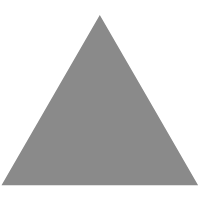
5
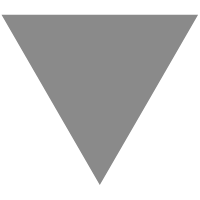
Yet Another Math Programming Consultant
source link: https://yetanothermathprogrammingconsultant.blogspot.com/2021/03/a-difficult-multiline-regression-data.html
Go to the source link to view the article. You can view the picture content, updated content and better typesetting reading experience. If the link is broken, please click the button below to view the snapshot at that time.
A difficult multi-line regression data set
In [1], I discussed some models for a small 2 line regression problem. Here I want to dive into a more challenging problem.
Data generation process
I started with the following five lines: f1(x)=200−3xf2(x)=150−xf3(x)=10f4(x)=−50+2xf5(x)=−120f1(x)=200−3xf2(x)=150−xf3(x)=10f4(x)=−50+2xf5(x)=−120 To generate points, I used xi∼U(0,100)ki∼U{1,5}ϵi∼N(0,15)yi=aki+bki⋅xi+ϵixi∼U(0,100)ki∼U{1,5}ϵi∼N(0,15)yi=aki+bki⋅xi+ϵi
This corresponds to the following picture:
Now, we drop the lines and just keep the points. Together with K=5K=5 (the number of lines), this is all the data we give to the model:
Looking at this picture it seems a very difficult task to recover our 5 lines.
The data set is thus:---- 32 PARAMETER x data i1 5.141, i2 0.601, i3 40.123, i4 51.988, i5 62.888, i6 22.575, i7 39.612 i8 27.601, i9 15.237, i10 93.632, i11 42.266, i12 13.466, i13 38.606, i14 37.463 i15 26.848, i16 94.837, i17 18.894, i18 29.751, i19 7.455, i20 40.135, i21 10.169 i22 38.389, i23 32.409, i24 19.213, i25 11.237, i26 59.656, i27 51.145, i28 4.507 i29 78.310, i30 94.575, i31 59.646, i32 60.734, i33 36.251, i34 59.407, i35 67.985 i36 50.659, i37 15.925, i38 65.689, i39 52.388, i40 12.440, i41 98.672, i42 22.812 i43 67.565, i44 77.678, i45 93.245, i46 20.124, i47 29.714, i48 19.723, i49 24.635 i50 64.648, i51 73.497, i52 8.544, i53 15.035, i54 43.419, i55 18.694, i56 69.269 i57 76.297, i58 15.481, i59 38.938, i60 69.543, i61 84.581, i62 61.272, i63 97.597 i64 2.689, i65 18.745, i66 8.712, i67 54.040, i68 12.686, i69 73.400, i70 11.323 i71 48.835, i72 79.560, i73 49.205, i74 53.356, i75 1.062, i76 54.387, i77 45.113 i78 97.533, i79 18.385, i80 16.353, i81 2.463, i82 17.782, i83 6.132, i84 1.664 i85 83.565, i86 60.166, i87 2.702, i88 19.609, i89 95.071, i90 33.554, i91 59.426 i92 25.919, i93 64.063, i94 15.525, i95 46.002, i96 39.334, i97 80.546, i98 54.099 i99 39.072, i100 55.782 ---- 32 PARAMETER y data i1 192.922, i2 -122.916, i3 21.992, i4 70.273, i5 91.422, i6 109.528 i7 129.230, i8 -140.463, i9 180.013, i10 24.614, i11 -108.546, i12 10.211 i13 -148.679, i14 24.443, i15 122.773, i16 164.505, i17 164.359, i18 132.833 i19 -19.868, i20 15.210, i21 155.782, i22 127.528, i23 122.108, i24 149.691 i25 -0.143, i26 -120.337, i27 94.868, i28 -39.217, i29 96.683, i30 46.506 i31 35.514, i32 22.589, i33 97.811, i34 -113.523, i35 88.707, i36 94.006 i37 9.232, i38 73.173, i39 26.600, i40 0.429, i41 3.943, i42 119.583 i43 101.666, i44 -38.179, i45 39.630, i46 137.980, i47 4.808, i48 18.422 i49 -10.888, i50 83.361, i51 65.726, i52 -39.174, i53 0.179, i54 126.818 i55 139.561, i56 -18.520, i57 105.170, i58 8.206, i59 95.491, i60 87.668 i61 -64.973, i62 12.739, i63 -13.237, i64 -70.810, i65 136.727, i66 178.416 i67 7.676, i68 -51.541, i69 78.491, i70 143.387, i71 115.522, i72 66.261 i73 52.631, i74 -100.755, i75 151.703, i76 47.462, i77 127.759, i78 -65.191 i79 -6.051, i80 137.610, i81 130.662, i82 128.182, i83 148.801, i84 -23.712 i85 -64.504, i86 27.111, i87 150.200, i88 171.403, i89 72.107, i90 -105.643 i91 -112.767, i92 112.218, i93 102.355, i94 5.007, i95 86.645, i96 -127.749 i97 -30.270, i98 79.430, i99 78.474, i100 -7.158
The questions we want to address are:
- Can we use an optimization model to find the best fit for five different lines?
