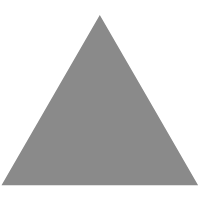
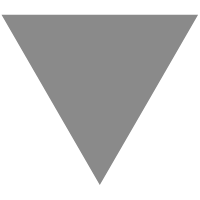
Pandas Tutorial Part #12 – Handling Missing Data
source link: https://thispointer.com/pandas-tutorial-part-12-handling-missing-data/
Go to the source link to view the article. You can view the picture content, updated content and better typesetting reading experience. If the link is broken, please click the button below to view the snapshot at that time.
Pandas Tutorial Part #12 – Handling Missing Data
This tutorial will discuss different ways to handle missing data or NaN values in a Pandas DataFrame, like deleting rows/columns with any NaN value or replacing NaN values with other elements.
Table of Contents
When we load data to the DataFrame, it might contain some missing values. Pandas will automatically replace these missing values with the NaN values. Let’s see how to drop those missing values or replace those missing values with default values.
Let’s create a DataFrame with some NaN / Missing values i.e.
import pandas as pd import numpy as np # List of Tuples empoyees = [('jack', np.NaN, 'Sydney', 5) , ('Riti', 31, 'Delhi', 7) , ('Aadi', 16, 'Karnal', 11) , ('Mark', np.NaN, 'Delhi', np.NaN), ('Veena', 33, 'Delhi', 4) , ('Shaunak', 35, 'Noid', np.NaN), ('Sam', 35, 'Colombo', np.NaN)] # Create a DataFrame object from list of tuples df = pd.DataFrame( empoyees, columns=['Name', 'Age', 'City', 'Experience'], index = ['a', 'b', 'c', 'd', 'e', 'f', 'g']) # Display the DataFrame print(df)
Output
Advertisements
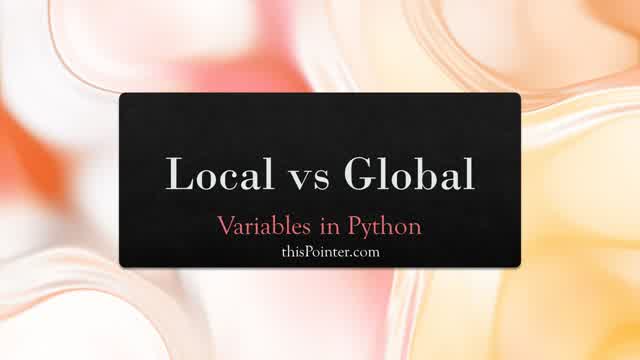
Name Age City Experience a jack NaN Sydney 5.0 b Riti 31.0 Delhi 7.0 c Aadi 16.0 Karnal 11.0 d Mark NaN Delhi NaN e Veena 33.0 Delhi 4.0 f Shaunak 35.0 Noid NaN g Sam 35.0 Colombo NaN
This DataFrame has seven rows and four columns, and it contains few NaN values. Let’s see how to handle NaN values in this DataFrame i.e. either delete rows or columns with NaN values or replace NaN values with some other values.
Drop Missing Values from the DataFrame
In Pandas, the DataFrame provides a function dropna(). We can use this to delete rows or columns based on the NaN or missing values. Let’s understand this with some practical examples.
Drop rows with one or more NaN / Missing values
If we call the dropna() function on the DataFrame object without any argument, it will delete all the rows with one or more NaN / Missing values. For example,
# Delete all rows with one or more NaN values newDf = df.dropna() # Display the new DataFrame print(newDf)
Output
Name Age City Experience b Riti 31.0 Delhi 7.0 c Aadi 16.0 Karnal 11.0 e Veena 33.0 Delhi 4.0
It deleted all the rows with any NaN value. It returned a copy of the modified DataFrame, and if we assign it to the same DataFrame object, it will change the existing DataFrame.
Drop columns with one or more NaN / Missing values
The dropna() function has a parameter axis. If the axis value is 0 (default value is 0), then rows with one or more NaN values get deleted. Whereas, if axis=1, the columns with one or more NaN values get deleted. For example,
# Delete all columns with one or more NaN values newDf = df.dropna(axis=1) # Display the new DataFrame print(newDf)
Output
Name City a jack Sydney b Riti Delhi c Aadi Karnal d Mark Delhi e Veena Delhi f Shaunak Noid g Sam Colombo
It deleted all the columns with any NaN value. It returned a copy of the modified DataFrame, and if we assign it to the same DataFrame object, it will change the existing DataFrame.
