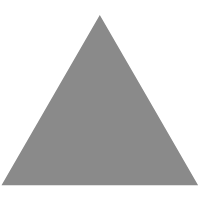
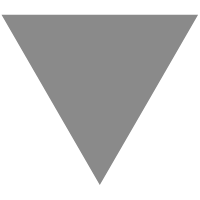
论文解读:Variational Graph Auto-Encoders
source link: https://weisenhui.top/posts/2838.html
Go to the source link to view the article. You can view the picture content, updated content and better typesetting reading experience. If the link is broken, please click the button below to view the snapshot at that time.
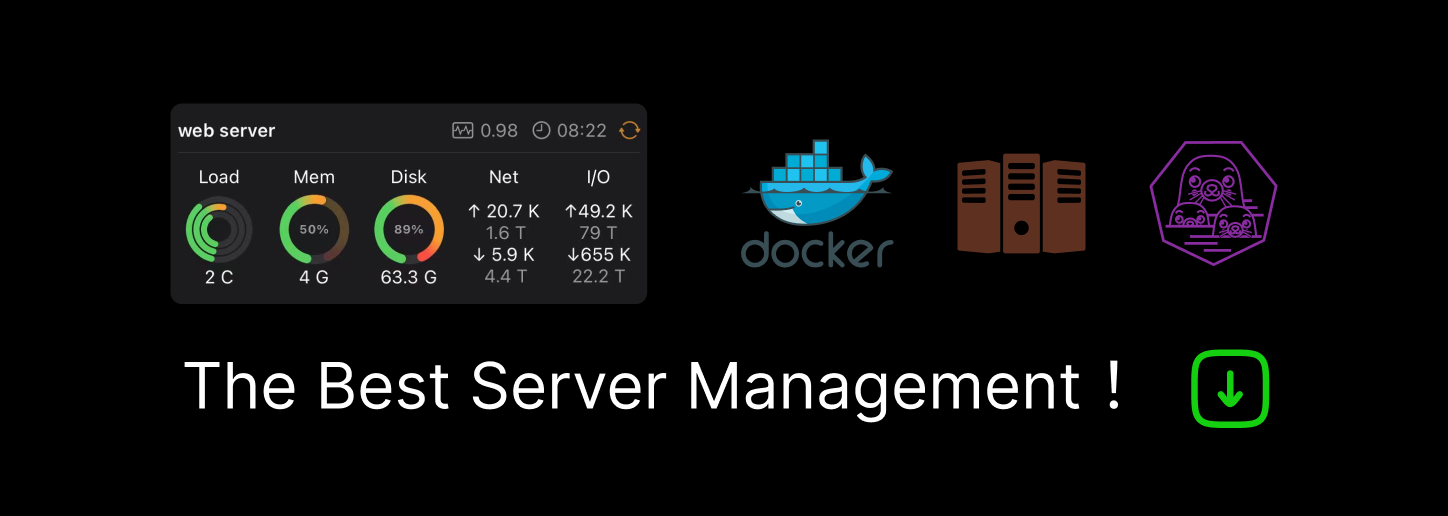
- 论文题目:Variational Graph Auto-Encoders
- 论文作者:Thomas N. Kipf, Max Welling
- 论文组织:University of Amsterdam
- 论文会议:NIPS Workshop 2016
- 论文地址:https://arxiv.org/pdf/1611.07308.pdf
- 论文代码:https://github.com/zfjsail/gae-pytorch/blob/master/gae/model.py
Kipf 与 Welling 16 年发表的「Variational Graph Auto-Encoders」提出了基于图的(变分)自编码器 Variational Graph Auto-Encoder(VGAE),自此开始,图自编码器凭借其简洁的 encoder-decoder 结构和高效的 encode 能力,在很多领域都派上了用场。
Thomas N. Kipf大佬不仅是这篇VGAE的作者,而且还是大名鼎鼎模型GCN的作者。二作是CIFAR10实验室的Max Welling,他是GCN和VAE的作者之一,Kipf是Max Welling的博士生。
图神经网络背景知识
假设我们的数据集是Cora数据集 每个样本点都是一篇科学论文,所有样本点被分为8个类别,现在想训练一个模型预测每个样本点对应的类别。
样本 特征x 标签y
31336: x=(0,1,0,0,0...0), y=Neural_Networks
10611: x=(0,0,1,0,1...0), y=Rule_Learning
...
