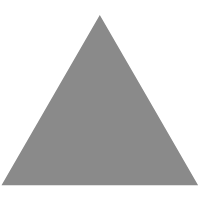
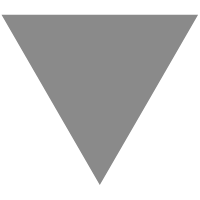
Predictive vs. generative design methods for design & architecture
source link: https://uxdesign.cc/predictive-v-generative-design-methods-for-design-architecture-f955f740444a
Go to the source link to view the article. You can view the picture content, updated content and better typesetting reading experience. If the link is broken, please click the button below to view the snapshot at that time.
Predictive vs. generative design methods for design & architecture
5 aspects for everyone to clarify expectations for digital tools that will shape the world of tomorrow, now.
We have a crisis. There are less than 10 years left to meet 2030 goals, and according to the AIA 2030 report for 2019 only 15% of new projects reported are meeting those goals. There is a long way to go with limited resources. Fortunately, automation and computational design are catapulting designers, architects, and engineers into the exponential realm of digital modeling of complex systems for buildings, cities, and the environment. In the last 10 years alone innovative technologies for performance-based design processes have advanced significantly with multi-objective simulations, cloud computing, and machine learning. The result is our current era proposing a new aesthetics and philosophy revolving around parametricism. Patrick Schumacher of Zaha Hadid Architects has fiercely pioneered this theory of parametric process as an aesthetic driver for avant-garde architecture. But what does this mean for the everyday designer, engineer, and architect?
Performance-based data-driven design tools push parametricism beyond aesthetics to evaluate the performance of a design in the near-real-time of its digital creation. These tools have many variations but can be categorized into two main groups that we’ll call predictive and generative design tools. As the AEC culture explores the various uses for each type, it is important to know how to examine them. Vetting a new digital process is possible by understanding what and how something is measured, what is assumed and why it matters, how to maintain quality and control, and ultimately expecting the appropriate return for the effort.
Knowing these key aspects of predictive and generative design processes will equip the everyday professional with a foundation, regardless of position or expertise, to understand what each tool can offer and which is better for them. Here are 5 key aspects of what to meaningfully expect from advanced computational modeling for predictive and generative design tools, and how to frame the argument for predictive v. generative design results.
5 aspects of predictive and generative design methods to help clarify expectations.
- Quantitative v. Qualitative Measures (you get out what you put in)
- Automation v. Machine Learning (different endgames)
- Trust v. Usefulness (bias in our assumptions)
- Quality v. Control (who decides?)
- Ford v. Ferrari (persistence is futile)
Note: Feel free to scan through and read the bold sections to get the gist of everything.
Let’s set the stage.
I’m going to ignore the development of quantum computing here for simplicity and for the fact that there is only a handful in operation currently working, and none of them (that I know of) are analyzing buildings.
Gone are the days of simply drawing with the pc in software like AutoCAD. Here to stay are the complex building information models (BIM) in an era of computer-aided “everything”. It’s 2020, and I’ve just attended the latest conference for the Association for Computer-Aided Design in Architecture (ACADIA), a group of architects, designers, and artists all exploring the digital realm to find new ways to design and make things. It was particularly interesting this year as the whole event was a virtual conference presenting the latest projects and research that explore advanced computational processes for design. The irony did not escape me that we’re talking about advanced computational methods leveraging advanced computational infrastructure. The power of readily available digital hardware, international digital infrastructure, and cloud-based programs allowed video streaming discussions around the topic of other advanced digital tools.
Historically, faster computing power has offered promises of finally developing quantitative methods to measure qualitative outcomes, but with very few relevant developments, especially for the majority of architectural design. The closest example we have is computational heuristics, which is to say a computational method that aids discovery through trial and error of different parameters. However, just because that is how humans discover new insights, doesn’t mean the computer is going to discover anything better using our method. Quicker maybe (hopefully), but not necessarily better.

Let’s clarify a few things about our design methods before getting into the bits and pieces.
Data-driven design is an umbrella term describing a method of using collected information as input data to analyze and provide a more objective insight into what is happening that drives the design motive. Predictive and generative design are two examples of the spectrum of data-driven design methods using computational heuristics applied at various stages throughout the design processes. Each method has recently gained a lot of momentum (and money) for architectural design, so it’s worth taking a moment to clarify a few things.
