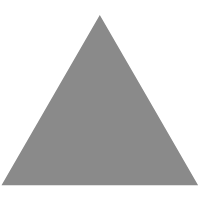
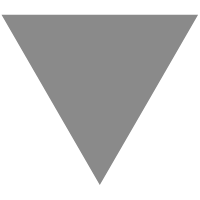
有关语义分割的奇技淫巧有哪些:分享一些实用的纯工程 tricks
source link: https://bbs.cvmart.net/articles/241
Go to the source link to view the article. You can view the picture content, updated content and better typesetting reading experience. If the link is broken, please click the button below to view the snapshot at that time.
作者:AlexL
来源:https://www.zhihu.com/question/272988870/answer/562262315
知乎问题:有关语义分割的奇技淫巧有哪些?
AlexL的回答:
代码取自在Kaggle论坛上看到的帖子和个人做过的project
1. 如何去优化IoU
在分割中我们有时会去用intersection over union去衡量模型的表现,具体定义如下:
在有了这个定义以后我们可以规定比如说对于predicted instance和actual instance,IoU大于0.5算一个positive。在这基础之上可以做一些F1,F2之类其他的更宏观的metric。
所以说怎么去优化IoU呢?¬¬
拿二分类问题举例,做baseline的时先扔上个binary-crossentropy看下效果,于是就有了以下的实现(PyTorch):
class BCELoss2d(nn.Module):
def __init__(self, weight=None, size_average=True):
super(BCELoss2d, self).__init__()
self.bce_loss = nn.BCELoss(weight, size_average)
def forward(self, logits, targets):
probs = F.sigmoid(logits)
probs_flat = probs.view (-1)
targets_flat = targets.view(-1)
return self.bce_loss(probs_flat, targets_flat)
但是问题在于,优化BCE不等价于优化IoU。这篇文章说的显然比我要好,但是直观来说在一个minibatch里每个pixel的权重其实是不一样的。两张图片,一张正样本有1000个pixels,另一张只有4个,第二张一个pixel带来的IoU损失就能顶得上第一张中250个pixel的损失。
“那能不能直接优化IoU?“
可以,但这肯定不是最优的:
def iou_coef(y_true, y_pred, smooth=1):
"""
IoU = (|X & Y|)/ (|X or Y|)
"""
intersection = K.sum(K.abs(y_true * y_pred), axis=-1)
union = K.sum((y_true,-1) + K.sum(y_pred,-1) - intersection
return (intersection + smooth) / ( union + smooth)
def iou_coef_loss(y_true, y_pred):
return -iou_coef(y_true, y_pred)
这次的问题在于训练过程的不稳定。一个模型从坏到好,我们希望监督它的loss/metric的过渡是平滑的,但直接暴力套用IoU显然不行。。。。
于是我们有了Lovász-Softmax!A tractable surrogate for the optimization of the intersection-over-union measure in neural networks
https://github.com/bermanmaxim/LovaszSoftmax
具体为什么这个loss比BCE/Jaccard要好我不敢瞎说......但从个人使用体验来看效果拔群 \ (•◡•) /
还有一个很有意思的细节是:原implementation中这一段:loss = torch.dot(F.relu(errors_sorted), Variable(grad))
如果把relu换成elu+1的话,有时效果更好。我猜测可能是因为elu+1比relu更平滑一些?
