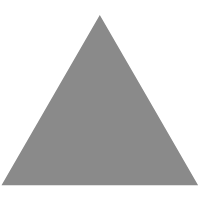
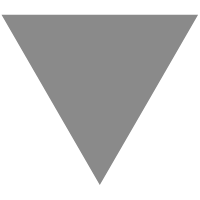
AI automates systemic racism, warns IBM strategist - why enterprises need to act...
source link: https://diginomica.com/ai-automates-systemic-racism-warns-ibm-strategist-why-enterprises-need-act-now
Go to the source link to view the article. You can view the picture content, updated content and better typesetting reading experience. If the link is broken, please click the button below to view the snapshot at that time.
AI automates systemic racism, warns IBM strategist - why enterprises need to act now

Calvin D. Lawrence
An FT report on 27 March claimed that Artificial Intelligence is poised to disrupt 300 million jobs in the US and Europe. That figure was extraordinary, because (according to research site Statista), 158 million Americans were in paid employment in 2022, and roughly the same number full time in the EU, a grand total of 316 million people. So, if the FT is correct, then few jobs won’t be affected - and, by extension, even fewer lives.
In 2023, barely an hour goes by without another press release announcing that AI – especially generative AI, or Large Language Models, such as OpenAI’s GPT-4 – can do something new. Indeed, we’re told they can solve problems that we didn’t even know were problems.
For example, they can now code, run corporate systems, provide high-level consultancy services, determine our healthcare and insurance needs, manage our finances, vet our job applications, plan our next vacation, write speeches and reports, teach school pupils, draw pictures, design objects, make movies, and even compose songs. The list goes on and on.
Or rather, AI can analyze reams of historic human data and generate a derivative work, or a plausible-sounding response to a query – questions such as, ‘Is this person trustworthy and employable?’. Increasingly, the tactical rush to adopt such systems – many of them facile – is fuelled by tools like ChatGPT, the makers of which have encouraged us to see them as fun, trusted playthings.
Epochocal importance
But are they? What if, beneath the surface, some AIs are really doing things that are centuries old: reinforcing prejudice, setting human against human, making assumptions about vulnerable groups, and denying others opportunity – while claiming to do the opposite?
A new book, ‘Hidden in White Sight: How AI Empowers and Deepens Systemic Racism’, says they are doing exactly that – and worse. Published this April, it is poised to become a work of epochal importance: a well-timed warning from inside the system itself.
Across more than 200 pages of personal stories, compelling evidence, and case studies, author Calvin D. Lawrence shows that, all too often, AI can be an engine for exclusion, automating and perpetuating deep societal problems.
More, it is giving racism a veneer of computer-generated trust and veracity, he suggests, which sit atop the decades of historic data that AI needs to function. Data pulled from systems that – often without us realising – may have been trained on years of biased human behaviour, by teams that lack any diversity at all.
In a deeply personal introduction, Lawrence writes:
It’s a painful reality that AI doesn’t fail everyone equally. When tech goes wrong, it often goes terribly for People of Color. That’s not to indicate that the objects of AI’s failure are somehow always pre-determined, but even when the outcome is unintended and unwelcome (even by the perpetrator), we still feel the effects and the impact.
Yet Lawrence isn’t some Luddite with a grudge or a skeptical academic - he is reporting on these problems from the inside, as both a Black American and as a highly experienced computer scientist.
Indeed, the former Distinguished Engineer and Chief Architect for Cognitive Systems at IBM is now that company’s CTO of Responsible and Trustworthy AI. In short, this is a man who knows what he is talking about.
Writing on his own behalf and about the AI sector as a whole – and also for his family, friends, and fellow citizens, he says – Lawrence’s book is a plea to take these issues seriously, and to not expect the industry to police itself. But he adds:
AI is not to be feared. First, however, we must educate ourselves about it and explore the field to eliminate biases against us. It is only from within that we can solve this issue.