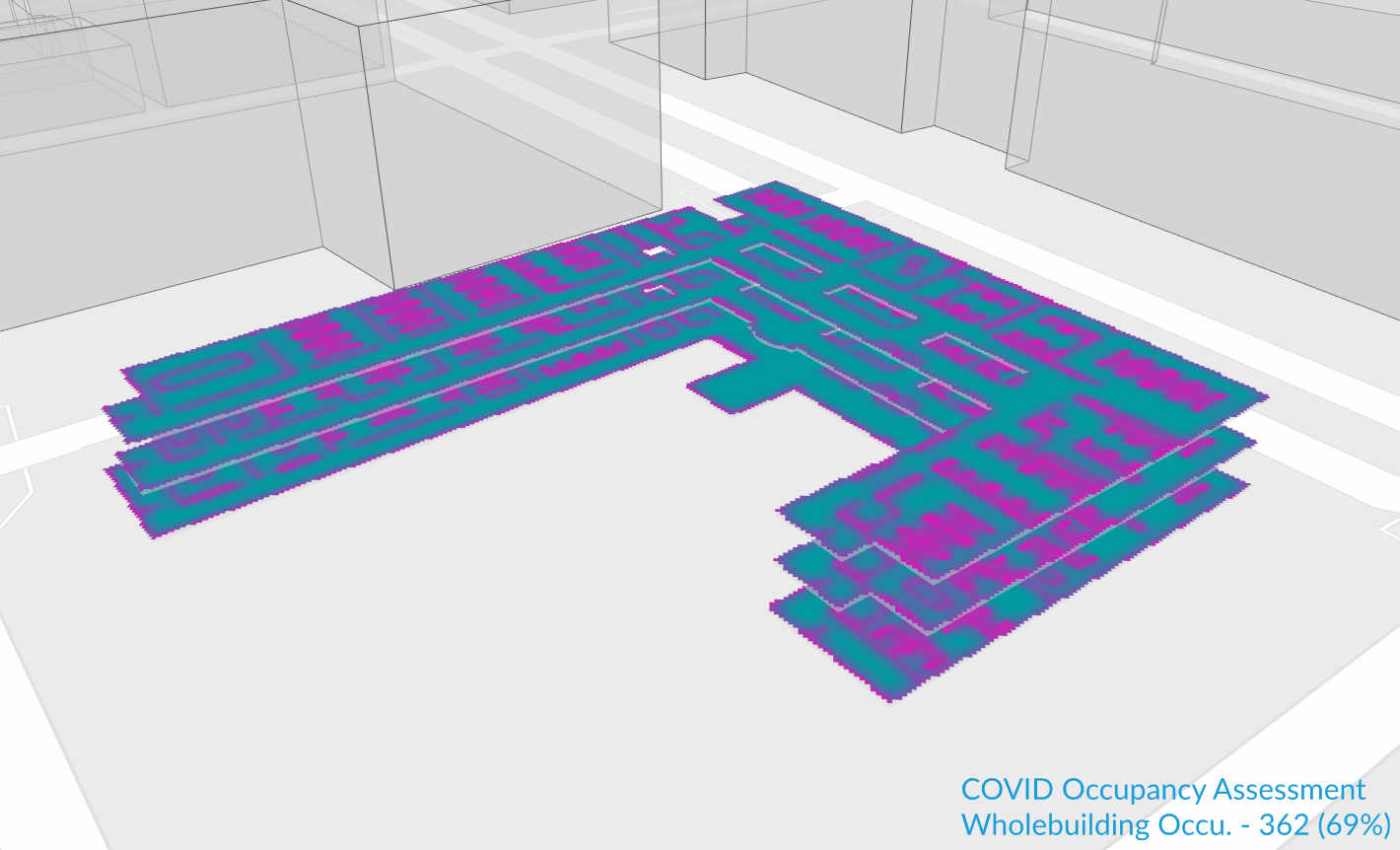
Predictive design offers a critically different approach to achieve optimal design performance compared to generative design. On one end of the spectrum, predictive analysis tends to be in tandem with the designer throughout the process allowing the human to determine the final shape or set of parameters. It essentially uses evidentiary information rather than a speculative rationalization to determine the value of a solution. In other words, it tests a set of “functions” of a given “form” to see how it performs. The responsibility of determining a good or bad performance result is clearly on the user, not the computer, to determine if that design is the best fit to move forward.
