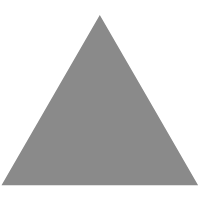
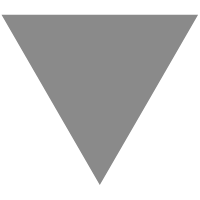
Find External Data for Machine Learning Pipelines
source link: https://www.analyticsvidhya.com/blog/2022/07/find-external-data-for-my-machine-learning-pipelines/
Go to the source link to view the article. You can view the picture content, updated content and better typesetting reading experience. If the link is broken, please click the button below to view the snapshot at that time.
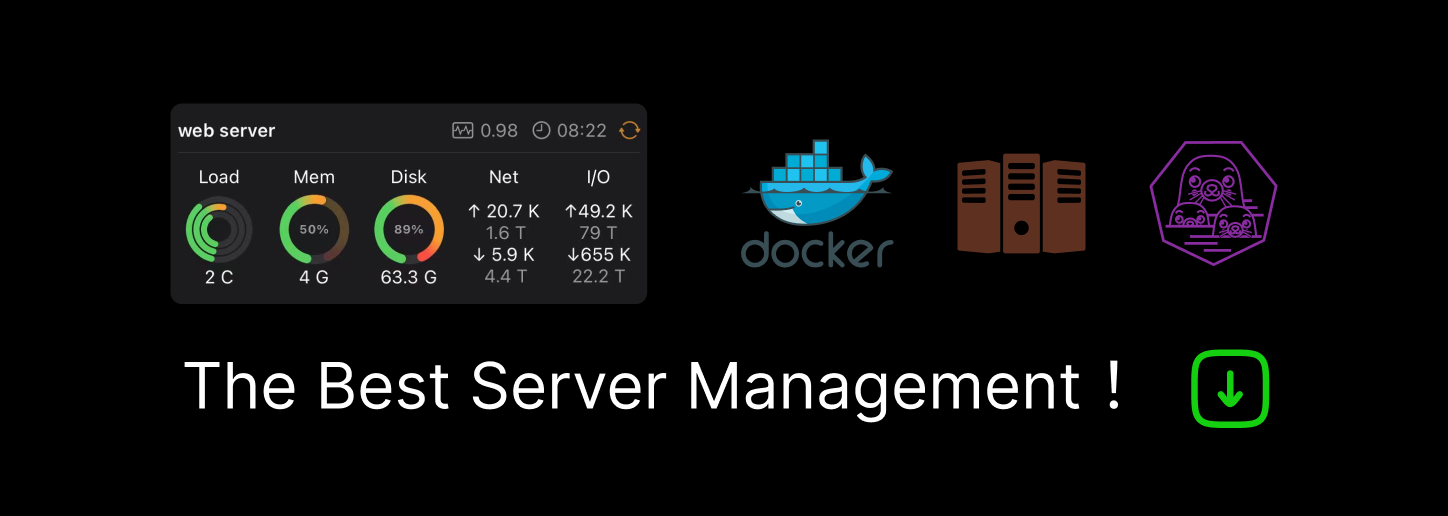
This article was published as a part of the Data Science Blogathon.
Introduction
Machine Learning pipelines are always about learning and best accuracy achievement. And every Data Scientist wants to progress as fast as possible, so time-saving tips & tricks are a big deal as well. That’s why low-code tools are adopted among data scientists. So, there are two major scenarios of external features & data introduction in the creation of machine learning pipelines for Data Scientists:
1. Final improvement of a polished machine learning pipelines
Use it to improve an already polished pipeline (optimized features, model architecture, hyperparameters) with new external features. And you want to answer the simple question – Are there any external data sources and features which might boost accuracy a bit more? However, there is a caveat to this approach: current model architecture & hyperparameters might be suboptimal for the new feature set, even a single new var after introduction. So extra step back for model tuning might be needed.
