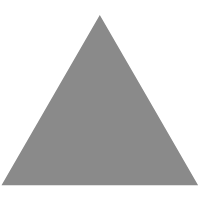
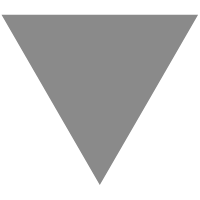
SRCNN-图像超分辨的学习
source link: http://mp.weixin.qq.com/s?__biz=MzIyNjM2MzQyNg%3D%3D&%3Bmid=2247486991&%3Bidx=2&%3Bsn=b4f956b000587279304390e82feab30d
Go to the source link to view the article. You can view the picture content, updated content and better typesetting reading experience. If the link is broken, please click the button below to view the snapshot at that time.
点击上方“ Datawhal e ”,选择“星标 ” 公众号
第一时间获取价值内容
文章摘要
《Learning a Deep Convolutional Network for Image Super-Resolution》的学习。
本文深度学习的方法实现单张图的超分辨,其中深度学习是采用卷积神经网络来实现。这种方法(SRCNN)是一种端到端的作法,输入低分辨率的图像,直接输出高分辨率的图像。本文设计是一种轻量级的神经网络结构,可是它能够实现先进的恢复质量,并且可以快速在线使用。
算法模型
此图展示了SRCNN的网络结构。
此图展示了在卷积神经网络下稀疏编码的结构。
SRCNN整个算法包括三个操作:
1. Patch extraction and representation
2. Non-linear mapping
3. Reconstruction
在该论文中,利用Relu作为收敛函数,利用最小均方差函数为Loss函数。
Loss函数:
TensorFlow代码
// 主函数
from model import SRCNN
from utils import input_setup
import numpy as np
import tensorflow as tf
import pprint
import os
flags = tf.app.flags
flags.DEFINE_integer("epoch", 15000, "Number of epoch [15000]")
flags.DEFINE_integer("batch_size", 128, "The size of batch images [128]")
flags.DEFINE_integer("image_size", 33, "The size of image to use [33]")
flags.DEFINE_integer("label_size", 21, "The size of label to produce [21]")
flags.DEFINE_float("learning_rate", 1e-4, "The learning rate of gradient descent algorithm [1e-4]")
flags.DEFINE_integer("c_dim", 1, "Dimension of image color. [1]")
flags.DEFINE_integer("scale", 3, "The size of scale factor for preprocessing input image [3]")
flags.DEFINE_integer("stride", 14, "The size of stride to apply input image [14]")
flags.DEFINE_string("checkpoint_dir", "checkpoint", "Name of checkpoint directory [checkpoint]")
flags.DEFINE_string("sample_dir", "sample", "Name of sample directory [sample]")
flags.DEFINE_boolean("is_train", True, "True for training, False for testing [True]")
FLAGS = flags.FLAGS
pp = pprint.PrettyPrinter()
def main(_):
pp.pprint(flags.FLAGS.__flags)
if not os.path.exists(FLAGS.checkpoint_dir):
os.makedirs(FLAGS.checkpoint_dir)
if not os.path.exists(FLAGS.sample_dir):
os.makedirs(FLAGS.sample_dir)
with tf.Session() as sess:
srcnn = SRCNN(sess,
image_size=FLAGS.image_size,
label_size=FLAGS.label_size,
batch_size=FLAGS.batch_size,
c_dim=FLAGS.c_dim,
checkpoint_dir=FLAGS.checkpoint_dir,
sample_dir=FLAGS.sample_dir)
srcnn.train(FLAGS)
if __name__ == '__main__':
tf.app.run()
Tensorflow完整代码:
https://github.com/tegg89/SRCNN-Tensorflow结果
双三次差值的 : PSNR=26.633759 dB
SRCNN的: PSNR=29.290147 dB
相比两种算法的PSNR,SRCNN有着明显的提升。
SRCNN的不足
-
利用Relu作为激活函数虽然速度快,但是训练的时候很”脆弱”,很容易就”die”;
-
SRCNN需要先通过双三次插值的方法对低分辨率的图片插值放大尺寸。
参考文献
[1] https://zhuanlan.zhihu.com/p/49846783
[2] https://medium.com/coinmonks/review-srcnn-super-resolution-3cb3a4f67a7c
[3] http://jiaqianlee.com/2018/06/09/SRCNN/
Recommend
About Joyk
Aggregate valuable and interesting links.
Joyk means Joy of geeK