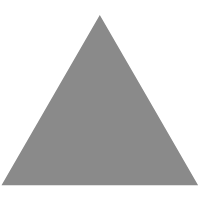
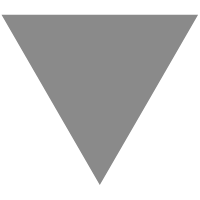
TensorFlow2.0 代码实战专栏(七):循环神经网络示例
source link: http://mp.weixin.qq.com/s?__biz=MzAxMjMwODMyMQ%3D%3D&%3Bmid=2456341528&%3Bidx=1&%3Bsn=cf5c5c332da0204d12710d8a36322a21
Go to the source link to view the article. You can view the picture content, updated content and better typesetting reading experience. If the link is broken, please click the button below to view the snapshot at that time.
作者 | Aymeric Damien
编辑 | 奇予纪
出品 | 磐创AI团队
原项目 | https://github.com/aymericdamien/TensorFlow-Examples/
循环神经网络示例
使用TensorFlow 2.0构建循环神经网络。
RNN 概述

参考文献:
长短时记忆(Long Short Term Memory) [1],Sepp Hochreiter & Jurgen Schmidhuber, Neural Computation 9(8): 1735-1780, 1997.
MNIST 数据集概述
此示例使用手写数字的MNIST数据集。 该数据集包含60,000个用于训练的示例和10,000个用于测试的示例。 这些数字已经过尺寸标准化并位于图像中心,图像是固定大小(28x28像素),值为0到255。 为简单起见,每个图像都被展平并转换为包含784个特征(28*28)的一维numpy数组。

为了使用递归神经网络对图像进行分类,我们将每个图像行都视为像素序列。 由于MNIST的图像形状为28 * 28px,因此我们将为每个样本处理28个时间步长的28个序列。
更多信息请查看链接: http://yann.lecun.com/exdb/mnist/
from __future__ import absolute_import, division, print_function # 导入TensorFlow v2. import tensorflow as tf from tensorflow.keras import Model, layers import numpy as np
# MNIST 数据集参数 num_classes = 10 所有类别(数字 0-9) num_features = 784 # 数据特征 (图像形状: 28*28) # 训练参数 learning_rate = 0.001 training_steps = 1000 batch_size = 32 display_step = 100 # 网络参数 # MNIST的图像形状为28 * 28px,因此我们将为每个样本处理28个时间步长的28个序列。 num_input = 28 # 序列数 timesteps = 28 # 时间步长 num_units = 32 # LSTM层神经元数目
# 准备MNIST数据 from tensorflow.keras.datasets import mnist (x_train, y_train), (x_test, y_test) = mnist.load_data() # 转化为float32 x_train, x_test = np.array(x_train, np.float32), np.array(x_test, np.float32) # 将图像展平为784个特征的一维向量(28*28)。 x_train, x_test = x_train.reshape([-1, 28, 28]), x_test.reshape([-1, num_features]) # 将图像值从[0,255]归一化到[0,1] x_train, x_test = x_train / 255., x_test / 255.
# 使用tf.data API对数据进行随机排序和批处理 train_data = tf.data.Dataset.from_tensor_slices((x_train, y_train)) train_data = train_data.repeat().shuffle(5000).batch(batch_size).prefetch(1)
# 创建LSTM模型 class LSTM(Model): 创建TF模型 def __init__(self): super(LSTM, self).__init__() # RNN (LSTM) 隐含层 self.lstm_layer = layers.LSTM(units=num_units) self.out = layers.Dense(num_classes) # 前向传播 def call(self, x, is_training=False): # LSTM层 x = self.lstm_layer(x) # 输出层 (num_classes). x = self.out(x) if not is_training: # tf 交叉熵接收没有经过softmax的概率输出,所以只有不是训练时才应用softmax x = tf.nn.softmax(x) return x # 创建LSTM模型 lstm_net = LSTM()
# 交叉熵损失 # 注意,这将对概率输出应用'softmax' def cross_entropy_loss(x, y): # 将标签转换为int 64 作为tf交叉熵函数的输入 y = tf.cast(y, tf.int64) # 对概率输出应用softmax并计算交叉熵 loss = tf.nn.sparse_softmax_cross_entropy_with_logits(labels=y, logits=x) # 批中的平均损失 return tf.reduce_mean(loss) # 准确率评估 def accuracy(y_pred, y_true): # 预测类是预测向量(即argmax)分数最高的分量下标 correct_prediction = tf.equal(tf.argmax(y_pred, 1), tf.cast(y_true, tf.int64)) return tf.reduce_mean(tf.cast(correct_prediction, tf.float32), axis=-1) # Adam 优化器 optimizer = tf.optimizers.Adam(learning_rate)
# 优化过程 def run_optimization(x, y): # 将计算封装在GradientTape中以实现自动微分 with tf.GradientTape() as g: # 前向传播 pred = lstm_net(x, is_training=True) # 计算损失 loss = cross_entropy_loss(pred, y) # 要更新的变量,即可训练变量 trainable_variables = lstm_net.trainable_variables # 计算梯度 gradients = g.gradient(loss, trainable_variables) # 按gradients更新 W 和 b optimizer.apply_gradients(zip(gradients, trainable_variables))
# 针对给定步骤数进行训练 for step, (batch_x, batch_y) in enumerate(train_data.take(training_steps), 1): # 运行优化过程以更新W和b值 run_optimization(batch_x, batch_y) if step % display_step == 0: pred = lstm_net(batch_x, is_training=True) loss = cross_entropy_loss(pred, batch_y) acc = accuracy(pred, batch_y) print("step: %i, loss: %f, accuracy: %f" % (step, loss, acc))
output:
step: 100, loss: 1.663173, accuracy: 0.531250 step: 200, loss: 1.034144, accuracy: 0.750000 step: 300, loss: 0.775579, accuracy: 0.781250 step: 400, loss: 0.840327, accuracy: 0.781250 step: 500, loss: 0.344379, accuracy: 0.937500 step: 600, loss: 0.884484, accuracy: 0.718750 step: 700, loss: 0.569674, accuracy: 0.875000 step: 800, loss: 0.401931, accuracy: 0.906250 step: 900, loss: 0.530193, accuracy: 0.812500 step: 1000, loss: 0.265871, accuracy: 0.968750
[1] : http://deeplearning.cs.cmu.edu/pdfs/Hochreiter97_lstm.pdf
还想看更多TensorFlow2.0 专栏文章? 可在公众号底部菜单栏子菜单“独家原创”中找到TensorFlow2.0 系列文章(如下图 ) , 同步更新中,关注公众号了解更多吧~
或点击下方“阅读原文”,进入TensorFlow2.0专栏,即可查看往期文章。
嗨,你还在看吗?
Recommend
About Joyk
Aggregate valuable and interesting links.
Joyk means Joy of geeK