1.1 如果你不在乎训练时间的话
试试这个:
def symmetric_lovasz(outputs, targets):
return (lovasz_hinge(outputs, targets) + lovasz_hinge(-outputs, 1 - targets)) / 2
1.2 如果你的模型斗不过Hard Examples的话
在你的loss后面加上这个:
def focal_loss(self, output, target, alpha, gamma, OHEM_percent):
output = output.contiguous().view(-1)
target = target.contiguous().view(-1)
max_val = (-output).clamp(min=0)
loss = output - output * target + max_val + ((-max_val).exp() + (-output - max_val).exp()).log()
# This formula gives us the log sigmoid of 1-p if y is 0 and of p if y is 1
invprobs = F.logsigmoid(-output * (target * 2 - 1))
focal_loss = alpha * (invprobs * gamma).exp() * loss
# Online Hard Example Mining: top x% losses (pixel-wise). Refer to https://www.robots.ox.ac.uk/~tvg/publications/2017/0026.pdf
OHEM, _ = focal_loss.topk(k=int(OHEM_percent * [*focal_loss.shape][0]))
return OHEM.mean()
2. 魔改U-Net
原始Unet长这样子(Keras):
def conv_block(neurons, block_input, bn=False, dropout=None):
conv1 = Conv2D(neurons, (3,3), padding='same', kernel_initializer='glorot_normal')(block_input)
if bn:
conv1 = BatchNormalization()(conv1)
conv1 = Activation('relu')(conv1)
if dropout is not None:
conv1 = SpatialDropout2D(dropout)(conv1)
conv2 = Conv2D(neurons, (3,3), padding='same', kernel_initializer='glorot_normal')(conv1)
if bn:
conv2 = BatchNormalization()(conv2)
conv2 = Activation('relu')(conv2)
if dropout is not None:
conv2 = SpatialDropout2D(dropout)(conv2)
pool = MaxPooling2D((2,2))(conv2)
return pool, conv2 # returns the block output and the shortcut to use in the uppooling blocks
def middle_block(neurons, block_input, bn=False, dropout=None):
conv1 = Conv2D(neurons, (3,3), padding='same', kernel_initializer='glorot_normal')(block_input)
if bn:
conv1 = BatchNormalization()(conv1)
conv1 = Activation('relu')(conv1)
if dropout is not None:
conv1 = SpatialDropout2D(dropout)(conv1)
conv2 = Conv2D(neurons, (3,3), padding='same', kernel_initializer='glorot_normal')(conv1)
if bn:
conv2 = BatchNormalization()(conv2)
conv2 = Activation('relu')(conv2)
if dropout is not None:
conv2 = SpatialDropout2D(dropout)(conv2)
return conv2
def deconv_block(neurons, block_input, shortcut, bn=False, dropout=None):
deconv = Conv2DTranspose(neurons, (3, 3), strides=(2, 2), padding="same")(block_input)
uconv = concatenate([deconv, shortcut])
uconv = Conv2D(neurons, (3, 3), padding="same", kernel_initializer='glorot_normal')(uconv)
if bn:
uconv = BatchNormalization()(uconv)
uconv = Activation('relu')(uconv)
if dropout is not None:
uconv = SpatialDropout2D(dropout)(uconv)
uconv = Conv2D(neurons, (3, 3), padding="same", kernel_initializer='glorot_normal')(uconv)
if bn:
uconv = BatchNormalization()(uconv)
uconv = Activation('relu')(uconv)
if dropout is not None:
uconv = SpatialDropout2D(dropout)(uconv)
return uconv
def build_model(start_neurons, bn=False, dropout=None):
input_layer = Input((128, 128, 1))
# 128 -> 64
conv1, shortcut1 = conv_block(start_neurons, input_layer, bn, dropout)
# 64 -> 32
conv2, shortcut2 = conv_block(start_neurons * 2, conv1, bn, dropout)
