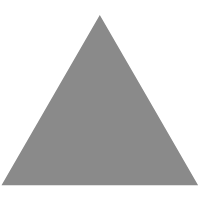
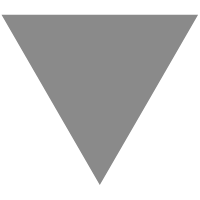
A Matrix for Symbiotic Collaborations Between Humans and Machines
source link: https://hackernoon.com/a-matrix-for-symbiotic-collaborations-between-humans-and-machines-w95j33sw
Go to the source link to view the article. You can view the picture content, updated content and better typesetting reading experience. If the link is broken, please click the button below to view the snapshot at that time.
A Matrix for Symbiotic Collaborations Between Humans and Machines
















@jouvenotBertrand Jouvenot
Advisor| Author | Speaker | Teacher| Blogueur
What is the true face of Artificial Intelligence (AI)? Can it bring many benefits? Unhesitatingly, yes. Can it bring any harm? Alarmingly, yes. A thorough and detailed study of the most prominent AI interfaces from Google to Uber was conducted by us.




The study highlighted familiar, and widely used and accepted AI such as IBM’s Watson, Apple’s Siri, Amazon’s Alexa, and Google Assistant. It shows us exactly how alarming this state of affairs has become.




The situation is serious and it may prove good advice to regain control before it’s too late. This is because we have yet to invent the ultimate matrix designed to construct the best possible scenario of collaboration between human and machine. May this first draft of such a matrix begin the conversation.




Why humans must learn to collaborate with machines
Artificial Intelligence is everywhere these days. It has invaded our homes. It has invaded our workspaces. It has invaded our lives. There is no point in resisting. Rather, the best path is to create and make the best of our mutual collaboration. In order to do this, we must form an alliance between human and machine.




We can start by keeping in mind the many encouraging conclusions that can be drawn from our first lessons learned from our experiments with AI. One of those lessons is that the relationship between humans and machine can prove to be very complementary. Another discovery is that humans can continue to progress and thrive even with constant contact with AI. Also, even though we are only in the early stages of our relationship with AI, we can ascertain with near certainty that machines will continue to adapt to us more and more… Etc., etc., etc. And the list goes on….




However, the potential disasters that artificial intelligence carries includes the destruction of jobs, intervening in and invasion of our lives, and the breach of privacy. We also face the prospect of political interference, possible amplification of fake news, and the creation of “wealth” at the grave expense of natural prosperity to name just a few.




The matrix of collaboration
Since we’ve come to the understanding that sooner or later, whether we like it or not, we will have to cooperate more and more with robots. By robots, we mean everything related to Artificial Intelligence such as algorithms, avatars, voice assistants, intelligent terminals, and all manner of modern robotics. Therefore, the time has arrived for us humans to form a solid foundation for this new matrix that will guide us, empower us, and enlighten us. By doing so, we will be able to create and keep a firm hold on and solid control of such a future collaboration.




Two key axes




Since humans and machines must work together to synergize their strengths, their capacities and their respective assets, let us draw one axis for humans and let us draw one axis for machines.




On the abscissa, we see the axis representing the level of collaboration of the human with the machine. The higher the level of collaboration, the more the algorithm will move to the right.




On the ordinate, we see the axis representing the level of collaboration of the machine with the human. The higher the collaboration, the higher the algorithm will be positioned.




Four possible configurations
Four possible scenarios emerge from this model.




Square A: Should most of the data land in Square A, located at the top left, this would indicate that the collaboration is not optimal because whether or not the human is in control, there is little that he or she is contributing as compared to the machine. The result, then, would be an asymmetrical combination in which the machine has an advantage over the human, possibly at our expense.




Square B: Should most the data fall in the B Square, located at the top right, the collaboration is optimal since the human and the machine collaborate with one another fully, in complete synergy. This indicates the maximized result.




Square C: When most of the data falls into the C Square, located at the bottom left, collaboration is not optimal. This is because in this scenario, the machine does not really collaborate. The result is an asymmetric combination in which humans have an advantage over the machine, at its expense.