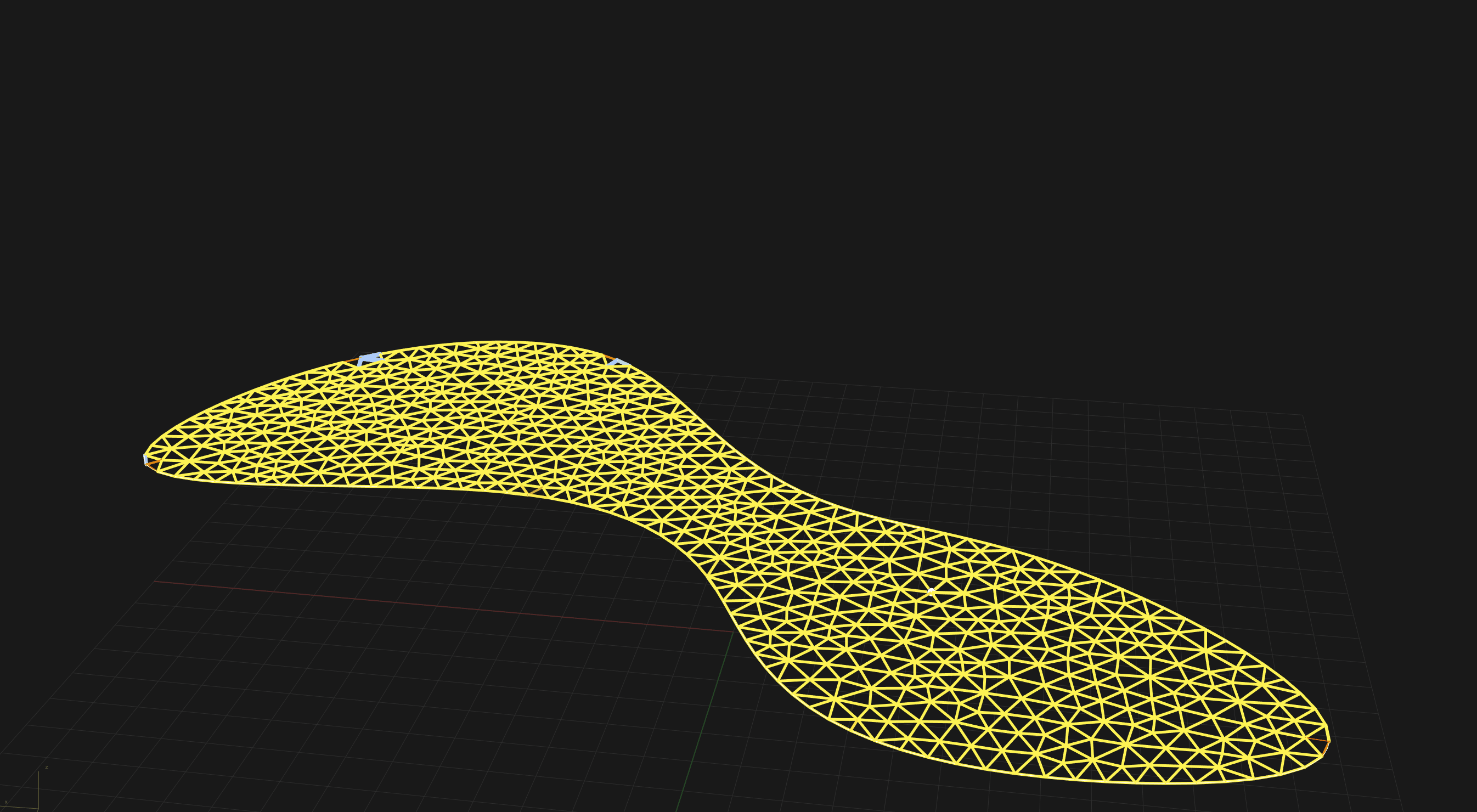
Generative design, on the other end, is designing how the data is used to produce many shapes and solutions as the result of automated analysis. Designing this way can easily be misinterpreted with a level of absoluteness or best solution in the designer’s mind. In its simplest form, it takes the resulting insight from a predictive method and adjusts a set of variables that generate a solution, or set of iterative solutions. In this way, it attempts to provide a “form” that satisfies a set of “functions” based on a set of performance goals. Ultimately, the user is still on the hook for deciding if any of the outcomes are worth using and picking one.
The truth of what each method can provide and what is best for the future of architectural design is somewhere in the middle. Debates for and against generative design are currently underway citing common topics of its potential, applicability, or doomed-ness (hi, Daniel) for building design. Predictive analysis is heavily used in the engineering steps of simulating critical performance levels structure, mechanical systems, and environmental metrics, but it appears to be overthrown by the lure of generative design promises when looking at recent funding and marketing trends. I’m not sure these promises can be upheld.
