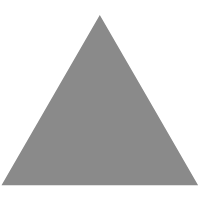
4
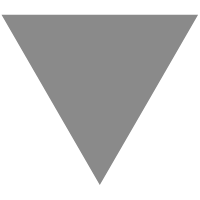
[2402.06184] The boundary of neural network trainability is fractal
source link: https://arxiv.org/abs/2402.06184
Go to the source link to view the article. You can view the picture content, updated content and better typesetting reading experience. If the link is broken, please click the button below to view the snapshot at that time.
Computer Science > Machine Learning
[Submitted on 9 Feb 2024]
The boundary of neural network trainability is fractal
Download PDF HTML (experimental)
Some fractals -- for instance those associated with the Mandelbrot and quadratic Julia sets -- are computed by iterating a function, and identifying the boundary between hyperparameters for which the resulting series diverges or remains bounded. Neural network training similarly involves iterating an update function (e.g. repeated steps of gradient descent), can result in convergent or divergent behavior, and can be extremely sensitive to small changes in hyperparameters. Motivated by these similarities, we experimentally examine the boundary between neural network hyperparameters that lead to stable and divergent training. We find that this boundary is fractal over more than ten decades of scale in all tested configurations.
Comments: | 3 pages, mesmerizing fractals |
Subjects: | Machine Learning (cs.LG); Neural and Evolutionary Computing (cs.NE); Chaotic Dynamics (nlin.CD) |
Cite as: | arXiv:2402.06184 [cs.LG] |
(or arXiv:2402.06184v1 [cs.LG] for this version) | |
https://doi.org/10.48550/arXiv.2402.06184 |
Submission history
From: Jascha Sohl-Dickstein [view email][v1] Fri, 9 Feb 2024 04:46:48 UTC (36,948 KB)
Recommend
About Joyk
Aggregate valuable and interesting links.
Joyk means Joy of geeK