Square D: When the data hits Square D, located at the bottom right, the collaboration is not at all optimal since neither the human nor the machine collaborates fully. This gives minimal results for both the Human and machine.




An ideal scenario




Naturally, one ideal scenario is that in which humans and machines are in synergy, working together for the greater good. In this model, both human and machine would collaborate to the point where they are reaching together, to not only produce a result that would have been impossible to be obtained otherwise, but to also discover possibilities that have never before been envisaged.




The necessary determination of the nature of the collaboration
To that end, it becomes necessary to first define the collaboration of humans with respect to the machine, then define the collaboration of the machine with respect to humans, and lastly, define the collaboration of their mutual efforts.




The collaborative effort of human with the machine
In next figure, we will find twelve criteria that can be used to evaluate the collaboration of humans with respect to the machine in this human-machine relationship:




The human:
Behaves like a master by giving instructions to the machine like a servant while still listening to the servant’s expert advice.- Also assumes the role of a manager while considering the machine an employee, therefore specifying objectives, gathering feedback, making the decisions, and so forth.
- Provides clear and unambiguous instructions.
- Strives to understand the machine’s logic while continually gaging its progress.
- Uses at least 25% of the machine’s capabilities.
- Admits that he or she is incapable of considering all possible contingencies.
- Uses an alert system to regulate the balance of power between humans and the machine.
- Understands the machine with an open mind, and without any hidden agenda, without any unnecessary fear and without any preconceived prejudice.
- Manages all the stress and fatigue, doubt and failures, exhaustion and more, that will inevitably arise.
- Benchmark him or herself with other humans who are living the same experience.
- Avoid competing with the machine.
- Constantly review and test all assumptions made about the behavior of the machine.
The collaborative effort of the machine with the human
Ten criteria can be found in the next figure and can be used in this matrix to measure the degree of collaboration with respect to the machine within this human-machine relationship:




The machine:
1. Scrupulously follows the three rules of cybernetics:




- a A robot cannot harm a human being. Nor, while remaining passive, allow a human being to be exposed to danger;
- b. A robot must obey the orders given to it by a human being, unless such orders conflict with the first law;
- c. A robot must protect its existence as long as this protection does not conflict with the first or second law.
2. Has a set of known, up-to-date, clear, and concise operating rules that anyone can easily understand.




3. Provides clear, real-time data about the human’s progress, its own progress, and the mutual, common progress of both the human and machine. The word “attack” is not limited here to a narrow meaning that refers only to possible physical attacks, but to a meaning that also encompasses possible physical attacks (destabilization, harassment, burn out, etc.).




4. Adapts to the human.




5. Respects, reacts with, and responds to the specificity of each user. This includes—but is not limited to—each human’s predispositions and skill level, intellectual preferences and even noticeable psychological traits.




6. Provides regular proposals, or counter-proposals, for joint work, and explains why.




7. Continually adapts to the rhythm of each user over time.




8. Takes into account that some data, on which its received information is based, may sometimes be biased.




9. With all transparency, vigorously manages the delicate balance between unsupervised learning, supervised learning and reinforced learning.




10. Continuously adjusts to produce the desired result.




The mutual effort of the human and the machine
In addition, the next figure gives twelve criteria that can be used to measure the degree of collaboration of both human and the machine in this human-machine relationship:




Both human and the machine:




1. Agree to conduct feedback sessions in order to learn together.




2. Share a common understanding of the intended result.




3. Measure and determine, in real-time, that the result remains in progress at all times.




4. Periodically perform more in-depth analyzes to assess whether the machine is behaving as expected in order to take any necessary steps to assure that it is.




5. Compare with other human-machine pairs, their own individual collaboration.




6. Strive to deeply understand each other’s preferences, learning styles, work patterns, strengths and weaknesses.




7. Show assertiveness.




8. Have realistic and reasonable expectations of one another.




9. Accept the expertise of each other’s respective fields and/or topics without trying to “be better” than the other in that field or topic.




10. Determine how to best share only the data necessary for the machine to operate against the eminent need of and deepest respect for human privacy.




