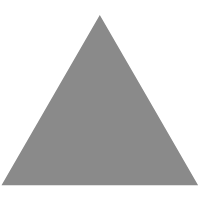
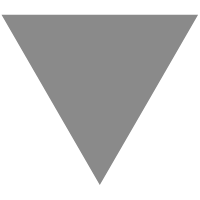
车辆跟随滑模控制的python实现 - 静候佳茵
source link: https://www.cnblogs.com/hitwherznchjy/p/16221206.html
Go to the source link to view the article. You can view the picture content, updated content and better typesetting reading experience. If the link is broken, please click the button below to view the snapshot at that time.
车辆跟随滑模控制的python实现
上一篇文章一个汽车跟踪问题的滑模控制实例,已经从理论上证明了可以使用滑模变结构控制策略来解决汽车跟踪问题。
下面分别采用指数趋近律、等速趋近律、准滑模控制的方法完成车辆跟随问题的仿真
import matplotlib.pyplot as plt
'''
指数趋近律、等速趋近律、准滑模控制的车辆跟随问题仿真, 运行结果以图片形式保存在同目录下。
'''
# q1, q2分别是切换函数ei1, ei2前面的系数
q1, q2 = 2, 1
# lan是指数趋近律前面的系数
lan = 0.5
# 设定期望车间距均为12
l1, l2, l3, l4 = 12, 12, 12, 12
# 设定汽车质量均为1000
m1, m2, m3, m4 = 1000, 1000, 1000, 1000
# 设定动力学模型分子的速度平方项前的系数ci均为0.5(按照模型符号是负的)
c1, c2, c3, c4 = 0.5, 0.5, 0.5, 0.5
# 设定动力学模型分子的常数项系数Fi均为200(按照模型符号是负的)
f1, f2, f3, f4 = 200, 200, 200, 200
# 设定五辆车汽车的位移、速度、加速度
x0, x1, x2, x3, x4 = [100.], [90.], [79.5], [68.5], [57.]
v0, v1, v2, v3, v4 = [20.], [19.], [18.], [17.], [16.]
a1, a2, a3, a4 = [0.], [0.], [0.], [0.]
# 设定趋近律
def reaching_law(m:int , s:float, q2:int, mode='exponential'):
'''
mode: 指数趋近律exponential| 等速趋近律uniform| 准滑模控制quasi_sliding
'''
if mode == 'exponential':
return -m * lan * s / q2
if mode == 'uniform':
epslion = 0.3
if s > 0:
return -m * epslion / q2
if s == 0:
return 0
if s < 0:
return m * epslion / q2
if mode == 'quasi_sliding':
delta, epslion = 0.8, 2.
if s < -delta:
return m * epslion / q2
if s > delta:
return -m * epslion / q2
else:
return -m * epslion * s / (delta * q2)
# 设定第一辆车的加速度(分段函数), 要注意t的长度和a0的长度相等
def get_a0(t:list):
a0 = []
for i in t:
if i < 4:
a0.append(0)
continue
if i >= 4 and i < 7:
a0.append(-0.25*(i-4))
continue
if i >= 7 and i < 10:
a0.append(-0.75)
continue
if i >= 10 and i < 16:
a0.append(0.25*(i-10)-0.75)
continue
if i >= 16 and i < 19:
a0.append(0.75)
continue
if i >= 19 and i < 22:
a0.append(0.25*(19-i)+0.75)
continue
if i >= 22 and i <= 30: # 注意i=30, 所以是取两端, 故为301份
a0.append(0)
return a0
if __name__ == "__main__":
t = [float(i/10) for i in range(301)] # 将30秒划分成301份, [0, 0.1, 0.2, ..., 29.9, 30]
a0 = get_a0(t)
# 四辆车的车间距误差ei1列表
e11 = [x1[0] - x0[0] + l1]
e21 = [x2[0] - x1[0] + l2]
e31 = [x3[0] - x2[0] + l3]
e41 = [x4[0] - x3[0] + l4]
# 四辆车的车间距误差导数ei2的列表
e12 = [v1[0] - v0[0]]
e22 = [v2[0] - v1[0]]
e32 = [v3[0] - v2[0]]
e42 = [v4[0] - v3[0]]
# 四辆车切换函数的列表
s1 = [q1 * e11[0] + q2 * e12[0]]
s2 = [q1 * e21[0] + q2 * e22[0]]
