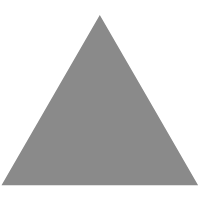
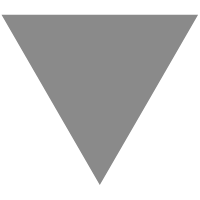
本是同根生,相煎何太急-用Google语音识别API破解reCaptcha验证码 | WooYun知识库
source link:
Go to the source link to view the article. You can view the picture content, updated content and better typesetting reading experience. If the link is broken, please click the button below to view the snapshot at that time.
本是同根生,相煎何太急-用Google语音识别API破解reCaptcha验证码
from:http://www.debasish.in/2014/04/attacking-audio-recaptcha-using-googles.html
0x00 背景
关于验证码和验证码破解的入门,请看:http://drops.wooyun.org/tips/141
什么是reCaptcha?
reCaptchas是由Google提供的基于云的验证码系统,通过结合程序生成的验证码和较难被OCR识别的图片,来帮助Google数字化一些书籍,报纸和街景里的门牌号等。
reCaptcha同时还有声音验证码的功能,用来给盲人提供服务。
0x01 细节
中心思想:
用Google的Web Speech API语音识别来破解它自己的reCaptcha声音验证码.
下面来看一下用来语音识别的API
Chrome浏览器内建了一个基于HTML5的语音输入API,通过它,用户可以通过麦克风输入语音,然后Chrome会识别成文字,这个功能在Android系统下也有。如果你不熟悉这个功能的话这里有个demo:
https://www.google.com/intl/en/chrome/demos/speech.html
我一直很好奇这个语音识别API是如何工作的,是通过浏览器本身识别的还是把音频发送到云端识别呢?
通过抓包发现,好像的确会把语音发送到云端,不过发送出去的数据是SSL加密过的。
于是我开始翻Chromium项目的源码,终于我找到了有意思的地方:
http://src.chromium.org/viewvc/chrome/trunk/src/content/browser/speech/
实现过程非常简单,首先从mic获取音频数据,然后发送到Google的语音识别Web服务,返回JSON格式的识别结果。 用来识别的Web API在这里:
https://www.google.com/speech-api/v1/recognize
比较重要的一点是这个API只接受flac格式的音频(无损格式,真是高大上)。
既然知道了原理,写一个利用这个识别API的程序就很简单了。
#!bash
./google_speech.py hello.flac
源代码:
#!python
'''
Accessing Google Web Speech API using Pyhon
Author : Debasish Mandal
'''
import httplib
import sys
print '[+] Sending clean file to Google voice API'
f = open(sys.argv[1])
data = f.read()
f.close()
google_speech = httplib.HTTPConnection('www.google.com')
google_speech.request('POST','/speech-api/v1/recognize?xjerr=1&client=chromium&lang=en-US',data,{'Content-type': 'audio/x-flac; rate=16000'})
print google_speech.getresponse().read()
google_speech.close()
研究了一下reCaptcha的语音验证码后,你会发现基本上有两种语音验证码,一种是非常简单的,没有加入很多噪音,语音也很清晰。另外一种是非常复杂的,故意加了很多噪音,连真人很难听出来。这种验证码里面估计加了很多嘶嘶的噪声,并且用很多人声作为干扰。
关于这个语音验证码的细节可以参考这里https://groups.google.com/forum/#!topic/recaptcha/lkCyM34zbJo
在这篇文章中我主要写了如何解决前一种验证码,虽然我为了破解后一种复杂的验证码也做了很多努力,但是实在是太困难了,即使是人类对于它的识别率也很低。
用户可以把recaptcha的语音验证码以mp3格式下载下来,但是Google语音识别接口只接受flac格式,所以我们需要对下载回来的mp3进行一些处理然后转换成flac再提交。
我们先手工验证一下这样行不行:
首先把recaptcha播放的音频下载成mp3文件。
然后用一个叫Audacity的音频编辑软件打开,如图
把第一个数字的声音复制到新窗口中,然后再重复一次,这样我们把第一位数字的声音复制成连续的两个相同声音。
比如这个验证码是76426,我们的目的是把7先分离出来,然后让7的语音重复两次。
最后把这段音频保存成wav格式,再转换成flac格式,然后提交到API。
#!bash
[email protected] ~/Desktop/audio/heart attack/final $ sox cut_0.wav -r 16000 -b 16 -c 1 cut_0.flac lowpass -2 2500
[email protected] ~/Desktop/audio/heart attack/final $ python send.py cut_0.flac
很好,服务器成功识别了这段音频并且返回了正确的结果,下面就需要把这个过程自动化了。
在自动提交之前,我们需要了解一下数字音频是处理什么原理。
这个stackoverflow的问题是个很好的教程:
http://stackoverflow.com/questions/732699/how-is-audio-represented-with-numbers
把一个wav格式的文件用16进制编辑器打开:
用Python WAVE模块处理wav格式的音频:
wave模块提供了一个很方便接口用来处理wav格式:
#!python
import wave
f = wave.open('sample.wav', 'r')
print '[+] WAV parameters ',f.getparams()
print '[+] No. of Frames ',f.getnframes()
for i in range(f.getnframes()):
single_frame = f.readframes(1)
print single_frame.encode('hex')
f.close()
getparams()函数返回一个元组,内容是关于这个wav文件的一些元数据,例如频道数量,采样宽度,采样率,帧数等等。
getnframes()返回这个wav文件有多少帧。
运行这个python程序后,会把sample.wav的每一帧用16进制表示然后print出来
[+] WAV parameters (1, 2, 44100, 937, 'NONE', 'not compressed')
[+] No. of Frames 937
[+] Sample 0 = 62fe <- Sample 1
