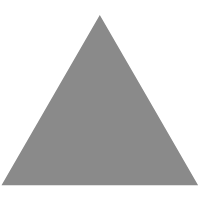
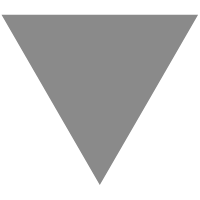
NeRF Differentiable Forward Maps
source link: https://jyzhu.top/NeRF-Differentiable-Forward-Maps/
Go to the source link to view the article. You can view the picture content, updated content and better typesetting reading experience. If the link is broken, please click the button below to view the snapshot at that time.
This is part of my journey of learning NeRF.
2.3. Differentiable Forward Maps
image-20221208175453557
Differentiable rendering
image-20221208181457315
Volume rendering can render fogs. Sphere rendering only render the solid surface, and needs ground truth supervision.? Neural renderer combines the two.
Differentiability of the rendering function itself
- BRDF Shading? details later.
Differentiation itself
Design a neural network with higher order derivatives constraints and therefore directly use its derivative.
image-20221208182302568
For example the Eikonal equation forces the neural network has a derivative as 1. Adding the eikonal loss then promises the neural network valid.
Generally, this kind of problems are: the solutions are constrained by its partial derivatives.
Special: Identity Operator
Reconstruction→1^()→Sensor domainReconstruction==Sensor domain
- Can we obtain a neural network in just one forward, without optimization?
- Can we design special forward maps for specific downstream tasks, eg., classification? Absolutely yes. We can design it to represent a compact representation as the sensor domain. The key idea is to get a differentiable function to map your specific recon and sensor domain.
Recommend
About Joyk
Aggregate valuable and interesting links.
Joyk means Joy of geeK