11. Plan any re-education of the machine needed in case of possible divergence, deviance, or gap between expectations and results.




12. Aim for information asymmetry close to zero as described above in our drawing of the two axes.




The need for a simple yet robust scoring method
Once set, criteria for assessing the level of collaboration between humans and one or more artificial intelligences can be rigorously measured with a simple scoring system that uses a scale ranging from one to ten (one being the lowest level of satisfaction of the criterion and ten being the highest level of satisfaction).




This collaboration can be rated in several different ways:




- Self-evaluation: Collaboration rating is performed by the human involved in this human-machine relationship.
- External evaluation: Collaboration is rated by an external and objective person in the form of an evaluation.
- Automated evaluation: Collaboration is scored by the machine only.
- Symbiotic evaluation: Collaboration is rated by both the human and the machine during each of their respective assessment sessions as seen in Figure 9, numbers 3 and 4 (“The necessary mutual collaboration” section above).
- Statistical evaluation: Collaboration rating comes from comparing similar human-machine relationships.
While there certainly remain even more (and possibly endless) options, we will stop with these suggestions, preferring to leave the rest to those experts who have more experienced in this specific methodological point.




Five case studies we can use to test the matrix
Now, we can go ahead and bring our matrix into real life examples, using it to study five human-machine relationships. Three of these human-machine relationships are well known and the other two may prove a bit of a surprise.




Google’s unwittingly ambiguous conception of equality.








Indeed, let’s take two people, Nathalie and Paul. Nathalie is an uptown girl, born to an affluent family and raised in a spectacular home in a beautiful neighborhood. Having also received the best education, she has just started Harvard University to study engineering.




Meanwhile, Paul comes from a significantly more modest background and only by dint of courage, has he become the pride of his parents. Neither parent had a degree and now their son has been admitted to the nearby IT Institute. It’s not far, located in the largest city closest to the small town where Paul was born and where English is a second language.




In our story, we see that Nathalie and Paul are both passionate about Artificial Intelligence. They both sit down to do a Google search of AI but—they both do it differently.




Nathalie types in “Artificial Intelligence” knowing exactly what links to click on. She knows that the labs of American universities like MIT or Stanford are at the forefront on the subject and she will click only on links to Native English sites from prestigious research centers she knows. What’s more, she will do this naturally without even thinking about it because it is so ingrained into her skillset.




Google’s algorithm will understand what she is clicking and why she is clicking it. With each new search, Google will continue showing more high quality sites in English.




As for Paul, who is barely familiar with MIT and its MediaLab, the story’s not the same. When he searches “Artificial Intelligence,” he does so in French, which is his native language. He then clicks on the links and of course they are French sites. By doing this, he inadvertently invites Google show him French language sites, not English (which does have a few sites that provide articles on Artificial Intelligence that are readable even to an old Frenchman who is allergic to English).




Certainly Google’s algorithm is just following the perceived commands but it is far from meeting many of the previously mentioned criteria. For example, it does not take into account the fact that the data on which it is based can be biased as seen in criterion 8.




Here, Benedict has put Google’s algorithm on the wrong path by directing it to French. At the same time, Google takes into account similar requests from other users, lumping Paul in with them, rather than with university peers, further depriving him the opportunity to compare his collaboration with Google with other human-machine pairs (criterion 5).




Most importantly, we are far from our goal of keeping information asymmetry close to zero (criterion 12). In this case, Google occupies Square A on our matrix.




Uber’s blatant employee dis dubiously disguised as customer service




Enter Lyft. In the United States, many drivers who started out working on both platforms, have ditched Uber for Lyft. This is because Lyft’s algorithm is more geared towards the interest of their drivers, rather than passengers.




Other taxi services are also trying to improve on Uber’s model. Like Lyft, Sidecar and SherpaShare focus attention on their drivers using reverse auctions to send those drivers to passengers. Thereby, they notify the closest, most popular driver (with the best reviews), to pick up the passenger thus assuring that other drivers are not having to scramble around for nothing.




So we see that with Uber, like Google, the information asymmetry is not zero and many criteria mentioned above have not been met. And, like Google, Uber will also occupy Square A of our matrix.