s3 = [q1 * e31[0] + q2 * e32[0]]
s4 = [q1 * e41[0] + q2 * e42[0]]
# 四辆车控制律的列表
u1, u2, u3, u4 = [0], [0], [0], [0]
for i in range(1, 301):
# 最前车0的速度、加速度更新,可以看出更新时用了直线等效, 0.1指的是时间标度(列表t划分的, 也是之后绘图打印的x轴)
v0.append(v0[i-1] + 0.1 * (a0[i] + a0[i - 1]) * 0.5)
x0.append(x0[i-1] + 0.1 * (v0[i] + v0[i - 1]) * 0.5)
# 车1的车间距误差及导数更新
e11.append(x1[i-1] - x0[i-1]+l1)
e12.append(v1[i-1] - v0[i-1])
# 车1的切换函数更新
s1.append(q1 * e11[i] + q2 * e12[i])
# 等效控制
u1equ = c1 * (e12[i] + v0[i]) * (e12[i] + v0[i]) - m1 * q1 * e12[i] / q2 + m1 * a0[i] + f1
# 反馈控制(指数趋近律)
u1n = reaching_law(m1, s1[i], q2) # 默认采用指数趋近律, 下同
# u1n = reaching_law(m1, s1[i], q2, mode='uniform') # 采用等速趋近律
# u1n = reaching_law(m1, s1[i], q2, mode='quasi_sliding') # 采用准滑模控制
# 更新控制律
u1.append(u1equ + u1n)
# 利用控制律更新车1的加速度、速度、位移, 加速度是利用动力学模型得到的
a1.append((-c1 * v1[i-1] * v1[i-1] + u1[i] - f1) / m1)
v1.append(v1[i-1] + 0.1 * (a1[i] + a1[i - 1]) * 0.5)
x1.append(x1[i-1] + 0.1 * (v1[i] + v1[i - 1]) * 0.5)
# 车2、3、4过程同车1
e21.append(x2[i-1] - x1[i-1]+l2)
e22.append(v2[i-1] - v1[i-1])
s2.append(q1 * e21[i] + q2 * e22[i])
u2equ = c2 * (e22[i] + v1[i]) * (e22[i] + v1[i]) - m2 * q1 * e22[i] / q2 + m2 * a1[i] + f2
u2n = reaching_law(m2, s2[i], q2) # 默认采用指数趋近律
# u2n = reaching_law(m2, s2[i], q2, mode='uniform') # 采用等速趋近律
# u2n = reaching_law(m2, s2[i], q2, mode='quasi_sliding') # 采用准滑模控制
u2.append(u2equ + u2n)
a2.append((-c2 * v2[i-1] * v2[i-1] + u2[i] -f2) / m2)
v2.append(v2[i-1] + 0.1 * (a2[i] + a2[i - 1]) * 0.5)
x2.append(x2[i-1] + 0.1 * (v2[i] + v2[i - 1]) * 0.5)
e31.append(x3[i-1] - x2[i-1]+l3)
e32.append(v3[i-1] - v2[i-1])
s3.append(q1 * e31[i] + q2 * e32[i])
u3equ = c3 * (e32[i] + v2[i]) * (e32[i] + v2[i]) - m3 * q1 * e32[i] / q2 + m3 * a2[i] + f3
u3n = reaching_law(m3, s3[i], q2)
# u3n = reaching_law(m3, s3[i], q2, mode='uniform')
# u3n = reaching_law(m3, s3[i], q2, mode='quasi_sliding')
u3.append(u3equ + u3n)
a3.append((-c3 * v3[i-1] * v3[i-1] + u3[i] -f3) / m3)
v3.append(v3[i-1] + 0.1 * (a3[i] + a3[i - 1]) * 0.5)
x3.append(x3[i-1] + 0.1 * (v3[i] + v3[i - 1]) * 0.5)
e41.append(x4[i-1] - x3[i-1]+l4)
e42.append(v4[i-1] - v3[i-1])
s4.append(q1 * e41[i] + q2 * e42[i])
u4equ = c4 * (e42[i] + v3[i]) * (e42[i] + v3[i]) - m4 * q1 * e42[i] / q2 + m4 * a3[i] + f4
u4n = reaching_law(m4, s4[i], q2)
# u4n = reaching_law(m4, s4[i], q2, mode='uniform')
# u4n = reaching_law(m4, s4[i], q2, mode='quasi_sliding')
u4.append(u4equ + u4n)
a4.append((-c4 * v4[i-1] * v4[i-1] + u4[i] -f4) / m4)