# 32 -> 16
conv3, shortcut3 = conv_block(start_neurons * 4, conv2, bn, dropout)
# 16 -> 8
conv4, shortcut4 = conv_block(start_neurons * 8, conv3, bn, dropout)
#Middle
convm = middle_block(start_neurons * 16, conv4, bn, dropout)
# 8 -> 16
deconv4 = deconv_block(start_neurons * 8, convm, shortcut4, bn, dropout)
# 16 -> 32
deconv3 = deconv_block(start_neurons * 4, deconv4, shortcut3, bn, dropout)
# 32 -> 64
deconv2 = deconv_block(start_neurons * 2, deconv3, shortcut2, bn, dropout)
# 64 -> 128
deconv1 = deconv_block(start_neurons, deconv2, shortcut1, bn, dropout)
#uconv1 = Dropout(0.5)(uconv1)
output_layer = Conv2D(1, (1,1), padding="same", activation="sigmoid")(deconv1)
model = Model(input_layer, output_layer)
return model
但一般与其是用transposed convolution我们会选择用upsampling+33 conv,具体原因请见这篇文章:Deconvolution and Checkerboard Artifacts (强烈安利distill,blog质量奇高)
再往下说,在实际做project的时候往往没有那么多的训练资源,所以我们得想办法把那些classification预训练模型嵌入到Unet中。ʕ•ᴥ•ʔ
把encoder替换预训练的模型的诀窍在于,如何很好的提取出pretrained models在不同尺度上提取出来的信息,并且如何把它们高效的接在decoder上。常见的用于嫁接的模型有Inception和Mobilenet,但我在这里就分析一下更直观一些的ResNet/ResNeXt这一类的模型:
def forward(self, x):
x = self.conv1(x)
x = self.bn1(x)
x = self.relu(x)
x = self.maxpool(x)
x = self.layer1(x)
x = self.layer2(x)
x = self.layer3(x)
x = self.layer4(x)
x = self.avgpool(x)
x = x.view(x.size(0), -1)
x = self.fc(x)
return x
我们可以很明显的看出不同尺度的feature map分别是由不同的layer来提取的,我们就可以从中选出几个来做concat,upsample,conv。唯一一点要注意的是千万不要错位concat,否则最后出来的output可能会和输入图大小不同。下面分享一个可行的搭法,其中为了提升各feature map的resolution我移去了原resnet conv1中的pool:
def __init__(self):
super().__init__()
self.resnet = models.resnet34(pretrained=True)
self.conv1 = nn.Sequential(
self.resnet.conv1,
self.resnet.bn1,
self.resnet.relu,
)
self.encoder2 = self.resnet.layer1 # 64
self.encoder3 = self.resnet.layer2 #128
self.encoder4 = self.resnet.layer3 #256
self.encoder5 = self.resnet.layer4 #512
self.center = nn.Sequential(
ConvBn2d(512,512,kernel_size=3,padding=1),
nn.ReLU(inplace=True),
ConvBn2d(512,256,kernel_size=3,padding=1),
nn.ReLU(inplace=True),
nn.MaxPool2d(kernel_size=2,stride=2),
)
self.decoder5 = Decoder(256+512,512,64)
self.decoder4 = Decoder(64 +256,256,64)
self.decoder3 = Decoder(64 +128,128,64)
self.decoder2 = Decoder(64 +64 ,64 ,64)
self.decoder1 = Decoder(64 ,32 ,64)
self.logit = nn.Sequential(
nn.Conv2d(384, 64, kernel_size=3, padding=1),
nn.ELU(inplace=True),
nn.Conv2d(64, 1, kernel_size=1, padding=0),
)
def forward(self, x):
mean=[0.485, 0.456, 0.406]
std=[0.229,0.224,0.225]
x=torch.cat([
(x-mean[2])/std[2],
(x-mean[1])/std[1],
(x-mean[0])/std[0],
],1)
e1 = self.conv1(x)
e2 = self.encoder2(e1)
e3 = self.encoder3(e2)
e4 = self.encoder4(e3)
e5 = self.encoder5(e4)
f = self.center(e5)
d5 = self.decoder5(f, e5)
d4 = self.decoder4(d5,e4)
d3 = self.decoder3(d4,e3)
d2 = self.decoder2(d3,e2)
d1 = self.decoder1(d2)
关于decoder的设计方法,还有两个可以参考的小技巧:
一是 Concurrent Spatial and Channel Squeeze & Excitation in Fully Convolutional Networks,可以理解为是一种attention,用很少的参数来校准feature map,详情请见论文,但实现细节可参考以下的PyTorch代码:
class sSE(nn.Module):
def __init__(self, out_channels):