Now that we’re clear on what each method is regarding architectural design, we can get to the good stuff. The following aspects are descriptions of the parameters and results for both methods. Knowing how to breakdown each aspect will help anyone understand what can be reasonably expected when designing with predictive versus generative design these methods. In the end, you can determine which computational design method is most beneficial for your workflow.
Quantitative & Qualitative Measures
The best architecture will derive from both rational and ineffable decisions. — Phillip G. Bernstein, Architecture Design Data

A digital analysis doesn’t mean the result will be the “best,” but rather the best of what you told it to solve. The key metrics for what it should measure and what can be measured are the secret, and that takes expertise.
Predictive and generative design methods are both intrinsically mathematical operations and are, therefore, dependant on quantitative methods and not qualitative methods. An oversimplification of these two is that quantitative methods interpreting measurements of quantities (like data or numbers), while qualitative methods interpret the quality of how things feel (so, feelings or experiences.) What is being measured is the key difference here, and is often not easily coded into ones and zeroes.
Trying to quantify qualitative measures is like trying to describe the feeling of the number 42. To some, it’s the meaning of life, while to others, it represents the number of delicious tacos they just ordered. The point here is that there has to be a qualifier (tacos) next to the quantifier (7) for that data to mean anything. Designing the relationship between what we quantify and what that means (the qualifier) is the job of the human specialist, and is easiest to understand with predictive design methods. Embedding the meaning of a data point within an algorithm for generative models is possible, but it begins to limit the capability of that algorithm.
Any digital analysis doesn’t mean the result will be the “best,” but rather the best of what you told it to solve. The key metrics for what it should measure and what can be measured are the secret, and that takes expertise. If we are able to mathematically compute the effects of building performance like those elements of daylight, energy use, and structural capability, then we can predict the performance of every iteration with the right set of simulations. Once you get the initial algorithm right, automation and advanced algorithms like machine learning can leverage that experience to its fullest potential. Well, sort of.
Automation & Machine Learning
The “designers’ role will be to support the social conscience of the product. Because the product is no longer the product, it’s now the people.” — John Maeda, EVP Chief Experience Officer at Publicis Sapient

Conceptually, predictive methods leverage automation to holistically study many parameters to predict the performance of the design state to make informed decisions. Generative design works in somewhat the inverse way, leveraging automation algorithms to generate singular alternative solutions for one problem with various parameters and objectives.
How we measure the things we can measure is another distinctive aspect. The holy grail for digital productivity is the data of one digital thing that supports the intelligence of another digital thing. There is a movement happening right now with technologies like artificial intelligence and deep learning neural networks striving to uncover qualitative insights through quantitative methods. In a way, we’re looking to create digital hands to help us feel out different solutions.
In architectural design, it clasps onto the hope that if we crunch enough variables and evaluate enough data we will come to an optimal “Best-fit” solution. However, as Daniel Davis so eloquently points out, regarding buildings, generative design can’t and will probably never provide such a perfect holistic solution. It simply can’t measure enough of the stuff we use to make qualitative decisions.
Still, there are levels of insights that designers can meaningfully uncover for specific design goals and at different stages throughout the design process.
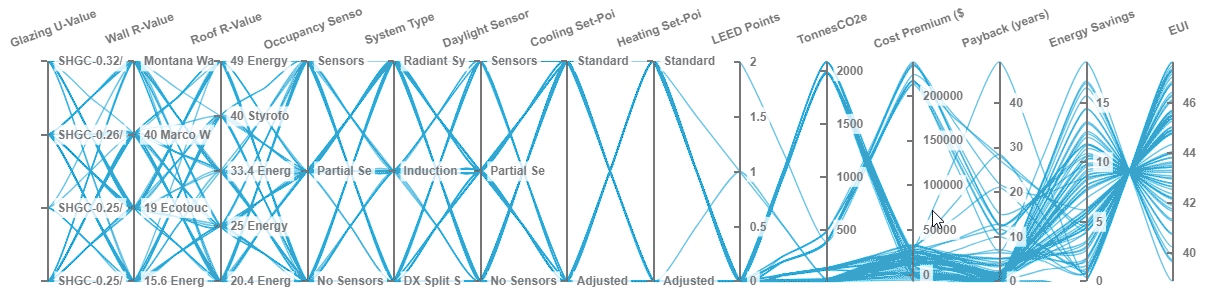
In its basic conceptual form, predictive methods leverage automation to holistically study many parameters of the current design to make informed decisions. For example, cove.tool’s cost versus energy optimization uses machine learning to simulate a network of interconnected building performance metrics and their cost to determine the holistic effect of alternative parameters collectively. One form and various attributes of it that change resulting in feedback to the user as a prediction of that bundle of parameters.