- Do the regression lines we find this way, correspond to the original functions we used in our data generation process?
The model has to make two decisions:
- Assign points to lines.
- Fitting: find the coefficients (constant term and slope) of each line.
I expected this to be not too difficult. This turned out to be wrong.
We have 5×100=5005×100=500 binary variables to model the assignment. This does seem small for a good MIP solver, but remember 25002500 is a big number [3]:
This is a number with 151 decimal digits.
Note: a better measure of size would be 51005100 which is a bit less: 7888609052210118054117285652827862296732064351090230047702789306640625 (70 decimal digits).
Solution process
To make things a bit easier, compared to a least squares objective, I used a linear MIP model based on LAD regression [2]:
Linear LAD Modelmin∑izi−zi≤ri≤zi∀iabsolute valueri=yi−α0,k−α1,k⋅xi+si,k∀i,kfit (possibly relaxed)−M⋅(1−δi,k)≤si,k≤M⋅(1−δi,k)∀i,kbig-M constraint∑kδi,k=1∀ipick one regression lineα0,k≤α0,k+1∀kexcept lastorder by constant termδi,k∈{0,1}min∑izi−zi≤ri≤zi∀iabsolute valueri=yi−α0,k−α1,k⋅xi+si,k∀i,kfit (possibly relaxed)−M⋅(1−δi,k)≤si,k≤M⋅(1−δi,k)∀i,kbig-M constraint∑kδi,k=1∀ipick one regression lineα0,k≤α0,k+1∀kexcept lastorder by constant termδi,k∈{0,1}The big-M constraints implement the implication δi,k=1⇒si,k=0δi,k=1⇒si,k=0i.e. if δi,k=1δi,k=1 then point ii is used in fitting line kk. This model could not be solved to proven optimality. The best bound started out very bad (at zero) and moved very slowly. In the end, I used a heuristic to find a good solution and then used the MIP model to improve upon this using MIPSTART.
The heuristic looked like:
- If time or iteration limit reached: stop
- Generate a random assignment. Evaluate the objective using an L1norm regression procedure (for each of the five lines).
- For each point ii, see if it improves the objective if we assign it to a different line.
- If there is no longer an improvement possible, go to step 1.
This only looks at one point at a time, so it will not deliver optimal solutions. Hence we restart with a fresh random solution if we are unable to further improve the solution. In step 3 I use initially a cheap test: keep the lines constant and only check residuals without rerunning regressions. A more expensive test is to compare the residuals after rerunning regressions. This more expensive test is used once the simple test does not produce reassignments anymore.
The results were:
- Heuristic: obj=957.1482
- MIP improvement: obj=946.787
The picture below is from this solution.
This picture is very much like the picture we started with! This is not bad at all.
The final LAD estimates are:
---- 473 PARAMETER est estimates a0 a1 line1 -123.012 0.160 line2 -66.229 2.246 line3 -0.078 0.041 line4 155.979 -1.123 line5 211.432 -3.213
We can compare this against the coefficients we used to generate the data.