v4.append(v4[i-1] + 0.1 * (a4[i] + a4[i - 1]) * 0.5)
x4.append(x4[i-1] + 0.1 * (v4[i] + v4[i - 1]) * 0.5)
# 开始绘图
# 绘制加速度曲线
plt.figure() # 设置画布
plt.plot(t, a0, label='car 0') # :是指绘制点划线
plt.plot(t, a1, label='car 1')
plt.plot(t, a2, label='car 2')
plt.plot(t, a3, label='car 3')
plt.plot(t, a4, label='car 4')
plt.xlabel("Time(s)",fontsize=13)
plt.ylabel("Acceleration(m/s^2)",fontsize=13)
plt.xlim(0, 30)
plt.legend()
plt.savefig('./acceleration.png') # 保存图像
# 绘制速度曲线
plt.clf() # 清空画布,不然会前后图像会重叠
plt.plot(t, v0, ':', label='car 0')
plt.plot(t, v1, ':', label='car 1')
plt.plot(t, v2, ':', label='car 2')
plt.plot(t, v3, ':', label='car 3')
plt.plot(t, v4, ':', label='car 4')
plt.xlabel("Time(s)",fontsize=13)
plt.ylabel("velocity(m/s)",fontsize=13)
plt.xlim(0, 30)
plt.legend()
plt.savefig('./velocity.png') # 保存图像
# 绘制位置曲线
plt.clf()
plt.plot(t, x0, ':', label='car 0')
plt.plot(t, x1, ':', label='car 1')
plt.plot(t, x2, ':', label='car 2')
plt.plot(t, x3, ':', label='car 3')
plt.plot(t, x4, ':', label='car 4')
plt.xlabel("Time(s)",fontsize=13)
plt.ylabel("position(m)",fontsize=13)
plt.xlim(0, 30)
plt.legend()
plt.savefig('./position.png')
# 绘制车间距误差ei1曲线
plt.clf()
plt.plot(t, e11, label='car 1')
plt.plot(t, e21, label='car 2')
plt.plot(t, e31, label='car 3')
plt.plot(t, e41, label='car 4')
plt.xlabel("Time(s)",fontsize=13)
plt.ylabel("space error(m)",fontsize=13)
plt.xlim(0, 30)
plt.legend()
plt.savefig('./space_error.png')
# 绘制车间距误差导数ei2曲线
plt.clf()
plt.plot(t, e12, ':', label='car 1')
plt.plot(t, e22, ':', label='car 2')
plt.plot(t, e32, ':', label='car 3')
plt.plot(t, e42, ':', label='car 4')
plt.xlabel("Time(s)",fontsize=13)
plt.ylabel("space_error_derivative(m)",fontsize=13)
plt.xlim(0, 30)
plt.legend()
plt.savefig('./space_error_derivative.png')
# 绘制切换函数曲线
plt.clf()
plt.plot(t, s1, label='car 1')
plt.plot(t, s2, label='car 2')
plt.plot(t, s3, label='car 3')
plt.plot(t, s4, label='car 4')
plt.xlabel("Time(s)",fontsize=13)
plt.ylabel("Switching Function",fontsize=13)
plt.xlim(0, 30)
plt.legend()
plt.savefig('./Switching_Function.png')
# 绘制控制输入U曲线
plt.clf()
plt.plot(t, u1, label='car 1')
plt.plot(t, u2, label='car 2')
plt.plot(t, u3, label='car 3')
plt.plot(t, u4, label='car 4')
plt.xlabel("Time(s)",fontsize=13)
plt.ylabel("Control Input",fontsize=13)
plt.xlim(0, 30)
plt.legend()
plt.savefig('./Control_Input.png')
Recommend
About Joyk
Aggregate valuable and interesting links.
Joyk means Joy of geeK