12135: x=(0,0,0,0,0...0), y= Reinforcement_Learning
- 方法1:直接对Py|x建模(传统监督学习),模型性能一般
- 方法2:先基于图结构的图神经网络模型(如GCN)提取更好的表征x−>z,再进行下游任务建模P(y|z),模型性能较好(这种方法就是图神经网络)
图神经网络的模型Pipeline:
所以图神经网络模型的目的是学习meaningful latent embeddings z
类型1:监督学习或半监督学习通过x,y来学习表征z
类型2:当图上没有标签y时,通过无监督学习的方式来学习z,即只通过x学习z
常见的基于图的无监督学习方法有:
- GAE:图自编码器 Graph Auto-Encoder
- VGAE:变分图自编码器 Variational Graph Auto-Encoder
一、GAE 图自编码器
简单回顾下经典的Auto-Encoder模型:
GAE是GCN在Auto-Encoders的应用,非常容易理解,隐变量Z就是图上的N个节点经过GCN后的N*F维特征,Encoder就是两层GCN, Decoder就是向量点积。可以将隐变量Z理解为某种意义上图的节点的相似度,通过向量点积得到的两个图节点的相似度越大,则两个节点之间存在边的概率越大
输入X:N×D,邻接矩阵A:N×N,隐向量Z:N×F,重构出来的邻接矩阵A′:N×N
- GAE使用
GCN
作为encoder来得到节点的latent representations- Z=GCN(X,A)=ˆAReLU(ˆAXW0)W1, 其中ˆA=D−12AD−12,注意VGAE论文中的A已经加上了I
- 原论文的描述:We introduce an adjacency matrix A of G weassumediagonalelementssetto1,i.e.everynodeisconnectedtoitself and its degree matrix D.
- GAE使用
inner-product
作为decoder来reconstruct原始的图- A′=σ(ZZT)=sigmoid(ZZT)
GAE是如何训练,它的loss函数是什么?
一个好的Z,就应该使重构出的邻接矩阵与原始的邻接矩阵尽可能的相似。损失函数要能衡量生成图和原始图之间差异:
Loss(A,A′)=−1N∑ylogy′+(1−y)log(1−y′)
上式中,y代表领接矩阵A(已加上I)中某个元素的值(0或1),y′代表重构的领接矩阵A′中相应元素的值(0到1之间)
下图来自综述《A Comprehensive Survey on Graph Neural
Networks》https://arxiv.org/pdf/1901.00596.pdf
二、VGAE 图变分自编码器
简单回顾下经典的VAE模型:
- VAE训练阶段:
- VAE预测阶段:
VGAE是GCN在Variational Graph Auto-Encoders VAE的应用。Encoder用两个两层GCN分别得到N个均值和标准差,这两个GCN会共享第一层的参数,从而得到N个正态分布。Decoder仍然是向量点积。
VGAE是如何训练,它的loss函数是什么?
另外为了使VGAE能进行梯度反向传播,论文采用了和VAE相同的重采样技巧
We perform full-batch gradient descent and make use of the reparameterization trick for training.
三、实验结果
任务:链路预测
四、参考资料
[1] VGAE(Variational graph auto-encoders)论文详解
[2] 【GNN】VGAE:利用变分自编码器完成图重构
[3] 【GNN五大类 VGAE】(变分图自编码器):Variational Graph Auto-Encoders
[4] Tutorial on Variational Graph Auto-Encoders
Recommend
-
43
Recently, I’ve been trying to learn more about electronics and embedded development. Maybe I’m just tired of operating purely in the virtual, but there’s something cool about being able to physically put together a circui...
-
14
README.md PreSumm This code is for EMNLP 2019 paper Text Summarization with Pretrained Enc...
-
51
An introduction to Variational Auto Encoders (VAEs) Understanding Variational Autoencoders (VAEs) from theory to practice using PyTorch
-
13
论文标题:GraphMAE: Self-Supervised Masked Graph Autoencoders论文作者:Zhenyu Hou, Xiao Liu, Yukuo Cen, Yuxiao Dong, Hongxia Yang, Chunjie Wang, Jie Tang论文来源:2022, KDD论文地址:
-
12
论文标题:Towards Explanation for Unsupervised Graph-Level Representation Learning论文作者:Qinghua Zheng, Jihong Wang, Minnan Luo, Yaoliang Yu, Jundong Li, Lina Yao, Xiaojun Chang论文来源:2022, arXiv论文地址:
-
8
论文标题:Learning Graph Augmentations to Learn Graph Representations论文作者:Kaveh Hassani, Amir Hosein Khasahmadi论文来源:2022, arXiv论文地址:download
-
6
论文标题:Efficient Graph Convolution for Joint Node RepresentationLearning and Clustering论文作者:Chakib Fettal, Lazhar Labiod,Mohamed Nadif论文来源:2021, WSDM论文地址:
-
8
论文标题:Interpretable and Generalizable Graph Learning via Stochastic Attention Mechanism论文作者:Siqi Miao, Mia Liu, Pan Li论文来源:2022,ICML论文地址:d...
-
5
论文标题:DropEdge: Towards Deep Graph Convolutional Networks on Node Classification论文作者:Yu Rong, Wenbing Huang, Tingyang Xu, Junzhou Huang论文来源:2020, ICLR论文地址:
-
7
论文标题:Graph U-Nets论文作者:Hongyang Gao, Shuiwang Ji论文来源:2019,ICML论文地址:download 论文代码:
About Joyk
Aggregate valuable and interesting links.
Joyk means Joy of geeK