Sidenote: checking the heuristic
Remember, our heuristic would try to move single points to another line. When it finishes it delivers a solution where such a move is no longer profitable. We can check if the heuristic is correct, by solving a slightly different version of the MIP model: restrict the number of changes in the assignment variable δi,kδi,k to one. If this model stops without an improvement, we have some confidence in the solution our heuristic produces.The modeling involved is as follows: di,k≥δi,k−δ0i,kdi,k≥δ0i,k−δi,k∑i,kdi,k≤1di,k∈[0,1]di,k≥δi,k−δi,k0di,k≥δi,k0−δi,k∑i,kdi,k≤1di,k∈[0,1] Here δ0i,kδi,k0 is the solution found by the heuristic. The variable di,k=1di,k=1 if we change an assignment from the heuristic solution.
GA heuristic
I also tried the GA algorithm in R. This heuristic only allows real-valued or binary variables. I used a real-valued vector of length 5 with bounds [1,5.9999][1,5.9999]. The fitness function used this algorithm:
- Use the floor function to convert the reals to integers between 1 and 5.
- Perform an L1 regression on each line.
- The objective is minus the sum of all absolute residuals.
fitness <- function(rx) { ix <- floor(rx) # rx are n=100 real numbers in [1,K+0.999] # ix are n=100 integers in [1,K] obj <- 0 for (k in 1:K) { cnt <- length(ix[ix==k]); if (cnt>2) { r <- l1fit(x[ix==k],y[ix==k]) obj <- obj + sum(abs(r$residuals)) } } return(-obj); }
The simplicity of this is very attractive. Also, it is easy to change the fitness function to work with a least-squares fit.
Note that sometimes the GA algorithm generated an assignment with less than two points to a line. In that case, we don't use regression (that would fail) but conclude the residuals are zero for that line. With two points assigned to a line, we also can prevent doing a regression.
The best objective was: 1092.948. This is not as good as the solution we found before.
As a final test, I did provide our best solution (obj=946.787), and let the GA algorithm run:
No improvement was found.
Conclusions
- This problem was much more challenging than I expected.
- The MIP model based on LAD regression has real troubles, but in the end, it was a good tool to improve the heuristic solution. Sometimes you have to be happy with just good solutions. Looking at the picture, the final solution is matching the original lines quite well.
- I'll keep thinking about this a bit to see if I can come up with a better way to do this.
- Is there a way to find proven optimal solutions for this problem? Maybe even for the least-squares problem? I suspect the combinatorics are just difficult here.
References
Appendix
The best solution I found:
---- 216 PARAMETER sol4b line number, best solution found i1 5, i2 1, i3 2, i4 2, i5 4, i6 4, i7 4, i8 1, i9 5, i10 3, i11 1 i12 3, i13 1, i14 2, i15 5, i16 2, i17 5, i18 4, i19 3, i20 2, i21 4, i22 4 i23 4, i24 5, i25 3, i26 1, i27 4, i28 2, i29 2, i30 4, i31 5, i32 5, i33 5 i34 1, i35 2, i36 4, i37 3, i38 2, i39 5, i40 3, i41 3, i42 4, i43 2, i44 5 i45 4, i46 4, i47 3, i48 3, i49 2, i50 4, i51 4, i52 2, i53 3, i54 4, i55 4 i56 5, i57 2, i58 3, i59 5, i60 2, i61 5, i62 5, i63 3, i64 2, i65 4, i66 5 i67 3, i68 2, i69 4, i70 4, i71 4, i72 4, i73 5, i74 1, i75 4, i76 2, i77 4 i78 5, i79 3, i80 4, i81 4, i82 4, i83 4, i84 3, i85 5, i86 5, i87 4, i88 5 i89 4, i90 1, i91 1, i92 4, i93 4, i94 3, i95 4, i96 1, i97 5, i98 4, i99 5 i100 3 ---- 216 PARAMETER a estimated coefficients, best solution found const.term slope sum|r| line1 -123.012 0.160 105.780 line2 -66.229 2.246 154.042 line3 -0.078 0.041 156.192 line4 155.979 -1.123 324.395 line5 211.432 -3.213 206.378 total 946.787
Recommend
About Joyk
Aggregate valuable and interesting links.
Joyk means Joy of geeK