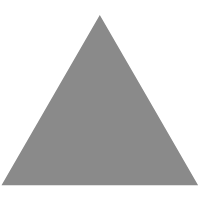
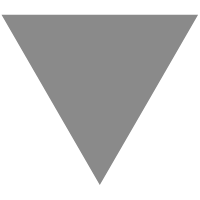
GitHub - kutoga/going_deeper: Going Deeper: Infinite Deep Neural Networks
source link: https://github.com/kutoga/going_deeper
Go to the source link to view the article. You can view the picture content, updated content and better typesetting reading experience. If the link is broken, please click the button below to view the snapshot at that time.
Going Deeper: Infinite Deep Neural Networks
This repository contains the code for the experiments of the following paper-like document: doc/going_deeper.pdf
Summary
The document describes a meta-layer for infinite deep neural networks. It basically wraps a few other layers in a special way that allows the neural network to decide how many sub-layers in the meta-layer should be used. Each sub-layer has its own weights, so the network also decides how many weights should be used. The complete training process may be done with gradient descent-based methods.
Please read doc/going_deeper.pdf for more details.
Library
The repository contains a small library that allows it to use the described meta-layer. The library is based on Keras. The library is very minimal, so not all network architectures may be created with it. A basic model (the model of the first experiment), may be created like this:
# Create the model
n_input_units = 8
n_internal_units = 24
model = TDModel()
model += Input((n_input_units,))
model += Dense(n_internal_units, activation='relu', trainable=False)
# The described meta-layer
model += GInftlyLayer(
# The name
'd0',
# f_i(x)
f_layer=[
lambda reg: Dense(n_internal_units),
lambda reg: GammaRegularizedBatchNorm(reg=reg, max_free_gamma=0.),
lambda reg: Dropout(0.1),
],
# h(x)
h_step=[
lambda reg: Activation('relu')
],
# Regularizers
w_regularizer=(c_l2, w_reg),
f_regularizer=(c_l2, f_reg)#1e-2)
)
model += Dense(1, activation='sigmoid', trainable=False)
# Build the model
model.init(
optimizer='adam',
loss='binary_crossentropy',
metrics=['accuracy']
)
You may try to test the first experiment to get a better feeling for the interface.
Experiments
All experiments are more detailed documented in doc/going_deeper.pdf.
The first experiment uses 8 binary inputs and calculates the XOR-result of them. The used netwok contains only trainable weights in a GInftyLayer
-layer. Tests are done with 0-8 active inputs for the XOR-calculation. Inactive inputs are not used for the XOR-calculation and just get random input values.
It can be assumed that a network with more active inputs for the XOR-computation is more complex and, therefore, requires more sub-layers in the GInftyLayer
-layer. Exactly this can be shown with the given experiment. The w
-value, which basically contains the amount of sub-layers is higher for more active inputs:
The second experiment is conducted on the MNIST-dataset. The used network architecture contains two convolutional GInftyLayer
-layers and one fully connected GInftyLayer
-layer. The test accuracy is up to 99.5 % and it can be seen that the second convolutional GInftyLayer
-layer is the deepest layer. The first convolutional and the fully connected GInftyLayer
-layer have a very low activation. The second convolutional layer has a depth of 2. The weights are visualized on the following plot:
Recommend
About Joyk
Aggregate valuable and interesting links.
Joyk means Joy of geeK