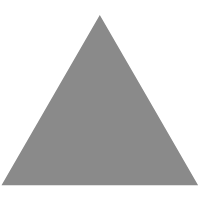
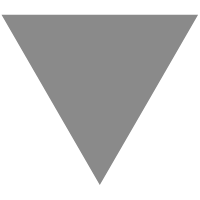
softmax:建立hard-attention到soft-attention的桥梁
source link: https://allenwind.github.io/blog/10256/
Go to the source link to view the article. You can view the picture content, updated content and better typesetting reading experience. If the link is broken, please click the button below to view the snapshot at that time.
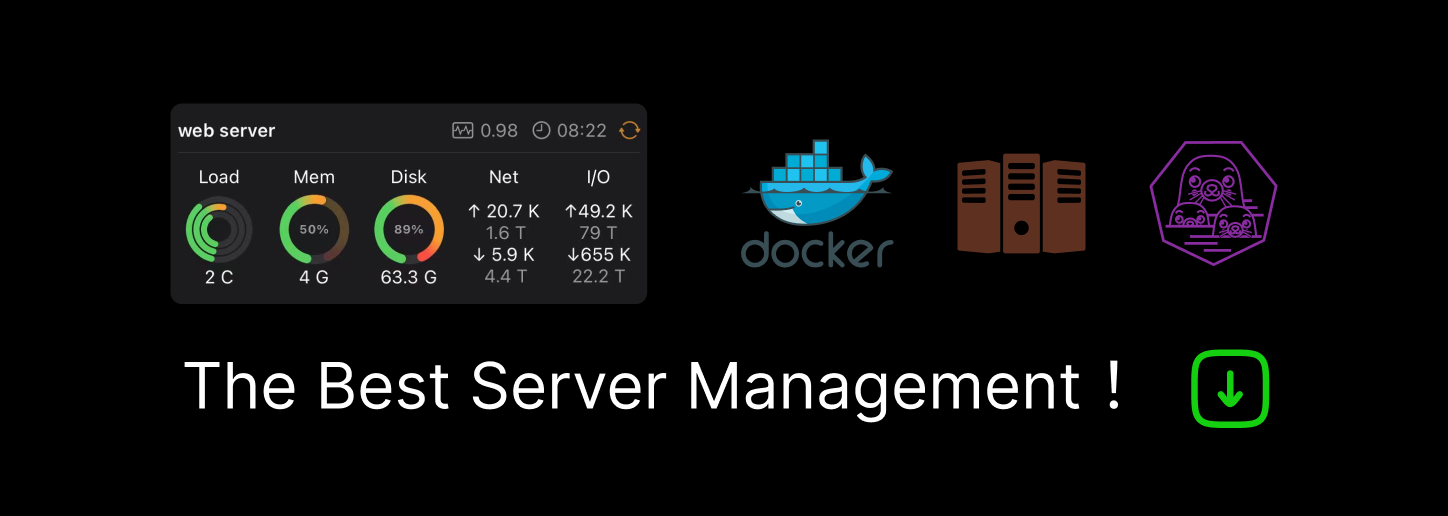
NLP、深度学习、机器学习、Python、Go
softmax:建立hard-attention到soft-attention的桥梁
查询向量 qq 与每个 xixi 计算相关性 αiαi 后,为什么要使用softmax进行归一化?其他归一化方法不行?hard-attention和soft-attention在数学上有什么联系?这里提供一种数学解释。
由于引入softmax函数对注意力评分函数进行归一化并对编码序列进行加权平均容易让人误解为注意力机制就是加权平均,下面会对这个误解展开分析。
注意力机制简述
假设有一向量序列 X=[x1,…,xn]∈Rn×dX=[x1,…,xn]∈Rn×d,有一个和任务相关的向量,称为查询向量 qq,那么注意力机制要做的事情分三步:
- 查询向量 qq 与每个 xixi 计算相关性 αiαi,通过评分函数获得,即αi=s(q,xi)αi=s(q,xi)
- 使用softmax归一化相关性 αiαi,获得注意力分布
- 根据注意力分布计算向量序列的加权平均
这个过程用数学表示如下。首先注意力分布为,
p(z=i|X,q)=softmax(α1,…,αn)=exp(αi)n∑i=1exp(αi)=exp(s(q,xi))n∑i=1exp(s(q,xi))p(z=i|X,q)=softmax(α1,…,αn)=exp(αi)∑i=1nexp(αi)=exp(s(q,xi))∑i=1nexp(s(q,xi))根据注意力分布计算向量序列的加权平均,获得注意力的输出,
Attention(X,q)=n∑i=1p(z=i|X,q)xiAttention(X,q)=∑i=1np(z=i|X,q)xi这就是注意力机制,严格来说说是soft的注意力机制。与之相反的hard注意力机制为,
xi=^Attention(X,q)=xargmaxi=1,⋯,ns(q,xi)xi=A^ttention(X,q)=xargmaxi=1,⋯,ns(q,xi)那么soft的注意力机制为什么要使用softmax多相关性进行归一化?