2. Low-code initial feature engineering – add relevant external features @start
Use it to save time on feature search and engineering. If there are some ready-to-use external features and data, let’s use them to speed up the overall progress. In this scenario always make sense to check that new external features have optimal representation for a specific task and target model architecture. Example – category features for linear regression models should be one-hot-encoded. This type of feature preparation should be done manually in any case. Same as scenario #1, there is a caveat to this approach: many features are not always a good thing – they might lead to dimensionality increase and model overfitting. So you have to check model accuracy improvement metrics after enrichment with the new features and ALWAYS with an appropriate cross-validation strategy.
In this guide, we’ll go with Scenario #1.
Let’s resolve such a task: TPS January 2022, SMAPE as a target metric.
And get the best open notebook for improvement.
To search for useful external data, we’ll use:
- Upgini – Low-code Feature search and enrichment python library for supervised machine learning applications.
Packages
Let's install and import the packages that we will need. %pip install -Uq upgini import pandas as pd import numpy as np import pickle import itertools import gc import math import matplotlib.pyplot as plt import dateutil.easter as easter from datetime import datetime, date, timedelta from sklearn.preprocessing import StandardScaler, MinMaxScaler from sklearn.impute import SimpleImputer from sklearn.model_selection import KFold, GroupKFold, TimeSeriesSplit from sklearn.linear_model import Ridge from sklearn.metrics import mean_squared_error from sklearn.compose import ColumnTransformer from sklearn.pipeline import make_pipeline import lightgbm as lgb import scipy.stats import os
Python Code:
def generate_main_features(df): new_df = df[["row_id", "date", "country", "segment", "num_sold"]].copy() ## one-hot encoding new_df['Rama'] = df.store == 'Rama' for country in ['Finland', 'Norway']: new_df[country] = df.country == country for product in ['Mug', 'Hat']: new_df[product] = df['product'] == product ## datetime features new_df['wd4'] = np.where(df.date.dt.weekday == 4, 1, 0) new_df['wd56'] = np.where(df.date.dt.weekday >= 5, 1, 0) dayofyear = df.date.dt.dayofyear for k in range(1, 3): sink = np.sin(dayofyear / 365 * 2 * math.pi * k) cosk = np.cos(dayofyear / 365 * 2 * math.pi * k) new_df[f'mug_sin{k}'] = sink * new_df['Kaggle Mug'] new_df[f'mug_cos{k}'] = cosk * new_df['Kaggle Mug'] new_df[f'hat_sin{k}'] = sink * new_df['Kaggle Hat'] new_df[f'hat_cos{k}'] = cosk * new_df['Kaggle Hat'] new_df.drop(columns=['mug_sin1'], inplace=True) new_df.drop(columns=['mug_sin2'], inplace=True) # special days new_df = pd.concat([ new_df, pd.DataFrame({f"dec{d}":(df.date.dt.month == 12) & (df.date.dt.day == d) for d in range(24, 32)}), pd.DataFrame({ f"n-dec{d}": (df.date.dt.month == 12) & (df.date.dt.day == d) & (df.country == 'Norway') for d in range(25, 32) }), pd.DataFrame({ f"f-jan{d}": (df.date.dt.month == 1) & (df.date.dt.day == d) & (df.country == 'Finland') for d in range(1, 15) }), pd.DataFrame({ f"n-jan{d}": (df.date.dt.month == 1) & (df.date.dt.day == d) & (df.country == 'Norway') for d in range(1, 10) }), pd.DataFrame({ f"s-jan{d}": (df.date.dt.month == 1) & (df.date.dt.day == d) & (df.country == 'Sweden') for d in range(1, 15) }) ], axis=1) # May and June new_df = pd.concat([ new_df, pd.DataFrame({ f"may{d}": (df.date.dt.month == 5) & (df.date.dt.day == d) for d in list(range(1, 10)) }), pd.DataFrame({ f"may{d}": (df.date.dt.month == 5) & (df.date.dt.day == d) & (df.country == 'Norway') for d in list(range(18, 26)) + [27] }), pd.DataFrame({ f"june{d}": (df.date.dt.month == 6) & (df.date.dt.day == d) & (df.country == 'Sweden') for d in list(range(8, 15)) }) ], axis=1) # Last Wednesday of June wed_june_map = { 2015: pd.Timestamp(('2015-06-24')), 2016: pd.Timestamp(('2016-06-29')), 2017: pd.Timestamp(('2017-06-28')), 2018: pd.Timestamp(('2018-06-27')), 2019: pd.Timestamp(('2019-06-26')) } wed_june_date = df.date.dt.year.map(wed_june_map) new_df = pd.concat([ new_df, pd.DataFrame({ f"wed_june{d}": (df.date - wed_june_date == np.timedelta64(d, "D")) & (df.country != 'Norway') for d in list(range(-4, 5)) }) ], axis=1) # First Sunday of November sun_nov_map = { 2015: pd.Timestamp(('2015-11-1')), 2016: pd.Timestamp(('2016-11-6')), 2017: pd.Timestamp(('2017-11-5')), 2018: pd.Timestamp(('2018-11-4')), 2019: pd.Timestamp(('2019-11-3')) } sun_nov_date = df.date.dt.year.map(sun_nov_map) new_df = pd.concat([ new_df, pd.DataFrame({ f"sun_nov{d}": (df.date - sun_nov_date == np.timedelta64(d, "D")) & (df.country != 'Norway') for d in list(range(0, 9)) }) ], axis=1) # First half of December (Independence Day of Finland, 6th of December) new_df = pd.concat([ new_df, pd.DataFrame({ f"dec{d}": (df.date.dt.month == 12) & (df.date.dt.day == d) & (df.country == 'Finland') for d in list(range(6, 15)) } )], axis=1) # Easter easter_date = df.date.apply(lambda date: pd.Timestamp(easter.easter(date.year))) new_df = pd.concat([ new_df, pd.DataFrame({ f"easter{d}": (df.date - easter_date == np.timedelta64(d, "D")) for d in list(range(-2, 11)) + list(range(40, 48)) + list(range(51, 58)) }), pd.DataFrame({ f"n_easter{d}": (df.date - easter_date == np.timedelta64(d, "D")) & (df.country == 'Norway') for d in list(range(-3, 8)) + list(range(50, 61)) }) ], axis=1) features_list = [ f for f in new_df.columns if f not in ["row_id", "date", "segment", "country", "num_sold", "Rama"] ] return new_df, features_list def get_gdp(row): country = 'GDP_' + row.country return gdp_df.loc[row.date.year, country] def get_cci(row): country = row.country time = f"{row.date.year}-{row.date.month:02d}" if country == 'Norway': country = 'Finland' return cci_df.loc[country[:3].upper(), time].Value def generate_extra_features(df, features_list): df['gdp'] = np.log(df.apply(get_gdp, axis=1)) df['cci'] = df.apply(get_cci, axis=1) features_list_upd = features_list + ["gdp", "cci"] return df, features_list_upd
Final Improvement of Polished Pipeline
1. Let’s take an existing open solution with external data as a baseline
There are already external data in this solution, so improvement shouldn’t be an easy walk ;-):
1) GDP statistics per year/country (“gdp-20152019-finland-norway-and-sweden” dataset);
2) Consumer Confidence Index per year/month/country (Value field of “oecd-consumer-confidence-index” dataset).
And we want to improve the winning kernel by finding new/better external features.
There are no changes in feature engineering from the original notebook by @ambrosm.