Generative design will work in somewhat the inverse way, leveraging automation algorithms to generate singular alternative solutions for one problem with various parameters and objectives. In this way it strives to answer one question considering (at best) a small set of objectives, often generating a form to fit the criteria. One example shows the method of determining the performance of various geometries regarding radiation as thermal performance for a self-shading strategy. Many options are provided, a shortlist of options is chosen, but in the end, it is solving one part of the equation and may need to be revised upon new data later.
The hope is that machine learning can then be leveraged to train computers to recognize good performance criteria or bad therefore help generate more informed design solutions. Doing so will certainly help determine the effective value of one design over another, but the decision-making needs to be handled with careful consideration so as to maintain a balance of trust and usefulness.
Trust & Usefulness
An algorithm is not blissfully rational and free from the inherent leanings of its designer. — Philip G. Bernstein, Architecture Design Data

No matter how cool or persuasive a solution may appear, it is always biased toward the code and the coder that made it. We can partially account for this through ethics and equality. Just because we can do something doesn’t mean we should, and it does not automatically make it useful.
In every computer program, there are assumptions made to help the design process be more automated, smarter, or simply more useful. A clear of example of this is the output building from modern modeling tools like Sketchup or Revit. SketchUp is a simplistic modeling platform and leaves a mark of that simplicity in the final output. Revit is a highly structured building information modeling software tool seeking to automated the coordination of digital objects, final construction drawings, and accurate representation. Sometimes these tools don’t allow the designer to achieve the desired concept and in that way is biased toward a common output supplying in one way and restricting on another.
The point I’d like to underline here is the fact that tools dictate what we can and cannot do. When they are useful for what we want, great. When they stop being so, no bueno. We like to think digital tools are objective because it is made up of 1’s and 0’s, but they are wrought with explicit definitions and assumptions that a person (or persons) decided should be a certain way. A negative bias can partially be overcome through ethics and equality, but if that is absent we are at the behest of the coder.

In response, architects, engineers, and designers have become the coder and are developing AEC specific predictive and generative tools to account for this bias. Groups like ACADIA are explicitly organizing efforts to create and explore computational design in architectural design. Data-driven and generative design methods have increased in the work presented in these annual conferences and the work produced by its members. However, as we explore this exponential realm, we should keep in mind that just because we can do something does not mean we should or that it will be automatically useful.
The computer will never know what it is making. It can provide a synthetic visual or auditory replication of it, but it will never know what it is or what it means. It has no awareness of the billions of variables outside of the explicit conditions provided to it, but we do. Determinant methods for worthy solutions require checkpoints from a set of experienced professionals. When those professionals start refuting the applicability of a tool, it is time to listen and evolve.
Quality & Control
More lines on paper do not mean a commensurate increase in quality — Barkin

What is the right question? We have to maintain a diligent effort on what we build into our process to ensure quality and control on what we create. Ensuring that the tools we make are sourced from asking the right questions will help us instill the proper caution when selecting data-driven solutions or generative outcomes.
The role of responsible control as laid out by NCARB and expounded on by Philip Berstein in his book Architecture Design Data. He makes the argument that with the ever-evolving productivity of digital tools people are still on the hook for maintaining responsible control of the end product. (are you sensing the theme here?)I would argue that predictive analytical tools are natively designed to provide a regular interjection of human control as the feedback loop includes the person through the process. The hope with generative design tools is to build-in a lot of intelligence to spit out a meaningful outcome, but by that logic, the human is not integrated into the process unless they are the one designing the generative tool.
Just because we can make 1 million iterations or variations of the one concept does not mean we should, especially if we don’t have the time to sift through the details of each one. Daniel Davis makes this point in a recent post on the doomed future of generative design, and I think he’s mostly right. Just because you make something, doesn’t mean it will be the right thing to make. What happens when we stop thinking like Louis Kahn asking the “brick what it wants to be and lose our insight to make the argument for better decisions?” (my short answer, post-modernism, but I digress.)

One of my mentors, Upali Nanda, has imparted a lot of wisdom impacting my professional career and personal outlook on life. Something she repeatedly emphasizes is “What is the right question?” We have to maintain a diligent effort on what we build into our process to ensure checks and balances on what we create. Ensuring that the tools we make are sourced from asking the right questions will help us instill the proper caution when selecting data-driven solutions or generative outcomes.