Amazon’s shameless voyeurism!




First, Google’s terminal must “hear” the user say the word, “Google,” when speaking to it. For instance, one would say, “Hello Google, what is the capital of India?” or “Hello Google, do you know the price of Bitcoin?” or “Hello Google, can you tell me what the weather in Paris will be tomorrow?”




In contrast, Amazon calls its AI powerhouse the cute and clever name of Alexa and requires users to use Alexa’s name to get answers, rather than directly addressing Amazon. In effect, this antic by Amazon causes users to forget that they are actually communicating with the most powerful online merchant site, not just an “information” source. Instead, they refer to Alexa as “her” and “she,” thus elevating her position as not just an assistant, but as a personal assistant, rather than realizing that they are actually communicating with a highly trained sales professional.




Secondly, Google requires the physical intervention of its users, as they must press the on/off button. This may seem trivial, until we understand that Amazon is, in contrast, constantly awake, constantly listening, and constantly calculating. All with one ultimate, ulterior motive: get you to buy from them.




Amazon’s Alexa is designed to get all the information “she” needs so that the e-commerce giant can easily and seamlessly market relevant products directly to you so that you will buy from Amazon. When Amazon’s Alexa “hears” a crying baby, this causes her to understand that there is a small child at home and allows her to suggest to the parents that they purchase diapers. When the father coughs, it signals Amazon’s Alexa to promote over-the-counter medicine (or even, perhaps, prescription) such as cough medicine and lozenges. The sound of a plate that falls accompanied by the cries of the mother, “And there we go, another broken plate!” thus allows Amazon’s Alexa to propose new tableware that the frustrated and busy mom doesn’t even have to leave her home to buy. Just a few clicks of a button and she’s got new plates on the way.




As we can see, with Amazon’s avaricious Alexa, many of the previously mentioned criteria are flouted.




Upon a purely rational evaluation, one might conclude that Amazon’s model is perfectly efficient and innocently altruistic. However one must still hold the reservation that users may not necessarily realize that Alexa is constantly listening and they can quickly forget about it over time. It is easy underestimate the capacity of the artificial intelligence that hides within and works in such a secretive, powerful, and intrusive way.




Therefore, Alexa deserves its (not her) place in Square A, right alongside Google and Uber.




SaneBox: A little patience please




When you download SaneBox, it quickly explains how it will, with your consent, create folders inside your inbox. Sanebox’s algorithm will sift through your emails and place them where it assumes they should go. The first folder is for those emails that appear to need to be read within the hour, another folder for those deemed to be read once a week, and another for those the algorithm has determined should be viewed as little as once per month. Other folders, such as one for spam and one for e-mails that need a response, are also used.




SaneBox values its model of transparency concerning the logic that it follows and above all proposes to learn with you by positioning itself as your diligent and obedient assistant. Part of that learning includes self-correction. Whenever its users correct a bad prediction made by SaneBox by moving their e-mail from one folder to another, SaneBox will learn from its mistake and no longer reproduce the errorwill not repeat it.




For example, when a user receives a monthly phone bill from their telecom operator, SaneBox will put the email in the “Read this month” folder. But, if this does not suit the user because they would rather it be in their weekly folder so that they don’t forget to pay the bill, the user can move the email to a folder they name, “Invoices Due Now.” The following month, SaneBoxe will know to send the email directly into the new folder.




This example of human-machine relationship places SaneBox firmly in Square B of the matrix.




Focus@Will and its harmonious approach




They guessed right. This handy AI delivers a choice of musical genres and songs to its users who keep the ones that they know and like, and sample any proposed music. When they begin a task, Focus@Will plays their music choices for the measured amount of time taken to carry out that specific task. Examples can include writing up a proposal, producing a wind chart, etc.). Each time a task is finished, Focus@Will offers new music in order of its algorithms and in succession in order to determine what music will further improve the user’s productivity. All of this is, of course, accomplished with the active and consenting contribution of the user.




Focus@Will’s solution gives it its rightful place in Square B of the matrix.