So let’s calculate metrics for the baseline solution. First, read train/test data from csv, combine them in one dataframe and generate features:
input_data_path = "/input" df, rama_ratio = read_main_data(input_data_path) # a lot of calendar based features df, baseline_features = generate_main_features(df) print(df.shape) print("Number of features:", len(baseline_features)) df.segment.value_counts() # features from GDP and CCI gdp_df, cci_df = read_additional_data(input_data_path) df, top_solution_features = generate_extra_features(df, baseline_features) print(df.shape) set(top_solution_features) - set(baseline_features)
(32868, 172) Number of features: 166 (32868, 174) {'cci', 'gdp'}
Define model, cross-validation split and apply cross-validation to estimate model accuracy:
model = Ridge(alpha=0.2, tol=0.00001, max_iter=10000) cv = KFold(n_splits=5) top_solution_scores, model_coef = cross_validate(model, df, top_solution_features, rama_ratio, cv=cv) print("Top solution SMAPE by folds:", top_solution_scores) print("Top solution avg SMAPE:", sum(top_solution_scores)/len(top_solution_scores))
Top solution SMAPE by folds: [4.239, 4.159, 4.168, 4.293, 4.077] Top solution avg SMAPE: 4.1872
Now, make the submission file:
submission_path = 'submission_top_solution.csv' make_submission(model, df, top_solution_features, rama_ratio, submission_path)
The submission scores 4.13 on the public leaderboard and 4.55 on the private leaderboard (first place in the competition). This is our baseline.
Can we improve the first place solution even more? Let’s find out!
2. Find relevant external features
To find new features, we’ll use Upgini Feature search and enrichment library for supervised machine learning applications
To initiate a search with the Upgini library, you must define so-called search keys – a set of columns to join external data sources. In this competition, we can use the following keys:
- Column date should be used as SearchKey.DATE.;
- Column country (after conversion to ISO-3166 country code) should be used as SearchKey.COUNTRY.
With this set of search keys, our dataset will be matched with different time-specific features (such as weather data, calendar data, financial data, etc.), considering the country where sales happened. Then relevant selection and ranking will be made. As a result, we’ll add new, only relevant features with additional information about specific dates and countries.
from upgini import SearchKey # here we simply map each country to its ISO-3166 code country_iso_map = { "Finland": "FI", "Norway": "NO", "Sweden": "SE" } df["country_iso"] = df.country.map(country_iso_map) ## define search keys search_keys = { "date": SearchKey.DATE, "country_iso": SearchKey.COUNTRY }
To start the search, we need to initiate a scikit-learn compatible FeaturesEnricher
transformer with appropriate search parameters. After that, we can call the fit method features_enricher
to start the search.
The ratio between Rama and Mart sales is constant, so we’ll use only Mart sales for feature search and model training
%%time from upgini import FeaturesEnricher from upgini.metadata import CVType, RuntimeParameters ## define X_train / y_train, remove Mart condition = (df.segment == "train") & (df.Rama == False) X_train, y_train = df.loc[condition, list(search_keys.keys()) + top_solution_features], df.loc[condition, "num_sold"] ## define Features Enricher features_enricher = FeaturesEnricher( search_keys = search_keys )
CPU times: user 82 ms, sys: 14.7 ms, total: 96.7 ms Wall time: 4.59 s
FeaturesEnricher.fit()
has a flag calculate_metrics
For the quick estimation of quality improvement on cross-validation and eval sets. This step is quite similar to sklearn.model_selection.cross_val_score, so you can pass the exact metric with the scoring
parameter:
- Built-in scoring functions;
- Custom scorer (in this case – scorer based on SMAPE loss).
And we pass the final Ridge model estimator with parameter, estimator
for correct metric calculation, right in search results.
Notice that you should pass X_train as the first argument and y_train as the second argument for, FeaturesEnricher.fit()
, just like in scikit-learn.
