 25 ft. All parameters are statistically significantFootnote 10 and, for each physical feature, the absolute values of the initial slopes are most pronounced while the effect size in the second segment decreases sharply. This suggests that shooting densities are highest within the immediate proximity of shooting attractors and then decline precipitously with path distance along street networks, although at varying rates.
According to the results, grocery stores largely concentrate gun shootings within 255 ft, but the effect is steepest within 25 ft of a store (slope 1 = − 7.263; see Table 1 and Fig. 6). Between 25 and 255 ft, the effect size declines to -.069. Beyond the second change point at 255 ft from a grocery store, the magnitude of the spatial influence of grocery stores on shooting density decays to virtually zero (slope 3 = − .001). The spatial influence of liquor stores on shooting distributions is similar to grocery stores, whereas bus stops have a smoother distance decay than do the other shooting attractors. Finally, for foreclosures, the spatial influence on shootings is the highest within 25 ft but, beyond 25 ft, the slope coefficient dramatically shrinks. Thus, the extent of the spatial influence of foreclosures on shooting density is fairly small (constrained within 25 ft of the foreclosure), and flattens considerably thereafter. The import of the finding that the strongest effects operate within 25 ft of all attractors, before declining markedly, is magnified when we consider that recent studies using street network approaches treat a street segment as the unit-of-analysis (Davies and Johnson 2015; Groff 2014; Weisburd et al. 2012). Street segments often exceed 200 or 300 ft or more in length. The more precise operationalization of network distance from physical feature point locations used above shows that the major spatial influence of physical features on gun crime operates at a scale that is considerably smaller than the micro street segment.
To summarize, the results of quantifying the spatial effects of physical features on shooting density indicate that the spatial influence of every crime attractor is strongest at very short distances, followed by a slow decline at a much lower rate. On the whole, the magnitude of the effects are largest within 25 ft and, while most of these physical features retain significance well in excess of 10 blocks away, the spatial influence shrinks to virtually zero as distance increases. Nonetheless, the segmented regression results clarify important variation in the strength and extent of spatial influence both across physical feature types and within types over street network distance. These effects are identifiable only when the analyses properly estimate the distance of network-constrained events along street networks rather than as the crow flies.
Discussion
Reliance on spatial data and spatial econometric techniques has gained considerable influence in criminology over the past 15–20 years. These methods enable scholars to evaluate the geographic effects of place on criminal activity patterns to better deploy police to problem areas (Braga et al. 2014; Weisburd et al. 2012) and to forecast crime risk as a function of place-based characteristics (Caplan et al. 2011), resulting in important theoretical and policy-related breakthroughs. Yet almost all of the research that takes space seriously treats cities as unbounded planes and measures distance as the crow flies, potentially biasing the findings and associated conclusions that can be drawn. This study employs a spatial analytic method that properly organizes networked spatial data along a street network plane. Such a measurement strategy is important, as criminal events and the physical features that attract those events are situated in or alongside streets. To accurately assess the attraction of crime to physical features of place then, scholars must consider that routes of travel, and both stochastic and deterministic events, are largely constrained by streets in urban areas rather than situated on unconstrained continuous planes. Measuring the shortest path distances between crimes and the physical features that attract those events on a street network thus generates less biased and more accurate results.
The findings of this study show that only four of the eight physical features examined as potential shooting attractors in Newark exerted a spatial influence on shootings that significantly varied from simulated random distributions during 2012; these are liquor stores, grocery stores, bus stops, and foreclosures. Each of these features may thus justifiably be described as criminogenic. By contrast, shooting density was unrelated to the location of gas stations, take out eateries and fast food restaurants, laundry and dry cleaning stores, and middle and high schools. At least during 2012, these latter places failed to significantly attract gun violence in Newark. Consistent with Tobler’s (1970, pp 236) first law of geography, which states that “everything is related to everything else, but near things are more related than distant things,” we find that the spatial influence of criminogenic physical features are strongest at very short distances. In all cases, the findings showed a rapid decrease in the strength of attraction as one moves further away from the feature along street networks, followed by a slower decline generating a much weaker, although still significant, influence.
