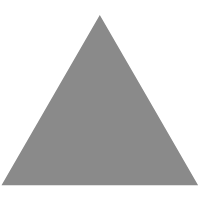
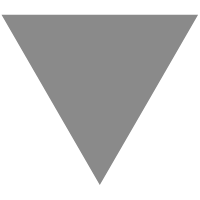
【TensorFlow1】session,变量
source link: https://www.guofei.site/2017/12/01/tf1.html
Go to the source link to view the article. You can view the picture content, updated content and better typesetting reading experience. If the link is broken, please click the button below to view the snapshot at that time.
【TensorFlow1】session,变量
2017年12月01日Author: Guofei
文章归类: 2-3-神经网络与TF ,文章编号: 281
版权声明:本文作者是郭飞。转载随意,但需要标明原文链接,并通知本人
原文链接:https://www.guofei.site/2017/12/01/tf1.html
1. Session
1.1 用法1
import tensorflow as tf
a=tf.constant([10.1,2.0],name='a')
b=tf.constant([1.0,2.1],name='b')
sess.run(a+b)
sess.close() # 关闭 session, 释放资源
1.2 用法2
with tf.Session() as sess:
sess.run(a+b)
# 无需调用sess.close(),而是运行完毕后自动释放资源
1.3 用法3
sess=tf.Session()
result=a+b
with sess.as_default():
print(result.eval())
sess=tf.Session()
result.eval(session=sess)
1.4 InteractiveSession
注册为默认Session,以后的运算会默认在这个session中运行
sess=tf.InteractiveSession()
2. config
config=tf.ConfigProto(allow_soft_placement=True, # 优先使用GPU,GPU不支持的时候换成CPU,而不是报错
log_device_placement=True) #记录节点分配日志,生产环境下可以设定为False以减小日志量
sess1=tf.InteractiveSession(config=config)
sess2=tf.Session(config=config)
3. 三大常用数据结构
- constant
- Variable (记得initialize)
- placeholder (记得在sess.run中,用feed_dict传入数据)
3.1 constant
tf.random_normal(shape=(3,2),mean=0.0,stddev=1.0,dtype=tf.float32) # 正态分布
b=tf.truncated_normal(shape=(3,2),mean=0.0,stddev=1.0,dtype=tf.float32) # 正态分布,如果离差超过2标准差,则重新随机
tf.random_uniform(shape=(3,2),minval=0.0,maxval=1.0,dtype=tf.float32) # 均匀分布
tf.random_gamma(shape=(3,2),alpha=1,beta=2,dtype=tf.float32) # Gamma分布
tf.random_shuffle(value, seed=None, name=None)
tf.random_crop(value, size, seed=None, name=None)
tf.multinomial(logits, num_samples, seed=None, name=None)
tf.random_gamma(shape, alpha, beta=None, dtype=tf.float32, seed=None, name=None)
tf.set_random_seed(seed)
tf.zeros(shape=[1,2])
tf.ones
tf.fill(dims=(2,3),value=9)#生成一个2*3矩阵,并用9填充
a=tf.constant([1,2,4])
tf.zeros_like(a, dtype=tf.float32, name=None)
tf.ones_like(a, dtype=tf.float32, name=None)
tf.linspace(start=0.0,stop=1.0,num=5) # 含头含尾,num个
3.2 Variable
import math
# 参数初始化
# 均值0,方差1的正态随机数:
weights=tf.Variable(tf.truncated_normal([3,2],stddev=1),name='weights')
#正态随机数除以神经元的个数
weights=tf.Variable(tf.truncated_normal([500,25],stddev=1/math.sqrt(500)),name='weights')
另一种写法,区别是,如果变量存在,则会报错。用 tf.Variable 则是覆盖:
W1 = tf.get_variable(name='W1',shape=[4, 4, 3, 8],initializer=tf.contrib.layers.xavier_initializer(seed = 0))
# 常用initializer:
tf.constant_initializer(value=0, dtype=tf.float32)
tf.random_normal_initializer(mean=0.0, stddev=1.0, seed=None, dtype=tf.float32)
tf.truncated_normal_initializer(mean=0.0, stddev=1.0, seed=None, dtype=tf.float32)
tf.random_uniform_initializer(minval=0, maxval=None, seed=None, dtype=tf.float32)
tf.uniform_unit_scaling_initializer(factor=1.0, seed=None, dtype=tf.float32)
tf.zeros_initializer(shape, dtype=tf.float32, partition_info=None)
tf.ones_initializer(dtype=tf.float32, partition_info=None)
tf.orthogonal_initializer(gain=1.0, dtype=tf.float32, seed=None)
Variable 的初始化
tf.Variable数据类型一定要 initialize 之后才可以参与sess.run()
(对比,constant和placeholder不需要初始化便可以放入到sess.run())
- w.initializer初始化单个Variable
sess.run(w2.initializer) sess.run(w3.initializer) sess.run(w2+w3)
- 也可以用global_variables_initializer()初始化所有变量
init=tf.global_variables_initializer() sess.run(init) sess.run(w4+w5)
- global_variables_initializer的另一种写法
tf.global_variables_initializer().run(session=sess) sess.run(w4+w5)
state=tf.Variable(0,name='counter')
one=tf.constant(1)
new_value=tf.add(state,one)
update=tf.assign(state,new_value)
sess.run(tf.global_variables_initializer())
for i in range(3):
sess.run(update)
print(sess.run(state))
3.3 placeholder占位
定义一个位置,这个位置的数据在运行时才指定
from scipy.stats import norm
rv=norm()
x=tf.placeholder(shape=(None,6),dtype=tf.float32)
m=tf.random_normal(shape=(6,2))
y=tf.matmul(x,m)
sess.run(tf.global_variables_initializer())
sess.run(y,feed_dict={x:rv.rvs(size=(3,6))}) #使用 feed_dict 指定placeholder
参考文献
《Matlab神经网络原理与实例精解》陈明,清华大学出版社
《神经网络43个案例》王小川,北京航空航天大学出版社
《人工神经网络原理》马锐,机械工业出版社
白话深度学习与TensorFlow,高扬,机械工业出版社
《TensorFlow实战Google深度学习框架》,郑泽宇,中国工信出版集团
您的支持将鼓励我继续创作!
Recommend
About Joyk
Aggregate valuable and interesting links.
Joyk means Joy of geeK