The step will take around 3.5 minutes
%%time from sklearn.metrics import make_scorer ## define SMAPE custom scoring function scorer = make_scorer(smape_loss, greater_is_better=False) scorer.__name__ = "SMAPE" ## launch fit features_enricher.fit(X_train, y_train, calculate_metrics = True, scoring = scorer, estimator = model)
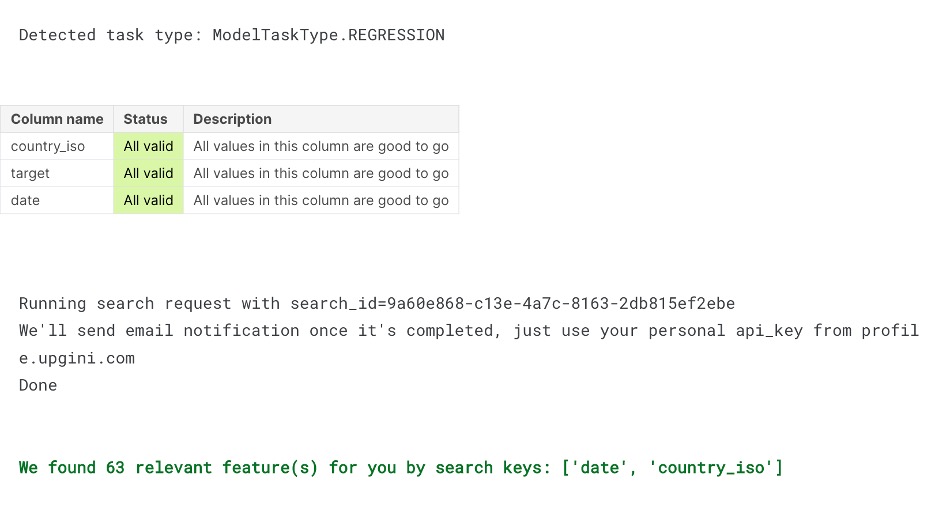
feature_name | shap_value | coverage % | type | |
---|---|---|---|---|
0 | Hat | 0.507071 | 100.000000 | NUMERIC |
1 | Mug | 0.115800 | 100.000000 | NUMERIC |
2 | f_cci_1y_shift_0fa85f6f | 0.088312 | 66.666667 | NUMERIC |
3 | mug_cos1 | 0.046179 | 100.000000 | NUMERIC |
4 | f_pcpiham_wt_531b4347 | 0.029045 | 100.000000 | NUMERIC |
5 | f_cci_6m_shift_653a5999 | 0.022123 | 66.666667 | NUMERIC |
6 | f_year_sin1_16137bbf | 0.019437 | 100.000000 | NUMERIC |
7 | f_weekend_d0c2211b | 0.014037 | 100.000000 | NUMERIC |
8 | f_c2c_fraud_score_5028232e | 0.010249 | 100.000000 | NUMERIC |
9 | hat_sin1 | 0.010188 | 100.000000 | NUMERIC |
10 | f_pcpihac_ix_16f52c8e | 0.009000 | 100.000000 | NUMERIC |
11 | f_credit_default_score_05229fa7 | 0.008933 | 100.000000 | NUMERIC |
12 | f_weather_pca_0_94efd18d | 0.007758 | 100.000000 | NUMERIC |
13 | f_pcpih_wt_10c16d11 | 0.007703 | 100.000000 | NUMERIC |
14 | Norway | 0.007180 | 100.000000 | NUMERIC |
15 | wd56 | 0.006366 | 100.000000 | NUMERIC |
16 | f_pcpihah_ix_260dd0c8 | 0.006058 | 100.000000 | NUMERIC |
17 | f_payment_fraud_score_3cae9c42 | 0.005449 | 100.000000 | NUMERIC |
18 | f_cci_diff_1m_shift_fa99295c | 0.004708 | 66.666667 | NUMERIC |
19 | f_transaction_fraud_union_score_f9bc093c | 0.003007 | 100.000000 | NUMERIC |
20 | f_cci_pca_8_b72fe9f6 | 0.002578 | 100.000000 | NUMERIC |
21 | f_holiday_public_7d_before_e3186344 | 0.002564 | 100.000000 | NUMERIC |
22 | f_pcpih_ix_c2a9fea0 | 0.002472 | 100.000000 | NUMERIC |
23 | f_days_to_election_e6b7c247 | 0.002158 | 100.000000 | NUMERIC |
24 | f_pcpir_pc_cp_a_pt_3c2d5a5f | 0.001977 | 100.000000 | NUMERIC |
25 | f_holiday_national_7d_before_5ca8fb46 | 0.001935 | 100.000000 | NUMERIC |
26 | f_pcpihabt_pc_cp_a_pt_41878919 | 0.001789 | 100.000000 | NUMERIC |
27 | f_pcpihao_pc_cp_a_pt_0c75e62a | 0.001649 | 100.000000 | NUMERIC |