Possible uses:
Not just a simple algorithm analyzer, it’s important to know that the matrix also can contribute in other ways and for the longer term. Here are some possible uses:




In the context of computer development
Where coders are increasingly confronted with Artificial Intelligence and algorithms that they must develop, enrich, maintain, study, use, and sustain.




In industrial or logistical environments
Where it’s no longer an OS2 environments and instead, the work focus is now more and more on robots that are, in turn, more and more intelligent.




In the digital sector, of course
In which most start-ups rely heavily on Artificial Intelligence and where those who manage the AI will ultimately learn to treat all AI as a workers—employees, if you will—in short creating a new generation of colleagues.




For human resources and managers
Those who are concerned with production, productivity, workplace wellbeing, and branding, and who see the increase in the direct and indirect interaction of their teams and collaborators with machines—even if only in apps such as Slack.




For politicians facing unprecedented challenges
Who use Google (which touches on knowledge), Airbnb and consort (who use regulated sectors), or any number of programs or apps that control cyber security, or cyber-delinquency.




For the legislator
To determine who is responsibility for what, such as establishing who is responsible when an autonomous car, driven by a computer program, causes an accident. Whose fault is it? The driver on board who did not use the manual mode, the vehicle manufacturer, or the fault may possibly lie with the program developer.




In the field of health




Where patients are increasingly forced to let machines collect data on them, and where the medical profession is facing a job that’s been literally taken over by new technology.




In education




Where we know that a large part of success is due to the personal efforts of the students and in this world every student turns to sites such as Wikipedia and Google to get their information.




Precautions
Using this matrix means using necessary precautions that are scientific, methodological and practical. Here are a few:




The moving and hyper-evolutionary digital character in which the algorithms work makes any conclusions about them likely to quickly become obsolete or simply invalidated.




The greater or lesser permeability of human to machines must be taken into account. Evidence suggests that the generation of an individual may have a strong influence on the human-machine collaboration. This may also be the case when comparing nationality (the French are particularly sensitive to the questions relating to the private life and the confidentiality of the data, while the Chinese are much less concerned about how their data is used).




The young age and immaturity of Artificial Intelligence is also to be taken into consideration. It is still in its infancy. Remember that it was developed quickly, continues to develop quickly, and may grow to quick to handle because of its lightning fast, self-learning abilities. This is a completely new environment with constantly emerging technology. It would therefore be unwise to draw too hasty a conclusion about it.




Developers that shroud their technology with secrecy and possible deception, is a determining factor in the veracity of its analysis.




Data biases related to the quality and reliability of the data used by an algorithm is a crucial element that, thus far, influences their efficiency.




The propensity of humans to produce an Artificial Intelligence robot or other program that in some respects resemble them, rather than being different or foreign, is to be noted. Remember that it was when humans stopped copying nature, especially birds, that they found the solution to fly their planes—a technology that in no way mimics any flying animal.




Remember to keep in mind the immaturity of machines that are still in their childhood and know that it is possible that they may grow too quickly because of their super-fast, self-learning ability.




Next steps
With the first reflection on this subject now established, we can see that the approach to Humans collaborating with machines is speculative, open, in progress, much like fresh paint on a white picket fence. This idea of a matrix is not meant to be strictly definitive. We are simply opening the discussion because we have all come to live in a digital world where open innovation is now a reality, transversal collaboration is possible, and updating is a perpetual necessity.




Now we turn to you.
Your testimonials, reactions, intellectual enrichment, donations or any provision of resources such as machines, brains, and labs are also welcome. We also invite machines—should they feel capable of contributing—to the table. They are more than welcome.




Thanks
We would like to offer our sincere thanks to a few authors whose works have greatly contributed to the development of this matrix: Tim O’Reilly, Kevin Kelly, Simon Head, Erik Brynjolfsson, Andrew Mcafee, Salim Ismail, Michael Malone, Peter Thiel, Black Masters, and Scott Galloway.




Also published here.




















Create your free account to unlock your custom reading experience.
Recommend
About Joyk
Aggregate valuable and interesting links.
Joyk means Joy of geeK