A handful of the cases he describes are familiar. They include the COMPAS sentencing guidelines algorithm used in US courts, which was found to recommend tougher sentences for even first-time black offenders, and more lenient ones for some hardened white criminals (thanks to years of historic data that reflected the unequal treatment of black defendants by human judges).
Also broadly familiar are stories about the sensors and computer vision systems that are unable to recognise black skin tones, because they have been calibrated for the greater reflectivity of white skin; the autonomous cars that are more prone to hit a black person because of the same problem, or because they have been trained on data sets of mainly white faces; and the job applicants who are never shortlisted because, in the past, people with similar names, zip codes, and educations were rejected by biased employers.
Revelations
But most cases are not well known. Such as the feedback loop facing Black Americans in one state who are automatically questioned by police for stopping in what has been designated a high-crime area. The more they stop there, the more they are questioned or detained, creating a policing center of gravity where it becomes impossible to escape officers’ original assumptions.
One victim was the author himself, whose flat tire triggered the arrival of three car loads of hostile officers and some aggressive questioning. We all know how these encounters sometimes end for Black males when US police are armed and angry.
Of course, such problems are broadly familiar to Black Britons too – who, Metropolitan Police data shows, are seven times more likely to be stopped and questioned by officers than whites.
Earlier this month a year-long review by Baroness Casey found that London’s police force is “institutionally racist, sexist, and homophobic”. Throw in future automation or AI that analyzes historic data sets from such an organization, and you can begin to see how problems like this might be automated – and thus perpetuated.
Lawrence’s book reveals how this is already happening across many fields. For example, AI tech that listens to callers’ voices on the phone, make assumptions about their ethnicity, and offer or deny them services and products accordingly – leading many black people to ‘try to sound white’ when calling help-desks, suggests Lawrence.
The cumulative impact of all these cases is stark and persuasive. Evoking the Civil Rights movement, he writes:
In his landmark and memorable ‘I Have a Dream’ speech, Martin Luther King Jr proclaimed, ‘The arc of the moral universe is long, but it bends toward justice’.
But he adds:
Most people haphazardly misconstrue the quote to mean that the arc automatically bends toward justice without any outside interference. But the moral universe’s arc is anything but that. It is unbending toward any form of justice apart from its own. No, it only does so because people pull it toward justice. It is an active exercise, not a passive one.
So, Lawrence is putting his own shoulder to the wheel of change.
Need more diversity
To be absolutely clear, no one is accusing AI coders of being inherently racist – least of all the author. Most are quite the reverse, and, like Lawrence, sincerely want to make the world a better, fairer place.
The problem stems, at least in part, from the lack of diversity in AI development teams, he says. All groups unavoidably – and often unconsciously – reflect their members’ perspectives or assumptions.
Put simply, if you want to design technology that works for all society, then all society needs to design it. But it doesn’t, and that is fast becoming a problem. Not just for ethnic minorities, but for all minorities. And for women, who are 50% of the population, yet only comprise 15% of employees in STEM careers.
According to techUK figures, the UK IT industry is roughly 85% male and over 91% white. And, as ‘Hidden in White Sight’ documents from a US perspective, the AI sector is far from an exception - diginomica’s 2020 report ‘Why the AI industry urgently needs more uncomfortable conversations about BAME representation’ explores how countless black coders want to work in the sector, yet are often denied opportunity.
Why now?
So, why did Lawrence – whose three decades of experience embrace entrepreneurship, working in the early years of Java and object programming, and writing a thesis on AI as far back as graduate school – feel compelled to write his book now? He tells me:
Over the last five years in particular, I’ve been working more with Artificial Intelligence, with data and AI, including at IBM Watson, as CTO for Cognitive Computing.
But it was really working in Smart Cities programs, where I was CTO, that I began to see the effects of technologies like facial recognition: a lot of policing apps, for example.
That was probably the first job where I really got the opportunity to work with clients who weren't typical buyers. With mayors, doctors, and attorneys. And that shaped my view of these technologies.