28 | mug_cos2 | 0.001381 | 100.000000 | NUMERIC |
29 | f_cci_1m_shift_8b298796 | 0.001263 | 66.666667 | NUMERIC |
30 | f_days_from_election_e1441706 | 0.001253 | 100.000000 | NUMERIC |
31 | f_pcpi_pc_cp_a_pt_c1d5fdeb | 0.001235 | 100.000000 | NUMERIC |
32 | f_gold_7d_to_1y_1df66550 | 0.001215 | 100.000000 | NUMERIC |
33 | f_holiday_code_7d_before_34f02a4f | 0.001169 | 100.000000 | NUMERIC |
34 | f_pcpifbt_ix_466ad65e | 0.001150 | 100.000000 | NUMERIC |
35 | f_holiday_bank_7d_before_efe82fb3 | 0.001147 | 100.000000 | NUMERIC |
36 | f_pcpiec_ix_5dc7bc66 | 0.001098 | 100.000000 | NUMERIC |
37 | f_cci_diff_6m_shift_52eb4cfe | 0.001090 | 66.666667 | NUMERIC |
38 | f_pcpied_pc_pp_pt_07be13cf | 0.001085 | 100.000000 | NUMERIC |
39 | f_pcpihah_wt_aad13a53 | 0.001043 | 100.000000 | NUMERIC |
40 | f_PCPIEPCH_47b21aec | 0.001013 | 100.000000 | NUMERIC |
41 | f_year_sin2_46dc3051 | 0.000979 | 100.000000 | NUMERIC |
42 | f_pcpio_ix_e60d7742 | 0.000933 | 100.000000 | NUMERIC |
43 | f_year_cos1_cd165f8c | 0.000922 | 100.000000 | NUMERIC |
44 | f_pcpif_pc_cp_a_pt_507a914e | 0.000915 | 100.000000 | NUMERIC |
45 | gdp | 0.000882 | 100.000000 | NUMERIC |
46 | f_pcpir_ix_90fbe7c2 | 0.000867 | 100.000000 | NUMERIC |
47 | Finland | 0.000851 | 100.000000 | NUMERIC |
48 | f_year_sin10_927d1cbc | 0.000821 | 100.000000 | NUMERIC |
49 | f_pcpihat_pc_cp_a_pt_803f3022 | 0.000787 | 100.000000 | NUMERIC |
50 | f_days_to_holiday_5ce1a653 | 0.000755 | 100.000000 | NUMERIC |
51 | f_cpi_pca_6_00674469 | 0.000713 | 100.000000 | NUMERIC |
52 | f_year_cos2_2d8bf75c | 0.000683 | 100.000000 | NUMERIC |
53 | f_week_sin1_a71d22f6 | 0.000673 | 100.000000 | NUMERIC |
54 | f_days_from_holiday_fbad7b66 | 0.000658 | 100.000000 | NUMERIC |
55 | f_BCA_1y_shift_bcc8c904 | 0.000637 | 100.000000 | NUMERIC |
56 | f_us_days_from_election_1658c931 | 0.000611 | 100.000000 | NUMERIC |
57 | f_cpi_pca_2_3c36cd6c | 0.000597 | 100.000000 | NUMERIC |
58 | f_usd_eb23e09d | 0.000591 | 100.000000 | NUMERIC |
59 | f_dow_jones_89547e1d | 0.000578 | 100.000000 | NUMERIC |
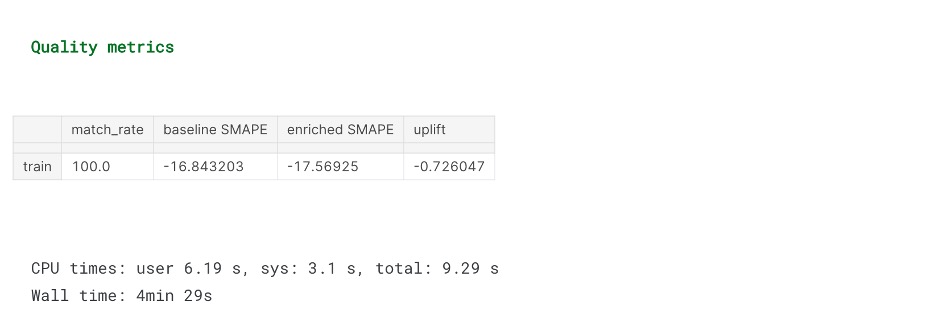
We’ve got 60+ relevant features, which might improve the model’s accuracy. Ranked by SHAP values.