The key insight of this research lies in proving the utility of a network-based measurement approach for estimating the attraction of stochastic spatial events (e.g., crimes) to deterministic spatial events (e.g., physical features of place) using distance measures that more accurately reflect the constrained nature of travel routes along street networks in cities. This network strategy produces more reliable and accurate results than the traditional planar methods employed in crime pattern analysis precisely because it captures the expected travel routes of victims and offenders along street networks. By testing the one-directional cumulative effects of physical features on gun shootings at all the scales of distance (e.g., 1 ft, 2 ft, 3 ft, …, through the end of the study plane; Lu and Chen 2007), the Network Cross K function can thus detect very subtle changes in the strength and extent of the spatial influence of deterministic spatial events on stochastic spatial events. Consequently, the Network Cross K Function more precisely estimates the presence and effect sizes of crime attractors in crime pattern theory.
Notes
- 1.
The geocoding hit rates for all data were in excess of 85 %, satisfying the threshold of a reliable matching process (Ratcliffe 2004).
- 2.
The authors thank Dr. Leslie Kennedy for generously providing access to the 2012 Newark crime data and for helpful comments on earlier versions of this paper.
- 3.
The Network Cross K Function is calculated with the SANET software package developed by Okabe et al. (1995).
- 4.
For ease of illustration, we compute the Network Cross K Function up to 3000 ft from the physical feature in Newark because the average size of one street block is approximately 280 ft. This means that anything occurring outside 10 blocks is not considered when measuring degree of attraction. While the specific outside distance buffers can vary by researcher preferences and city characteristics, research has generally shown that the effects of physical features are strongest in the immediate vicinity of the feature and fall rather dramatically thereafter (Fagan and Davies 2000; Groff 2014; McNulty and Holloway 2000; Ratcliffe 2012).
- 5.
Unlike other approaches, the Monte Carlo simulation is restricted within the street networks of Newark, rather than across the whole plane of Newark. For each simulated Network Cross K Function analysis, a fifty times of Monte Carlo simulation is conducted within each distance band to obtain the expected distributions. In a random distribution, each point event has an equal probability of occurrence at any location in space; the presence of one point event at a location does not impact the possibility that other point events will occur. Monte Carlo simulation relies on repeated trials to produce sufficient output for generalization.
- 6.
The XY coordinates of all the shooting incidents, physical features’ locations, and the boundaries of the study area were first calculated in ArcGIS using the North American Datum 1983 State Plane New Jersey 2900 ft projected coordinate system and then exported to R.
- 7.
To the extent that city street widths vary, some minor error may be introduced in our decision to treat street length rather than total area of streets as the denominator. Nonetheless, city streets tend to be reasonably similar in width, and thus the total area of streets within a specified distance buffer should be largely proportional to the cumulative length of the streets within the same buffer.
- 8.
Each shooting point is counted only once within a scale of distance, even if it is within the range of multiple liquor stores. Consequently, a shooting that occurs within 300 ft of multiple liquor stores is counted as occurring within 300 ft of only the most proximate liquor store to the shooting.
- 9.
The segmented regression model is estimated for the observations (x1, y1), … (xn , yn ), where x1 < … < xn represent the network distance variable. Yi , where i = 1, 2, …, n is the response variable or, in this case, shooting density. The regression equation can be written as:
E[y|x] = \beta_{0} + \beta_{1} x + \gamma_{1} (x - \tau_{1} )^{ + } + \cdots + \gamma_{n} (x - \tau_{k} )^{ + }where β0, β1, γ1, …, γn are regression coefficients and the τk , k = 1, 2, …, n, n < N, is the kth unknown change point in which (xi − τk )+ = (xi − τk ) if (xi − τk ) > 0. To determine change points, we use the grid search method, rather than Hudson’s method, because it is computationally more efficient. In these analyses, the minimum number of observations between two change points was set at four. To find the optimal model (i.e. the optimal number of change points and the optimal locations of those change points), the Bayesian Information Criterion (BIC) is used. The model with the minimum BIC is the best fitting model.