Drop Rows / Columns with NaN but with threshold limits
We can also supply the threshold while deleting rows or columns with NaN values. The thesh parameter in the dropna() function means that that row or column will require at least that many non-NaN values to avoid deletion. For example, let’s delete only those columns from the DataFrame which do not have at least 5 non-NaN values. For this, we will pass the thresh value 5,
# Delete columns who dont have at least 5 non NaN values newDf = df.dropna(axis=1, thresh=5) # Display the new DataFrame print(newDf)
Output
Name Age City a jack NaN Sydney b Riti 31.0 Delhi c Aadi 16.0 Karnal d Mark NaN Delhi e Veena 33.0 Delhi f Shaunak 35.0 Noid g Sam 35.0 Colombo
It deleted the column ‘Experience’ because it had only four non-NaN values, whereas the threshold was 5. The column ‘Age’ had NaN values, but it got protected from deletion because it had five non-NaN values under the threshold of 5.
Replacing NaN / Missing values in DataFrame
Instead of deleting, we can also replace NaN or missing values in a DataFrame with some other values. Let’s see how to do that,
Replace NaN values with default values
In Pandas, the DataFrame provides a function fillna() to replace the NaN with default values. The fillna() has a parameter value, which will be used to fill the NaN or missing values. Let’s understand this with some examples,
Contents of out DataFrame object df is,
Name Age City Experience a jack NaN Sydney 5.0 b Riti 31.0 Delhi 7.0 c Aadi 16.0 Karnal 11.0 d Mark NaN Delhi NaN e Veena 33.0 Delhi 4.0 f Shaunak 35.0 Noid NaN g Sam 35.0 Colombo NaN
Replace all NaN values with 0 in this DataFrame,
# Replace all NaN values with zero newDf = df.fillna(value=0) # Display the new DataFrame print(newDf)
Output
Name Age City Experience a jack 0.0 Sydney 5.0 b Riti 31.0 Delhi 7.0 c Aadi 16.0 Karnal 11.0 d Mark 0.0 Delhi 0.0 e Veena 33.0 Delhi 4.0 f Shaunak 35.0 Noid 0.0 g Sam 35.0 Colombo 0.0
It replaced all the NaN values 0s in the DataFrame. It returned a copy of the modified DataFrame, and if we assign it to the same DataFrame object, it will modify the existing DataFrame.
Here, we replaced all the NaN values with a specific value, but what if we want to replace the NaN values with some other values like the mean of values in that column. Let’s see how to do that.
Replace NaN values in a column with the mean
Select the column by its name using the subscript operator i.e. df[column_name] and call the fillna() function and pass the mean of column values. It will replace all the NaN values in that column with the mean. For example,
# Replace NaN values in column with the mean of column values df['Experience'] = df['Experience'].fillna(df['Experience'].mean()) # Display the new DataFrame print(df)
Output
Name Age City Experience a jack NaN Sydney 5.00 b Riti 31.0 Delhi 7.00 c Aadi 16.0 Karnal 11.00 d Mark NaN Delhi 6.75 e Veena 33.0 Delhi 4.00 f Shaunak 35.0 Noid 6.75 g Sam 35.0 Colombo 6.75
Here, we replaced all the NaN values in the column ‘Experience’ with the mean of values in that column.
Summary:
We learned how to handle NaN values in the DataFrame i.e., delete rows or columns with NaN values. Then we also looked at the ways to replace NaN values with some specific values.
Pandas Tutorials -Learn Data Analysis with Python
Are you looking to make a career in Data Science with Python?
Data Science is the future, and the future is here now. Data Scientists are now the most sought-after professionals today. To become a good Data Scientist or to make a career switch in Data Science one must possess the right skill set. We have curated a list of Best Professional Certificate in Data Science with Python. These courses will teach you the programming tools for Data Science like Pandas, NumPy, Matplotlib, Seaborn and how to use these libraries to implement Machine learning models.
Checkout the Detailed Review of Best Professional Certificate in Data Science with Python.
Remember, Data Science requires a lot of patience, persistence, and practice. So, start learning today.
Recommend
About Joyk
Aggregate valuable and interesting links.
Joyk means Joy of geeK