Initial features from the search dataset will also be checked for relevancy, so you don’t need an extra feature selection step.
SMAPE uplift after enrichment with all of the new external features is negative – as it doesn’t make sense to use ALL of them for the linear Ridge model.
Let’s enrich the initial feature space with only the TOP-3 most important features.
Generally, it’s a bad idea to put a lot of features with unknown structure (and possibly high pairwise correlation) into a linear model, like Ridge or Lasso without careful selection and pre-processing.
The step will take around 2 minutes
%%time ## call transform and enrich dataset with TOP-3 features only df_enriched = features_enricher.transform(df, max_features=3, keep_input = True) ## put top-3 new external features names into selected features list enricher_features = [ f for f in features_enricher.get_features_info().feature_name.values if f not in list(search_keys.keys()) + top_solution_features ] best_enricher_features = enricher_features[:3]
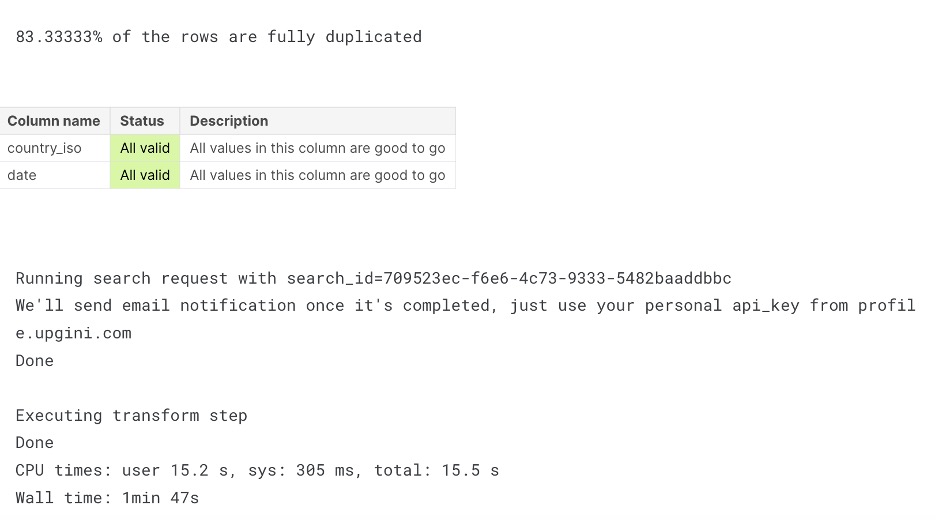
3. Submit and calculate the final leaderboard progress
Let’s estimate model quality and make a submission file:
#same cross-validation split and model estimator as for baseline notebook in #1 Part upgini_scores, model_coef = cross_validate( model, df_enriched, top_solution_features + best_enricher_features, rama_ratio, cv=cv ) print("Top solution SMAPE by folds:", top_solution_scores) print("Upgini SMAPE by folds:", upgini_scores) print("Top solution avg SMAPE:", sum(top_solution_scores)/len(top_solution_scores)) print("Upgini avg SMAPE:", sum(upgini_scores)/len(upgini_scores))
Top solution SMAPE by folds: [4.239, 4.159, 4.168, 4.293, 4.077] Upgini SMAPE by folds: [4.26, 4.129, 4.144, 4.258, 4.051] Top solution avg SMAPE: 4.1872 Upgini avg SMAPE: 4.1684
Make a submission file:
submission_path = 'submission.csv' make_submission( model, df_enriched, top_solution_features + best_enricher_features, rama_ratio, submission_path)
This submission scores 4.095 on public LB and 4.50 on private LB.