- 10.
The shooting densities reported in Table 1 are multiplied by 100 to magnify the slope values for ease of interpretation; this is necessary when the values of slopes are very small.
References
Baddeley A, Turner R (2005) Spatstat: an R package for analyzing spatial point patterns. J Stat Softw 12:1–42
Bernasco W, Block R (2011) Robberies in Chicago: a block-level analysis of the influence of crime generators, crime attractors, and offender anchor points. J Res Crime Delinq 48:33–57
Braga AA, Papachristos AV, Hureau DM (2014) The effects of hot spots policing on crime: an updated systematic review and meta-analysis. Justice Q 31:633–663
Brantingham PJ, Brantingham PL (1995) Criminality of place: crime generators and crime attractors. Eur J Crim Policy Res 3:1–26
Caplan JM, Kennedy LW, Miller J (2011) Risk terrain modeling: brokering criminological theory and GIS methods for crime forecasting. Justice Q 28:361–381
Cohen L, Felson M (1979) Social change and crime rate trends: a routine activity approach. Am Sociol Rev 44:588–608
Davies T, Johnson SD (2015) Examining the relationship between road structure and burglary risk via quantitative network analysis. J Quant Criminol 31:481–507
Fagan J, Davies G (2000) Crime in public housing: two-way diffusion effects in surrounding neighborhoods. In: Goldsmith V, McGuire PG, Mollenkopf JH, Ross TA (eds) Analyzing crime patterns: frontiers of practice. Sage Publications, Thousand Oaks, pp 121–135
FBI (2010) Crime in the United States by Metropolitan Statistical Area, 2010. Crime 2010
Fried C (2008) America’s safest city: Amherst, NY; The most dangerous: Newark, NJ. Money Magazine
Gorman D, Speer PW, Gruenewald PJ, Labouvie EW (2001) Spatial dynamics of alcohol availability, neighborhood structure and violent crime. J Stud Alcohol 62:628–636
Grannis R (1998) The importance of trivial streets: residential streets and residential segregation. Am J Sociol 103:1530–1564
Griffiths E, Tita G (2009) Homicide in and around public housing: is public housing a hotbed, a magnet, or a generator of violence for the surrounding community? Soc Probl 56:474–493
Groff E (2014) Quantifying the exposure of street segments to drinking places nearby. J Quant Criminol 30:527–548
Groff E, Lockwood B (2014) Criminogenic facilities and crime across street segments in Philadelphia: uncovering evidence about the spatial extent of facility influence. J Res Crime Delinq 51:277–314
Kennedy LW, Caplan JM, Piza EL, Buccine-Schraeder H (2015) Vulnerability and exposure to crime: applying risk terrain modeling to the study of assault in Chicago. Appl Spat Anal. doi:10.1007/s12061-015-9165-z
Kim H, Fay MP, Feuer EJ, Midthune DN (2000) Permutation tests for join point regression with applications to cancer rates. Stat Med 19:335–351
Krause EF (1975) Taxicab geometry. Addison-Wesley, California
LaGrange T (1999) The impact of neighborhoods, schools, and malls on the spatial distribution of property damage. J Res Crime Delinq 36:393–422
Levine N (2005) CrimeStat III: A Spatial Statistics Program for the Analysis of Crime Incident Locations (version 3.0). Ned Levine & Associates, Houston, TX.; National Institute of Justice, Washington, DC
Levine N, Wachs M, Shirazi E (1986) Crime at bus stops: a study of environmental factors. J Archit Plan Res 3:339–361
Lipton R, Gruenewald PJ (2002) The spatial dynamics of violence and alcohol outlets. J Stud Alcohol 63:187–195
