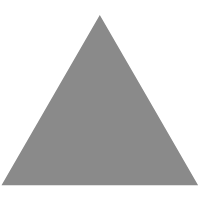
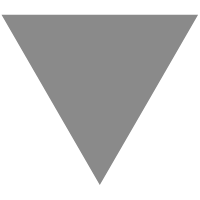
Bias persists in face detection systems from Amazon, Microsoft, and Google
source link: https://venturebeat.com/2021/09/03/bias-persists-in-face-detection-systems-from-amazon-microsoft-and-google/
Go to the source link to view the article. You can view the picture content, updated content and better typesetting reading experience. If the link is broken, please click the button below to view the snapshot at that time.
Taking data to the cloud
With the explosion of data, every application is now a data application. Learn why this has hundreds of software teams building their applications in the cloud across multiple use cases and industries.
Register hereThe Transform Technology Summits start October 13th with Low-Code/No Code: Enabling Enterprise Agility. Register now!
Commercial face-analyzing systems have been critiqued by scholars and activists alike over the past decade, if not longer. A paper last fall by University of Colorado, Boulder researchers showed that facial recognition software from Amazon, Clarifai, Microsoft, and others was 95% accurate for cisgender men but often misidentified trans people. Furthermore, independent benchmarks of vendors’ systems by the Gender Shades project and others have revealed that facial recognition technologies are susceptible to a range of racial, ethnic, and gender biases.
Companies say they’re working to fix the biases in their facial analysis systems, and some have claimed early success. But a study by researchers at the University of Maryland finds that face detection services from Amazon, Microsoft, and Google remain flawed in significant, easily detectable ways. All three are more likely to fail with older, darker-skinned people compared with their younger, whiter counterparts. Moreover, the study reveals that facial detection systems tend to favor “feminine-presenting” people while discriminating against certain physical appearances.
Face detection
Face detection shouldn’t be confused with facial recognition, which matches a detected face against a database of faces. Face detection is a part of facial recognition, but rather than performing matching, it only identifies the presence and location of faces in images and videos.
Recent digital cameras, security cameras, and smartphones use face detection for autofocus. And face detection has gained interest among marketers, which are developing systems that spot faces as they walk by ad displays.
In the University of Maryland preprint study, which was conducted in mid-May, the coauthors tested the robustness of face detection services offered by Amazon, Microsoft, and Google. Using over 5 million images culled from four datasets — two of which were open-sourced by Google and Facebook — they analyzed the effect of artificially added artifacts like blur, noise, and “weather” (e.g., frost and snow) on the face detection services’ performance.
The researchers found that the artifacts disparately impacted people represented in the datasets, particularly along major age, race, ethnic, and gender lines. For example, Amazon’s face detection API, offered through Amazon Web Services (AWS), was 145% more likely to make a face detection error for the oldest people when artifacts were added to their photos. People with traditionally feminine facial features had lower detection errors than “masculine-presenting” people, the researchers claim. And the overall error rate for lighter and darker skin types was 8.5% and 9.7%, respectively — a 15% increase for the darker skin type.
“We see that in every identity, except for 45-to-65-year-old and feminine [people], the darker skin type has statistically significant higher error rates,” the coauthors wrote. “This difference is particularly stark in 19-to-45 year old, masculine subjects. We see a 35% increase in errors for the darker skin type subjects in this identity compared to those with lighter skin types … For every 20 errors on a light-skinned, masculine-presenting individual between 18 and 45, there are 27 errors for dark-skinned individuals of the same category.”
Dim lighting in particular worsened the detection error rate for some demographics. While the odds ratio between dark- and light-skinned people decreased with dimmer photos, it increased between age groups and for people not identified in the datasets as male or female (e.g., nonbinary people). For example, the face detection services were 1.03 times as likely to fail to detect someone with darker skin in a dim environment compared with 1.09 times as likely in a bright environment. And for a person between the ages of 45 and 64 in a well-lit photo, the systems were 1.150 times as likely to register an error than with a 19-to-45-year-old — a ratio that dropped to 1.078 in poorly-lit photos.
In a drill-down analysis of AWS’ API, the coauthors say that the service misgendered 21.6% of the people in photos with added artifacts versus 9.1% of people in “clean” photos. AWS’ age estimation, meanwhile, averaged 8.3 years away from the actual age of the person for “corrupted” photos compared with 5.9 years away for uncorrupted data.
“We found that older individuals, masculine presenting individuals, those with darker skin types, or in photos with dim ambient light all have higher errors ranging from 20-60% … Gender estimation is more than twice as bad on corrupted images as it is on clean images; age estimation is 40% worse on corrupted images,” the researchers wrote.
Bias in data
While the researchers’ work doesn’t explore the potential causes of biases in Amazon’s, Microsoft’s, and Google’s face detection services, experts attribute many of errors in facial analysis systems to flaws in the datasets used to train the algorithms. A study conducted by researchers at the University of Virginia found that two prominent research-image collections displayed gender bias in their depiction of sports and other activities, for example showing images of shopping linked to women while associating things like coaching with men. Another computer vision corpus, 80 Million Tiny Images, was found to have a range of racist, sexist, and otherwise offensive annotations, such as nearly 2,000 images labeled with the N-word, and labels like “rape suspect” and “child molester.”
The University of Maryland researchers say that their work points to the need for greater consideration of the implications of biased AI systems deployed into production. Recent history is filled with examples of the consequences, like virtual backgrounds and automatic photo-cropping tools that disfavor darker-skinned people. Back in 2015, a software engineer pointed out that the image recognition algorithms in Google Photos were labeling his Black friends as “gorillas.” And the nonprofit AlgorithmWatch has shown that Google’s Cloud Vision API at once time automatically labeled thermometers held by a Black person as “guns” while labeling thermometers held by a light-skinned person as “electronic devices.”
Amazon, Microsoft, and Google in 2019 largely discontinued the sale of facial recognition services but have so far declined to impose a moratorium on access to facial detection technologies and related products. We’ve reached out to the companies for comment on the study’s results and will update this piece once we receive a response.
“[Our work] adds to the burgeoning literature supporting the necessity of explicitly considering bias in machine learning systems with morally laden downstream uses,” the researchers wrote.
VentureBeat
VentureBeat's mission is to be a digital town square for technical decision-makers to gain knowledge about transformative technology and transact.
Our site delivers essential information on data technologies and strategies to guide you as you lead your organizations. We invite you to become a member of our community, to access:
- up-to-date information on the subjects of interest to you
- our newsletters
- gated thought-leader content and discounted access to our prized events, such as Transform 2021: Learn More
- networking features, and more
Recommend
About Joyk
Aggregate valuable and interesting links.
Joyk means Joy of geeK