[+] Sample 1 = 99fe <- Sample 2
[+] Sample 2 = c1ff <- Sample 3
[+] Sample 3 = 9000
[+] Sample 4 = 8700
[+] Sample 5 = b9ff
[+] Sample 6 = 5cfe
[+] Sample 7 = 35fd
[+] Sample 8 = b1fc
[+] Sample 9 = f5fc
[+] Sample 10 = 9afd
[+] Sample 11 = 3cfe
[+] Sample 12 = 83fe
[+] ....
从输出文件中我们可以看到,这个wav文件是单通道的,每个通道是2字节长,因为音频是16比特的,我们也可以用 getsampwidth()函数来判断通道宽度,getchannels() 可以用来确定音频是单声道还是立体声。
接下来对每帧进行解码,这个16进制编码实际上是小端序保存的(little-endian),所以还需要对这段python程序做一些修改,并且利用struct模块把每帧的值转换成带符号的整数。
#!python
import wave
import struct
f = wave.open('sample.wav', 'r')
print '[+] WAV parameters ',f.getparams()
print '[+] No. of Frames ',f.getnframes()
for i in range(f.getnframes()):
single_frame = f.readframes(1)
sint = struct.unpack('<h', single_frame) [0]
print "[+] Sample ",i," = ",single_frame.encode('hex')," -> ",sint[0]
f.close()
修改完毕后再次运行,输出内容差不多这样:
[+] WAV parameters (1, 2, 44100, 937, 'NONE', 'not compressed')
[+] No. of Frames 937
[+] Sample 0 = 62fe -> -414
[+] Sample 1 = 99fe -> -359
[+] Sample 2 = c1ff -> -63
[+] Sample 3 = 9000 -> 144
[+] Sample 4 = 8700 -> 135
[+] Sample 5 = b9ff -> -71
[+] Sample 6 = 5cfe -> -420
[+] Sample 7 = 35fd -> -715
[+] Sample 8 = b1fc -> -847
[+] Sample 9 = f5fc -> -779
[+] Sample 10 = 9afd -> -614
[+] Sample 11 = 3cfe -> -452
[+] Sample 12 = 83fe -> -381
[+] Sample 13 = 52fe -> -430
[+] Sample 14 = e2fd -> -542
这样是不是更明白了?下面用python的matplotlib画图模块把这些数值画出来:
#!python
import wave
import struct
import matplotlib.pyplot as plt
data_set = []
f = wave.open('sample.wav', 'r')
print '[+] WAV parameters ',f.getparams()
print '[+] No. of Frames ',f.getnframes()
for i in range(f.getnframes()):
single_frame = f.readframes(1)
sint = struct.unpack('<h', single_frame)[0]
data_set.append(sint)
f.close()
plt.plot(data_set)
plt.ylabel('Amplitude')
plt.xlabel('Time')
plt.show()
这个图实际上就是声音的波形图
进一步自动化:
下面这段python程序通过音量不同把音频文件分割成多个音频文件,相当于图片验证码识别中的图片分割步骤。
#!python
'''
简单的基于音量的音频文件分割程序
作用:
1. 简单的降噪处理
2. 识别文件中的高音量部分
3. 根据高音量部分的数目把文件分割成独立文件
'''
import wave
import sys
import struct
import os
import time
import httplib
from random import randint
ip = wave.open(sys.argv[1], 'r')
info = ip.getparams()
frame_list = []
for i in range(ip.getnframes()):
sframe = ip.readframes(1)
amplitude = struct.unpack('<h', sframe)[0]
frame_list.append(amplitude)
ip.close()
for i in range(0,len(frame_list)):
if abs(frame_list[i]) < 25:
frame_list[i] = 0
################################ Find Out most louder portions of the audio file ###########################
thresh = 30
output = []
nonzerotemp = []
length = len(frame_list)
i = 0
while i < length:
zeros = []
while i < length and frame_list[i] == 0:
i += 1
zeros.append(0)
if len(zeros) != 0 and len(zeros) < thresh:
nonzerotemp += zeros
elif len(zeros) > thresh:
if len(nonzerotemp) > 0 and i < length:
output.append(nonzerotemp)
nonzerotemp = []
else:
nonzerotemp.append(frame_list[i])
i += 1
if len(nonzerotemp) > 0:
output.append(nonzerotemp)
chunks = []
for j in range(0,len(output)):
if len(output[j]) > 3000:
chunks.append(output[j])
#########################################################################################################
for l in chunks:
for m in range(0,len(l)):
if l[m] == 0:
l[m] = randint(-0,+0)
inc_percent = 1 #10 percent
for l in chunks:
for m in range(0,len(l)):
if l[m] <= 0:
# negative value
l[m] = 0 - abs(l[m]) + abs(l[m])*inc_percent/100
else:
#positive vaule
l[m] = abs(l[m]) + abs(l[m])*inc_percent/100
########################################################
# Below code generates separate wav files depending on the number of loud voice detected.
NEW_RATE = 1 #Change it to > 1 if any amplification is required
print '[+] Possibly ',len(chunks),'number of loud voice detected...'
for i in range(0, len(chunks)):
new_frame_rate = info[0]*NEW_RATE
print '[+] Creating No. ',str(i),'file..'
split = wave.open('cut_'+str(i)+'.wav', 'w')
split.setparams((info[0],info[1],info[2],0,info[4],info[5]))
# split.setparams((info[0],info[1],new_frame_rate,0,info[4],info[5]))
#Add some silence at start selecting +15 to -15
for k in range(0,10000):
single_frame = struct.pack('<h', randint(-25,+25))
split.writeframes(single_frame)
# Add the voice for the first time
for frames in chunks[i]:
single_frame = struct.pack('<h', frames)
split.writeframes(single_frame)
#Add some silence in between two digits
for k in range(0,10000):
single_frame = struct.pack('<h', randint(-25,+25))
split.writeframes(single_frame)
# Repeat effect : Add the voice second time
for frames in chunks[i]:
single_frame = struct.pack('<h', frames)
split.writeframes(single_frame)
#Add silence at end
for k in range(0,10000):
single_frame = struct.pack('<h', randint(-25,+25))
split.writeframes(single_frame)
split.close()#Close each files
当这个文件被分割成多份之后我们可以简单的把他们转换成flac格式然后把每个文件单独发送到Google语音识别API进行识别。
视频已翻墙下载回来:
Solving reCaptcha Audio Challenge using Google Web Speech API Demo
现在我们已经解决了简单的音频验证码,我们再来尝试一下复杂的。
这个图片是用前面的程序画出来的复杂语音验证码的波形图:
从图里我们可以看到,这段音频中一直存在一个恒定的噪声,就是中间横的蓝色的那条,对于这样的噪声我们可以用标准的离散傅里叶变换,通过快速傅里叶变换fast Fourier transform(挂在高树上的注意了!)来解决。
回到多年前校园中的数字信号处理这门课,让我们在纯洁的正弦波 s(t)=sint(w*t)
上叠加一个白噪声,S(t)=S(t+n)
, F为S的傅里叶变换,把频率高于和低于w的F值设为0,噪声就被这样过滤掉了。
比如这张图里,正弦波的频谱域被分离了出来,只要把多余频率切掉,再逆变换回去就相当于过滤掉部分噪音了。其实自己写这样的过滤器实在太蛋疼了,Python有不少音频处理库并且自带降噪滤镜。
但是就像识别图形验证码一样,噪音(相当于图片里的干扰线和噪点)并不是破解语音验证码的难点,对于计算机来说,最难的部分还是分割,在复杂的语音验证码里,除了主要的人声之外,背景中还有2,3个人在念叨各种东西,并且音量和主要的声音差不多,无法通过音量分离,这样的手段即使对于人类也很难识别的出。
我把目前的代码放在了https://github.com/debasishm89/hack_audio_captcha
这些代码还很原始,有很大改进的余地。
0x02 结论
我把这个问题报给了Google安全团队,他们说这个东西就是这样设计的(苦逼的作者),如果系统怀疑对方不是人是机器的时候会自动提升到高难度验证码,目前Google不打算改进这个设计。
Recommend
About Joyk
Aggregate valuable and interesting links.
Joyk means Joy of geeK