soft Attention的导出
评分函数s计算查询向量 qq 与每个 xixi 相关性αi=s(q,xi)αi=s(q,xi),另α=[α1,…,αn]α=[α1,…,αn]。Q[i]Q[i]表示向量QQ的第ii个分量。xi=^Attention(X,q)xi=A^ttention(X,q)表示在给定查询向量qq情况下,在向量序列 X=[x1,…,xn]∈Rn×dX=[x1,…,xn]∈Rn×d中找到与其最相关的向量xixi,也就是hard-attention,然后通过一系列推导soft化hard-attention,
xi=^Attention(X,q)=xargmaxi=1,⋯,ns(q,xi)=n∑i=1one-hot(argmaxi=1,⋯,ns(q,xi))[i]×xi=n∑i=1one-hot(argmaxi=1,⋯,nαi)[i]×xi=n∑i=1one-hot(argmaxi=1,⋯,nα−max(α))[i]×xi≈n∑i=1one-hot(argmaxi=1,⋯,neα−logn∑i=1eαi)[i]×xi≈n∑i=1eαin∑i=1eαixi=n∑i=1softmax(α)[i]×xi=Attention(X,q)xi=A^ttention(X,q)=xargmaxi=1,⋯,ns(q,xi)=∑i=1none-hot(argmaxi=1,⋯,ns(q,xi))[i]×xi=∑i=1none-hot(argmaxi=1,⋯,nαi)[i]×xi=∑i=1none-hot(argmaxi=1,⋯,nα−max(α))[i]×xi≈∑i=1none-hot(argmaxi=1,⋯,neα−log∑i=1neαi)[i]×xi≈∑i=1neαi∑i=1neαixi=∑i=1nsoftmax(α)[i]×xi=Attention(X,q)需要说明几点:
- 引入α−max(α)α−max(α)使得最大值为0,使得e0=1e0=1,对应one-hot中的1
- 引入exex是考虑到e0=1,0<ex|x<0<1e0=1,0<ex|x<0<1,更好适配one-hot特点
- max不具有光滑性,被替换为其光滑近似logsumexp
理解好这三点就明白上述推导过程。
注意力机制,本质上是想找与查询向量最相关的隐向量序列,即计算argmax,考虑到输出要对齐,实际上是想找,onehot(argmax),比如的任务是中视野中找苹果,直接依据苹果的特征查找苹果。但是这个求解是无法计算梯度,所以才用onehot(argmax)的光滑版本softmax,以获得良好的梯度特性。也就是说,软性注意力机制中用到加权平均只不过是一种数学上的妥协,同时也带来很多有作用的side effect,但这些side effect不是注意力机制引入加权平均的本质原因。
因此,可以看到softmax是hard-attention到soft-attention的桥梁。
本文从数学角度解释Attention为什么要使用softmax多相关性进行归一化,即加权平均只能说是一种数学技巧,而不是注意力机制的目标。同时也明白,softmax是建立hard-attention到soft-attention的桥梁。
转载请包括本文地址:https://allenwind.github.io/blog/10256
更多文章请参考:https://allenwind.github.io/blog/archives/
Recommend
-
96
之前一直在学习回归和二分类, 今天记录一下多分类的情况。 Softmax 二分类和多分类其实没有多少区别。用的公式仍然是 y=wx + b。 但有一个非常大的区别是他们用的激活函数是不同的。 逻辑回归用的是 sigmoid,这个激活函数的除了给函...
-
40
↑ 点击 蓝字 关注极市平台 作者丨苏剑...
-
16
Hard Forks, Soft Forks, Defaults and Coercion Hard Forks, Soft Forks, Defaults and Coercion 2017 Mar 14 See all posts Hard Forks, Soft Forks, Defaults and Coercion One of the im...
-
9
Artificial Intelligence...
-
5
5 Hard and Soft Skills Every Project Manager Should Have By Tamal Das Published 2 days ago Check out the list of must-have skills ev...
-
7
Sabine Benz February 21, 2022 1 minute read
-
11
Generate image captions In cognitive science, selective attention illustrates how we restrict our attention to particular objects in the surroundings. It helps us focus, so we can tune out irrelevant informat...
-
6
The Problem with Hard and Soft Skills Imagine you are giving awards for individuals that best demonstrate hard and soft technical skills. Who would get the hard skill award? Who would get the soft skill award? W...
-
8
很久之前我维护了一个个人专栏,在其中时常分享一些对行业的看法和理解,但随着时间推移发现了几个让人略感不适的问题:尽管已经有一些关注者,但在无法得到算法青睐的情况下,阅读量和互动情况始终不尽如人意,很多文章发出之后犹如石沉大海;
-
9
Coding Controversies is my new podcast where in each episode I explore a debate in the world of software en...
About Joyk
Aggregate valuable and interesting links.
Joyk means Joy of geeK