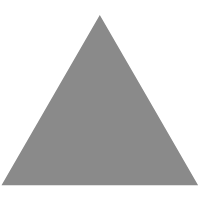
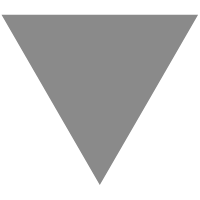
特征工程学习,19项实践Tips!代码已开源!
source link: http://mp.weixin.qq.com/s?__biz=MzIyNjM2MzQyNg%3D%3D&%3Bmid=2247488624&%3Bidx=1&%3Bsn=183efbd2d3bc2f149f1bbfcae5290663
Go to the source link to view the article. You can view the picture content, updated content and better typesetting reading experience. If the link is broken, please click the button below to view the snapshot at that time.
随着我们在机器学习、数据建模、数据挖掘分析这条发展路上越走越远,其实越会感觉到特征工程的重要性,平时我们在很多地方都会看到一些很好的特征工程技巧,但是都会是一个完整项目去阅读,虽然说这样子也可以学习挖掘思路,但有的时候浓缩的技巧总结也是十分重要!
GitHub上有一个专门针对 特征工程技巧 的“锦囊”,叫做 《Tips-of-Feature-engineering》 ,作者把网路上、书本上的一些特征工程的项目,抽取其中的挖掘技巧,并把这些小技巧打包成一个又一个的小锦囊,供大家去检索并且学习,还蛮不错的!
项目地址为:
https://github.com/Pysamlam/Tips-of-Feature-engineering
大家可以先看看目前更新到的内容明细:
项目目前更新到19节,一般来说是每天一更新,每一节都会有配套的数据集以及代码, 下面我们拿几个“锦囊”来看一下!
Tip8:怎么把几个图表一起在同一张图上显示?
这里是使用泰坦尼克号的数据集,前期我们做EDA的时候需要探查数据的分布,从而发现其中的规律,这一节的技巧就是教我们如何画一些常见的图形,同时显示在同一张图上。
关键代码
import matplotlib.pyplot as plt # 设置figure_size尺寸 plt.rcParams['figure.figsize'] = (8.0, 6.0) fig = plt.figure() # 设定图表颜色 fig.set(alpha=0.2) # 第一张小图 plt.subplot2grid((2,3),(0,0)) data_train['Survived'].value_counts().plot(kind='bar') plt.ylabel(u"人数") plt.title(u"船员获救情况 (1为获救)") # 第二张小图 plt.subplot2grid((2,3),(0,1)) data_train['Pclass'].value_counts().plot(kind="bar") plt.ylabel(u"人数") plt.title(u"乘客等级分布") # 第三张小图 plt.subplot2grid((2,3),(0,2)) plt.scatter(data_train['Survived'], data_train['Age']) plt.ylabel(u"年龄") plt.grid(b=True, which='major', axis='y') plt.title(u"按年龄看获救分布 (1为获救)") # 第四张小图,分布图 plt.subplot2grid((2,3),(1,0), colspan=2) data_train.Age[data_train.Pclass == 1].plot(kind='kde') data_train.Age[data_train.Pclass == 2].plot(kind='kde') data_train.Age[data_train.Pclass == 3].plot(kind='kde') plt.xlabel(u"年龄") plt.ylabel(u"密度") plt.title(u"各等级的乘客年龄分布") plt.legend((u'头等舱', u'2等舱',u'3等舱'),loc='best') # 第五张小图 plt.subplot2grid((2,3),(1,2)) data_train.Embarked.value_counts().plot(kind='bar') plt.title(u"各登船口岸上船人数") plt.ylabel(u"人数") plt.show()
我们从上面的可视化操作结果可以看出,其实可以看出一些规律,比如说生还的几率比死亡的要大,然后获救的人在年龄上区别不大,然后就是有钱人(坐头等舱的)的年龄会偏大等。
Tip15:如何使用sklearn的多项式来衍生更多的变量?
关于这种衍生变量的方式,理论其实大家应该很早也都听说过了,但是如何在Python里实现,也就是今天在这里分享给大家,其实也很简单,就是调用 sklearn
的 PolynomialFeatures
方法,具体大家可以看看下面的demo。
这里使用一个人体加速度数据集,也就是记录一个人在做不同动作时候,在不同方向上的加速度,分别有3个方向,命名为x、y、z。
关键代码
# 扩展数值特征 from sklearn.preprocessing import PolynomialFeatures x = df[['x','y','z']] y = df['activity'] poly = PolynomialFeatures(degree=2, include_bias=False, interaction_only=False) x_poly = poly.fit_transform(x) pd.DataFrame(x_poly, columns=poly.get_feature_names()).head()
就这样子简单的去调用,就可以生成了很多的新变量了。
Tip17:如何把分布修正为类正态分布?
今天我们用的是一个新的数据集,也是在kaggle上的一个比赛,大家可以先去下载一下:
下载地址:
https://www.kaggle.com/c/house-prices-advanced-regression-techniques/data
import pandas as pd import numpy as np # Plots import seaborn as sns import matplotlib.pyplot as plt # 读取数据集 train = pd.read_csv('./data/house-prices-advanced-regression-techniques/train.csv') train.head()
首先这个是一个价格预测的题目,在开始前我们需要看看分布情况,可以调用以下的方法来进行绘制:
sns.set_style("white") sns.set_color_codes(palette='deep') f, ax = plt.subplots(figsize=(8, 7)) #Check the new distribution sns.distplot(train['SalePrice'], color="b"); ax.xaxis.grid(False) ax.set(ylabel="Frequency") ax.set(xlabel="SalePrice") ax.set(title="SalePrice distribution") sns.despine(trim=True, left=True) plt.show()
我们从结果可以看出,销售价格是右偏,而大多数机器学习模型都不能很好地处理非正态分布数据,所以我们可以应用log(1+x)转换来进行修正。那么具体我们可以怎么用Python代码实现呢?
# log(1+x) 转换 train["SalePrice_log"] = np.log1p(train["SalePrice"]) sns.set_style("white") sns.set_color_codes(palette='deep') f, ax = plt.subplots(figsize=(8, 7)) sns.distplot(train['SalePrice_log'] , fit=norm, color="b"); # 得到正态分布的参数 (mu, sigma) = norm.fit(train['SalePrice_log']) plt.legend(['Normal dist. ($\mu=$ {:.2f} and $\sigma=$ {:.2f} )'.format(mu, sigma)], loc='best') ax.xaxis.grid(False) ax.set(ylabel="Frequency") ax.set(xlabel="SalePrice") ax.set(title="SalePrice distribution") sns.despine(trim=True, left=True) plt.show()
目前这个项目更新到了19节,但是会持续不断更新“锦囊”,欢迎大家来进行star哦!
项目地址为:
https://github.com/Pysamlam/Tips-of-Feature-engineering
AI学习路线和优质资源,在后台回复"AI"获取
Recommend
About Joyk
Aggregate valuable and interesting links.
Joyk means Joy of geeK