Lu Y, Chen X (2007) On the false alarm of planar K-function when analyzing urban crime distributed along streets. Soc Sci Res 36:611–632
McNulty TL, Holloway SR (2000) Race, crime, and public housing in Atlanta: testing a conditional effect hypothesis. Soc Forces 79:707–729
Murr A, Noonoo J (2007) A return to the bad old days? Newsweek, August 17, 2007
Okabe A, Yamada I (2001) The K-Function method on a network and its computational implementation. Geogr Anal 33:271–290
New Jersey State Police (n.d.) Operation Cease Fire. Retrieved from: http://www.njsp.org/divorg/invest/operation-cease-fire.html
Okabe A, Yomono H, Kitamura M (1995) Statistical analysis of the distribution of points on a network. Geogr Anal 27:152–175
Ratcliffe JH (2004) Geocoding crime and a first estimate of a minimum acceptable hit rate. Int J Geogr Inf Sci 18:61–72
Ratcliffe JH (2012) The spatial extent of criminogenic places on the surrounding environment: a change-point regression of violence around bars. Geogr Anal 44:302–320
Ratcliffe JH, Rengert GF (2008) Near repeat patterns in Philadelphia shootings. Secur J 21:58–76
Roncek DW, Faggiani D (1985) High schools and crime. Sociol Q 26:491–505
Roncek DW, Francik JMA (1981) Housing projects and crime. Soc Probl 29:151–166
Roncek DW, Maier PA (1991) Bars, blocks, and crimes revisited: linking the theory of routine activities to the empiricism of “hot spots”. Criminology 29:725–753
SANET. A Spatial Analysis along Networks (Ver. 4.1). Atsu Okabe, Kei-ichi Okunuki and SANET Team, Tokyo, Japan
Scribner RA, MacKinnon DP, Dwyer JH (1995) The risk of assaultive violence and alcohol availability in Los Angeles County. Am J Public Health 85:335–340
Smith W, Frazee SG, Davison E (2000) Furthering the integration of routine activity and social disorganization theories: small units of analysis and the study of street robbery as a diffusion process. Criminology 38:489–523
Stucky TD, Ottensmann JR (2009) Land use and violent crime. Criminology 47:1223–1264
Tobler WR (1970) A computer movie simulating urban growth in the Detroit region. Econ Geogr 46:234–240
Townsley M, Sidebottom A (2010) All offenders are equal, but some are more equal than others: variation in journeys to crime between offenders. Criminology 48:897–917
United States Census Bureau (2012) The Counties and Most Populous Cities and Townships in 2010 in New Jersey: 2000 and 2010
Weisburd D, Groff ER, Yang S (2012) The criminology of place: street segments and our understanding of the crime problem. Oxford University Press, Oxford
Yamada I, Thill JC (2004) Comparison of planar and network K-functions in traffic accident analysis. J Transp Geogr 12:149–158
Author information
Affiliations
School of Criminal Justice, Rutgers University, 123 Washington St., Newark, NJ, 07102, USA
Jie Xu & Elizabeth Griffiths
Corresponding author
Correspondence to Jie Xu.
Rights and permissions
About this article
Cite this article
Xu, J., Griffiths, E. Shooting on the Street: Measuring the Spatial Influence of Physical Features on Gun Violence in a Bounded Street Network. J Quant Criminol 33, 237–253 (2017). https://doi.org/10.1007/s10940-016-9292-y
Published30 March 2016
Issue DateJune 2017
Share this article
Anyone you share the following link with will be able to read this content:
Provided by the Springer Nature SharedIt content-sharing initiative
Keywords
- Spatial influence
- Physical features of place
- Street network
- Network Cross K Function
- Gun violence
Recommend
About Joyk
Aggregate valuable and interesting links.
Joyk means Joy of geeK