Today, Lawrence is one of the leaders of IBM’s go-to-market strategy for Responsible AI, ensuring that its internal and customer-facing systems are developed and deployed ethically. Despite this, he began to doubt how much impact he has had on the industry as a whole over the course of a long career. And that led to “a personal epiphany”, he says:
I did a lot of self-reflection on so many apps that I've built, and on some of the systems that I’ve architected and been involved with. And many times, to be quite honest, it was clear that I didn't take the perspective of a Person of Color when it came to functional and non-functional requirements.
For example, if I was building an app for a police department, then I was building that out for the police. I didn't really consider how it would be used. I didn't consider the user, or the person who would be impacted.
He adds:
I’ve dedicated a chapter to the concept of ‘no seat at the table’, meaning the lack of diversity in teams, and in discussions about AI technology. It’s a global problem. It impacts everything from the requirements gathering phase, to the design, to the information that we've gotten from clients. Lack of diversity is impacting all that.
For me, the fundamental issue with AI and with technologies like it, is the fact that you don't have the full, proper perspective. Or the right folk involved.
I've worked on hundreds and hundreds of projects, yet I've never worked on a design and development team with more than two People of Color. Never in my whole career. Now, that's not necessarily a statement of intent [for any project], but it is a statement of fact.
The point is that AI needs to work equally well for all members of society, he explains, even if some groups are minorities in that society:
As a person who has worked in AI, and built AI, I understand the importance of training data – of ensuring that you have proper representation. If you don't have that, you don’t just have the problem yourself, but the people who might be impacted have the problem.
It is the nature of a technology like Artificial Intelligence – which is meant to mimic human behaviour and automate it – that if you don’t reflect all human diversity, then you may have both intentional and unintentional consequences.
But it is not just biases in historic data or algorithm design that are the problem, he says, nor simply the lack of diversity in teams – though all are contributing factors. It is also what he calls “data drift”, a concept explored in depth in the book:
Even if you do all the right things, data will move. Data changes constantly. And it will become biased, and it will introduce bias into your processes.
As a result, constant vigilance is essential, he says – particularly when you inadvertently create feedback loops or gravity wells of biased behavior, as suggested by some examples in the book.
If you start with the assumption that a certain neighborhood means more crime and pour police resources into that area, then they will inevitably detect more crime, thus confirming your bias. But this ignores the fact that if you pour officers into any neighborhood, more crimes will be detected. Now add AI, and you have automated racial profiling.
But what can be done about biased data and AI that automates societal problems? The book suggests numerous solutions and essential guardrails – which I will leave you to find for yourself in the text.
But in recent years, regulators in the EU have also begun to focus on the need for ethical AI, while the US federal government has published an AI Bill of Rights, a non-binding statement of ethical principles. So, does Lawrence believe it is now up to regulators and lawmakers to step in? Or should the industry be left to police itself (as some have suggested)? Lawrence argues:
I fundamentally believe that government and policymakers have a critical role. I’m not necessarily a ‘Big Government’ person, but I think in this particular case, it's important. Because it's almost impossible to believe that tech companies will police themselves.
Part of the book talks about this concept of profit versus social responsibility. And that whole chapter, really, is about best practices and guidance to corporate leaders.
But the concept behind AI is all about automation and efficiency. So, the reason someone would want to invest in AI is mainly to automate things and be efficient. First, they want to do things faster and cheaper. And second, they want to do things that they haven't done before. AI gives them the ability to do that.
So, the profit side of that will always be overwhelming. Corporate leaders have responsibility and accountability to their shareholders first, and that’s why profit will always win.
My take
As a huge wave of hype and tactical AI adoption rises, ‘Hidden in White Sight’ arrives like a lifeboat launched by a captain of industry. Its arrival tells us a storm is coming. We should listen.
• ‘Hidden in White Sight: How AI Empowers and Deepens Systemic Racism’ by Calvin D. Lawrence is published by CRC Press, part of the Taylor & Francis group.
Recommend
About Joyk
Aggregate valuable and interesting links.
Joyk means Joy of geeK