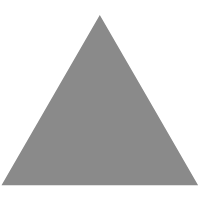
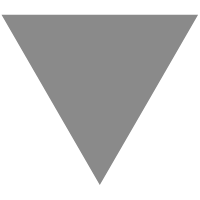
Speeding up the Sieve of Eratosthenes with Numba
source link: https://www.tuicool.com/articles/hit/6zAfe2n
Go to the source link to view the article. You can view the picture content, updated content and better typesetting reading experience. If the link is broken, please click the button below to view the snapshot at that time.
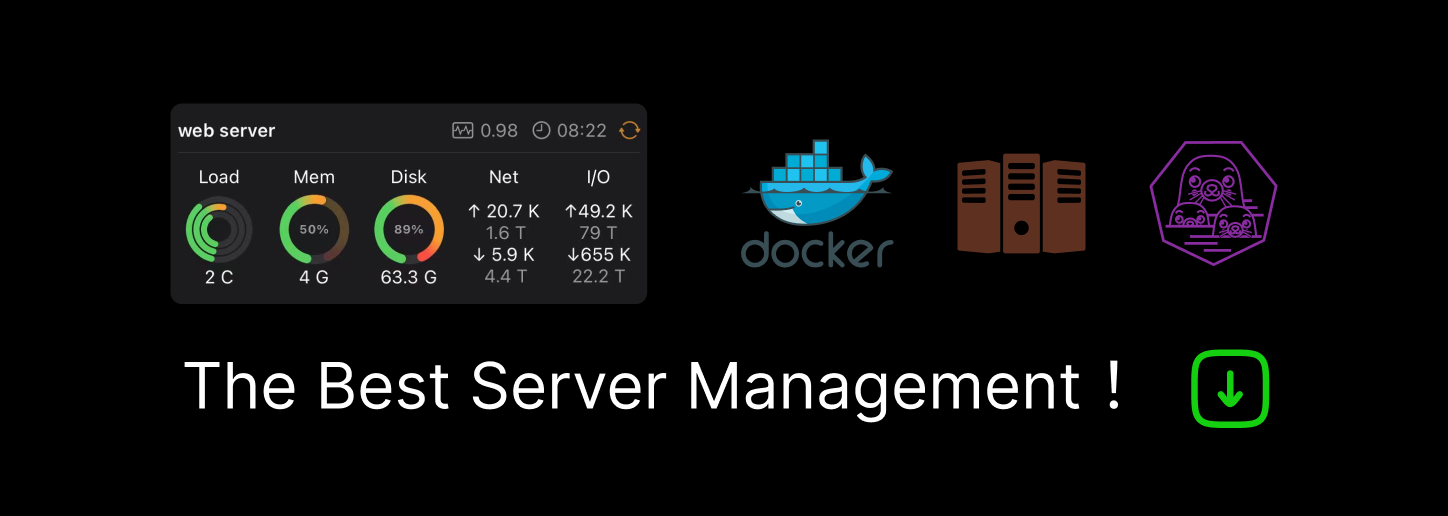
, the remaining numbers are the eagerly-awaited primes. Here's the first version of the implementation I came up with:
def sieve_python(limit): is_prime = [True]*limit is_prime[0] = False is_prime[1] = False for d in range(2, int(limit**0.5) + 1): for n in range(d*d, limit, d): is_prime[n] = False return is_primeThis returns a list is_prime where is_prime[n] is True n
is a prime number. The code is straightforward but it wasn't fast enough for my taste so I decided to time it:
from timeit import timeit def elapse_time(s): s = timeit(s, number=100, globals=globals()) return f'{s:.3f} seconds' print(elapse_time('sieve_python(100000)'))
2.733 seconds2.7 seconds to check 100000 values sounded indeed too slow so I decided to precompile the function with Numba
:
from numba import njit @njit def sieve_python_jit(limit): is_prime = [True]*limit is_prime[0] = False is_prime[1] = False for d in range(2, int(limit**0.5) + 1): for n in range(d*d, limit, d): is_prime[n] = False return is_prime sieve_python_jit(10) # compilation print(elapse_time('sieve_python_jit(100000)'))
0.158 secondsThe only addition to the previous version is the decorator @njit and this simple change resulted in a whopping 18x speed up! However, Michal
shared with me some code making me notice that combining Numba with the appropriate Numpy data structures leads to impressive results so this implementation materialized:
import numpy as np @njit def sieve_numpy_jit(limit): is_prime = np.full(limit, True) is_prime[0] = False is_prime[1] = False for d in range(2, int(np.sqrt(limit) + 1)): for n in range(d*d, limit, d): is_prime[n] = False return is_prime sieve_numpy_jit(10) # compilation print(elapse_time('sieve_numpy_jit(100000)'))
0.096 seconds
The speed up respect to the first version is 27x!
Lessons learned:
- Using Numba is very straightforward and a Python function written in a decent manner can be speeded up with little effort.
- Python lists are too heavy in some cases. Even with pre-allocation of the memory they can't beat Numpy arrays for this specific task.
- Assigning types correctly is key. Using a Numpy array of integers instead of bools in the function sieve_numpy_jit would result in a slow down.
Recommend
-
18
Numba is a just-in-time compiler for Python that works best on code that uses NumPy arrays and functions, and loops. The most common way to use Numba is through its collection of decorators that can be applied to your func...
-
9
RAGANWALD.COM Implementing the Sieve of Eratosthenes with Functional Programming Programming interviews often include a “Fizzbuzz” test,...
-
6
The sieve of Eratosthenes finds all prime numbers up to a given limit. Method The algorithm st...
-
11
In this article, we will learn to implement the Sieve of Eratosthenes using C++. If you’re a competitive programmer, most probably you’d be familiar with this term. But if you’re not, sit back and relax, we’ll see in the following sections wh...
-
9
Large Prime Check Using The Sieve Of Eratosthenes in C++ Filed Under: C++In this article, we will learn...
-
13
Prime Factorization Using The Sieve Of Eratosthenes in C++ Filed Under: JavaToday we will learn prime factoriz...
-
7
[JavaScript] Sieve of Eratosthenes April 28, 2018 JavaScript implementation...
-
7
Online Sieve of Eratosthenes Demo via Go and Vue.js May 01, 2018 Onli...
-
21
[Vue.js] Online Sieve of Eratosthenes Demo May 02, 2018
-
7
[Golang] Sieve of Eratosthenes April 17, 2017 Go implementation of
About Joyk
Aggregate valuable and interesting links.
Joyk means Joy of geeK