It is the professional organization of architects, engineers, contractors, sub-contractors, developers, and owners that work together to ensure our cities progress and develop for humanity’s sake. It is on the shoulders of architects as the assigned professional coordinators to ensure these efforts are maintained for public welfare and protection. It is therefore on the shoulders of architects to ensure the tools and methods we develop and use allow for the same.
Economies of Scale & Diminishing Returns
A surplus of ideas is as dangerous as a drought. — Scott Belsky, Making Ideas Happen

At some point, you will spend more time and money than you’ll gain by using one method or the other. The key is to know if you are to making Fords or Ferraris.
In economics, the valuation of any business or entity is directly related to the efficiency of it to produce a product or service. Economies-of-scale refers to a proportionate saving in costs gained by an increased level of production. If you can make more things for less cost, you make more money. The hope for generative design methods is to expand the number of forms to be analyzed so the design of each one costs less. But, there is a diminishing return after a certain number of solutions, because you can only pick one after all.

Diminishing returns refers to a point at which the level of profits or benefits gained is less than the amount of money or energy invested. If it takes too much time or money to make the final product, it may not be worth it. Generative models like the sample above produce project-specific solutions, but may not scale to other projects unless you have similar conditions. Predictive design leverages these concepts to use as many known variables as possible and get to meaningful design insights quicker, limiting the amount of time spent on analysis and alternatives.
It’s a bit like Ford v. Ferrari. Ferrari’s are arguably the most perfect racing machine ever designed. It took Enzo Ferrari and his team decades to perfect the system of systems that have won the most Gand Prix championships of all time. They do one thing amazingly well, like a generative method for design, even if that effort only returns in wins and not revenue. Ford, consequentially, prides itself on efficient and equitable business. It produces millions of one type of product. At some point, you will spend more time and money than you’ll gain by using one method or the other. The key is to know if you are to making Fords or Ferraris.
Moving Forward
I’m writing this in hope that both non-specialists and the tech. savvy alike can begin to see the value of using automation in the right way. A wave of automated digital tools powered by AI is already integrating into our everyday workflows. Parametricism is pressing beyond avant-garde aesthetics as automation and machine learning are helping to uncover design solutions for more durable, sustainable, and better performing architecture worldwide. These tools can benefit the way we design, engineer, and build our cities if we can learn how to value them and integrate the right tools for the right part of the job.
I suggest making it a habit to step back and ask the right questions.
- Is this a quantitative value or a qualitative one, and am I interpreting the data correctly?
- How can I leverage automation and machine learning, and do I want a variety of forms or meaningful insight into the current design?
- What are assumptions that control the outcome of my design, can I trust it, and is it useful?
- Who is overseeing the quality of these results and am I depending on the computer with checks and balances?
- Do I get to the right design insight or am I losing value because the results take too long or too much effort?
Specialty design conditions appear to be the best area to use generative design for performative facades, curvilinear forms, and possibly early-stage planning layouts. However, those areas are a very limited portion of the whole building and are often more expensive keeping them out of reach for lower budget work that makes up the majority of new architectural projects. With the new environmental regulations, limited financial stability, and a climate crisis to help keep the human species living through the next century, we have to find ways to allow design performance measures for these goals to inform all work moving forward.
Predictive design processes are best for whole building design and make the largest contribution to our world. The performance goals and what is being measured are explicit, easy to comprehend and keep expectations limited to the simulation. Automating predictive simulations is easier to manage, provides a quicker response time for design insight, while not restricting the output to a particular form. Predictive design processes tend to limit misinterpretations while maintaining control through co-authorship of results between humans and computers.
All of these benefits make the predictive processes easier to adopt, finance, and learn across all types of teams, buildings, and workflows. With less than 10 years left to meet 2030 goals, and less than 15% of new projects meeting those goals, we have a long way to go with limited resources. Generative tools are fantastic, but integrating automation through performance-based predictive analysis will be what makes the most impact on achieving our goals. Consider what you need most early in the design process that can make the most informed decisions along the way. We all need to think about how to leverage automation for a moire integrative workflow, and what tools make it easiest to learn and apply those processes. I hope this helps clarify the next conversation you have about predictive or generative design and arm you with the knowledge to evaluate the next wave of digital tools.
Cheers!
Recommend
About Joyk
Aggregate valuable and interesting links.
Joyk means Joy of geeK