Just to remind you – the baseline TOP-1 solution had 4.13 on public LB and 4.55 on private LB (with 2 external data sources already).
We’ve got a consistent improvement both in public and private parts of LB!
Relevant External Features & Data Sources
Leader board accuracy improved from enrichment with 3 new external features:
- f_cci_1y_shift_0fa85f6f – Consumer Confidence Index with 1 year lag. It’s a Consumer Confidence Index value derivative for Finland and Sweden (CCI is unavailable for Norway). CCI as a feature already has been introduced in the baseline notebook, but as a raw CCI index value with scaling on the data prep step.
- f_pcpiham_wt_531b4347 – Consumer Price index for Health group of products & services. In general, Consumer price indexes are index numbers that measure changes in the prices of goods and services purchased or otherwise acquired by households, which households use directly or indirectly to satisfy their needs and wants.
So it has a lot of information about inflation in a specific country and for a specific types of services and goods.
It’s been updated by the Organisation for Economic Cooperation and Development (OECD) every month. - f_cci_6m_shift_653a5999 – Consumer Confidence Index with 6 months lag.
Conclusion
When solving machine learning pipelines task, many data scientists forget that accuracy can be improved by implementing useful external data. Searching for external data can make the accuracy of your machine learning pipeline better, more accurate, and thus make you more money. I showed you how to find useful external data for minutes in this article.
Key takeaways of the article:
- The article contains a description with python code of the way of developing of machine learning model with the best accuracy;
- After reading the article, one can easily improve its ML model with external data;
- The article contains an example of the real syntax of using the upgini python library;
- Moreover, there is a list of real external data contained in upgini database for ML models that helped to improve an existing solution for ML tasks.
I hope you liked my article on machine learning pipelines and if you have any feedback or concerns, share with me in the comments below.
The media shown in this article is not owned by Analytics Vidhya and is used at the Author’s discretion.
Recommend
-
54
README.md
-
8
Building Data Pipelines Learning Number 01- Die Monolith Die Jul 20, 2020 I am in the process of wrapping up a year...
-
12
Netflix's Metaflow: Reproducible machine learning pipelinesFrom training to deployment with Metaflow and Cortex
-
15
Top 10 US Cities To Find a Job in AI and Machine LearningRanking US Cities by Jobs Available per CandidateSourceRegardless of how much experience yo...
-
7
Building Machine Learning Pipelines: Common Pitfalls In recent years, there have been rapid advancements in Machine Learning and this has led to many companies and startups delving into the field without un...
-
9
@erykmlEryk LewinsonData Scientist, Book Author
-
6
Configuration Driven Machine Learning Pipelines Ramandeep Singh and Stefan Krawczyk...
-
6
Introduction Data Scientists have an important role in the modern machine-learning world. Leveraging ML pipelines can save th...
-
3
How to Orchestrate Data for Machine Learning PipelinesHow to Orchestrate Data for Machine Learning PipelinesMay 30th 2023 New Story
-
3
Machine learning for Java developers: Machine learning data pipelines Learn how to build and deploy a machine-learning data model in a Java-based product...
About Joyk
Aggregate valuable and interesting links.
Joyk means Joy of geeK