super(sSE, self).__init__()
self.conv = ConvBn2d(in_channels=out_channels,out_channels=1,kernel_size=1,padding=0)
def forward(self,x):
x=self.conv(x)
#print('spatial',x.size())
x=F.sigmoid(x)
return x
class cSE(nn.Module):
def __init__(self, out_channels):
super(cSE, self).__init__()
self.conv1 = ConvBn2d(in_channels=out_channels,out_channels=int(out_channels/2),kernel_size=1,padding=0)
self.conv2 = ConvBn2d(in_channels=int(out_channels/2),out_channels=out_channels,kernel_size=1,padding=0)
def forward(self,x):
x=nn.AvgPool2d(x.size()[2:])(x)
#print('channel',x.size())
x=self.conv1(x)
x=F.relu(x)
x=self.conv2(x)
x=F.sigmoid(x)
return x
class Decoder(nn.Module):
def __init__(self, in_channels, channels, out_channels):
super(Decoder, self).__init__()
self.conv1 = ConvBn2d(in_channels, channels, kernel_size=3, padding=1)
self.conv2 = ConvBn2d(channels, out_channels, kernel_size=3, padding=1)
self.spatial_gate = sSE(out_channels)
self.channel_gate = cSE(out_channels)
def forward(self, x, e=None):
x = F.upsample(x, scale_factor=2, mode='bilinear', align_corners=True)
#print('x',x.size())
#print('e',e.size())
if e is not None:
x = torch.cat([x,e],1)
x = F.relu(self.conv1(x),inplace=True)
x = F.relu(self.conv2(x),inplace=True)
#print('x_new',x.size())
g1 = self.spatial_gate(x)
#print('g1',g1.size())
g2 = self.channel_gate(x)
#print('g2',g2.size())
x = g1*x + g2*x
return x
还有一个就是为了进一步鼓励模型在多尺度上的鲁棒性,我们可以引入Hypercolumn去直接把各个scale的feature map concatenate起来:
f = torch.cat((
F.upsample(e1,scale_factor= 2, mode='bilinear',align_corners=False),
d1,
F.upsample(d2,scale_factor= 2, mode='bilinear',align_corners=False),
F.upsample(d3,scale_factor= 4, mode='bilinear',align_corners=False),
F.upsample(d4,scale_factor= 8, mode='bilinear',align_corners=False),
F.upsample(d5,scale_factor=16, mode='bilinear',align_corners=False),
),1)
f = F.dropout2d(f,p=0.50)
logit = self.logit(f)
更神奇的方法就是直接把每个scale的feature map和downsized gt进行比较计算loss,最后各个尺度的loss进行加权平均。详情请见这里的讨论:Deep semi-supervised learning | Kaggle 这里就不再赘述了。
3. Training
其实训练我觉得真的是case by case,在task A上用的heuristics放到task B效果就反而没那么好,所以我就介绍一个大多场合下都能用的trick:Cosine Annealing w. Snapshot Ensemble
听上去听酷炫的,实际上就是每个一段时间warm restart学习率,这样在单位时间内能得到多个而不是一个converged local minina,做融合的话手上的模型会多很多。放几张图上来感受一下:
实现的话,其实挺简单的:CYCLE=8000
LR_INIT=0.1
LR_MIN=0.001
scheduler = lambda x: ((LR_INIT-LR_MIN)/2)*(np.cos(PI*(np.mod(x-1,CYCLE)/(CYCLE)))+1)+LR_MIN
然后每个batch/epoch去用scheduler(iteration)去更新学习率就可以了
4. 其他的一些小tricks(持续更新)
目前能想到的就是DSB2018 第一名的solution。与其是用mask rcnn去做instance segmentation,他们选择了U-Net生成class probability map+watershed小心翼翼分离离得比较近的instances。最后也是取得了领先第二名一截的成绩。不得不说有时候比起研究模型,研究数据并精炼出关键的insight往往能带来更多的收益......
最后安利一下我自己的repo: liaopeiyuan/ml-arsenal-public ,里面会有我所有参与过的Kaggle竞赛的源代码,目前有两个Top 1% solution:TGS Salt和Quick Draw Doodle。欢迎大家提issues/pull requests! XD
你有哪些关于语义分割的实用技巧?欢迎留言与大家交流~
推荐阅读
语义分割汇总
图片语义分割深度学习算法要点回顾
全景分割这一年,端到端之路
微信公众号: 极市平台(ID: extrememart )
每天推送最新CV干货
Recommend
About Joyk
Aggregate valuable and interesting links.
Joyk means Joy of geeK