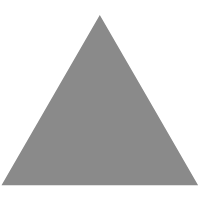
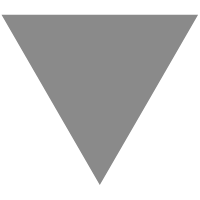
Interpretable Machine Learning for Image Classification with LIME
source link: https://www.tuicool.com/articles/2mueemJ
Go to the source link to view the article. You can view the picture content, updated content and better typesetting reading experience. If the link is broken, please click the button below to view the snapshot at that time.
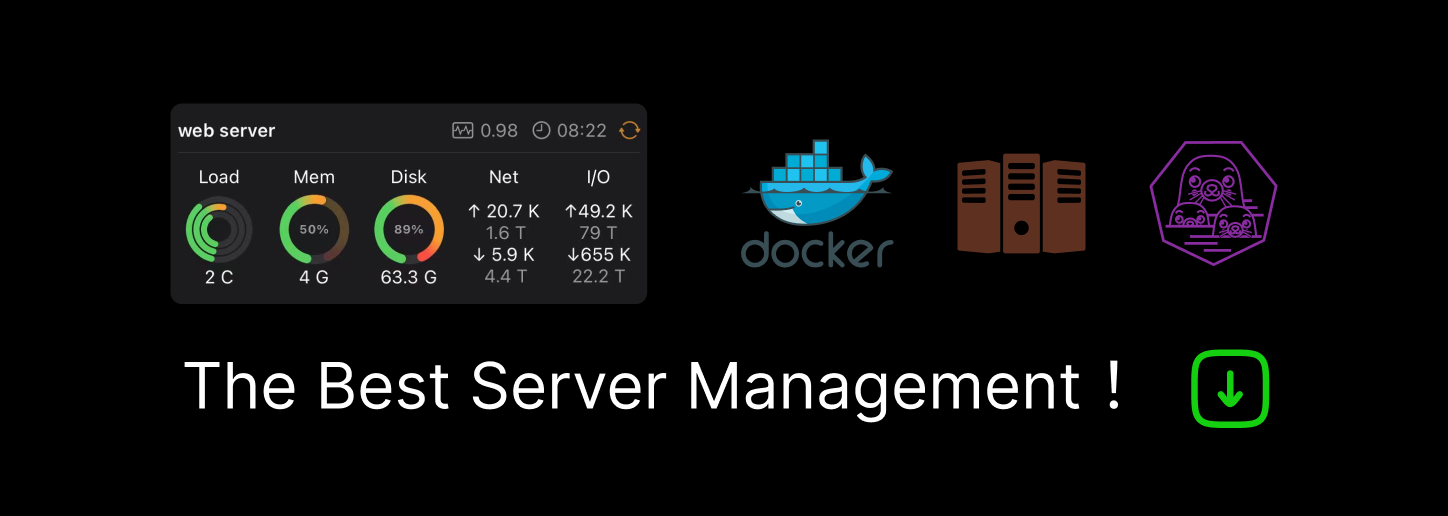
Increase confidence in your machine-learning model by understanding its predictions.
Oct 21 ·5min read
The increasing trend in the use of machine learning for critical applications such as self-driving vehicles and medical diagnosis suggests an imperative need for methodologies that can help to understand and evaluate the predictions of machine-learning models. Local Interpretable Model-agnostic Explanations (LIME)[1] is a technique that provides explanations of how the input features of a machine learning model affect its predictions. For instance, for image classification tasks, LIME finds the region of an image (set of super-pixels) with the strongest association with a prediction label. This post is a step by step guide with Python code on how LIME for image classification internally works.
Let’s start by reading an image and using the pre-trained InceptionV3 model available in Keras to predict the class of such image.
This script loads the input image in the variable Xi
and prints the top 5 classes (and probabilities) for the image as shown below:
- Labrador Retriever (82.2%)
- Golden Retriever (1.5%)
- American Staffordshire Terrier (0.9%)
- Bull Mastiff (0.8%)
- Great Dane (0.7%)
With this information, the input image and the pre-trained InceptionV3 model, we can proceed to generate explanations with LIME. In this example we will generate explanations for the class Labrador Retriever .
LIME Explanations
LIME creates explanations by generating a new dataset of random perturbations (with their respective predictions) around the instance being explained and then fitting a weighted local surrogate model. This local model is usually a simpler model with intrinsic interpretability such as a linear regression model. For more details about the basics behind LIME, I recommend you to check this short tutorial . For the case of image classification, LIME generates explanations with the following steps:
Step 1: Generate random perturbations for input image
For the case of images, LIME generates perturbations by turning on and off some of the super-pixels in the image. The following script uses the quick-shift segmentation algorithm to compute the super-pixels in the image. In addition, it generates an array of 150 perturbations where each perturbation is a vector with zeros and ones that represent whether the super-pixel is on or off.
After computing the super-pixels in the image we get this:
The following are examples of perturbation vectors and perturbed images:
Step 2: Predict class for perturbations
The following script uses the inceptionV3_model
to predict the class of each of the perturbed images. The shape of the predictions
is (150,1000)
which means that for each of the 150 images, we get the probability of belonging to the 1,000 classes in InceptionV3. From these 1,000 classes we will use only the Labrador class in further steps since it is the prediction we want to explain. In this example, 150 perturbations were used. However, for real applications, a larger number of perturbations will produce more reliable explanations.
Now we have everything to fit a linear model using the perturbations
as input features X
and the predictions for Labrador predictions[labrador]
as output y
. However, before we fit a linear model, LIME needs to give more weight (importance) to images that are closer to the image being explained.
Step 3: Compute weights (importance) for the perturbations
We use a distance metric to evaluate how far is each perturbation from the original image. The original image is just a perturbation with all the super-pixels active (all elements in one). Given that the perturbations are multidimensional vectors, the cosine distance is a metric that can be used for this purpose. After the cosine distance has been computed, a kernel function is used to translate such distance to a value between zero and one (a weight). At the end of this process we have a weight (importance) for each perturbation in the dataset.
Step 4: Fit a explainable linear model using the perturbations
, predictions
and weights
We fit a weighted linear model using the information obtained in the previous steps. We get a coefficient for each super-pixel in the image that represents how strong is the effect of the super-pixel in the prediction of Labrador.
We just need to sort these coefficients to determine what are the most important super-pixels ( top_features
)for the prediction of Labrador. Even though here we used the magnitude of the coefficients to determine the most important features, other alternatives such as forward or backward elimination can be used for feature importance selection. After computing the top super-pixels we get:
This is what LIME returns as explanation.The area of the image (super-pixels) that have a stronger association with the prediction of “Labrador Retriever”. This explanation suggests that the pre-trained InceptionV3 model is doing a good job predicting the labrador class for the given image. This example shows how LIME can help to increase confidence in a machine-learning model by understanding why it is returning certain predictions.
A Jupyter Notebook with all the Python code used in this post can be found here . You can easily test explanations on your own images by opening this notebook in Google Colab .
References
[1] Ribeiro, Marco Tulio, Sameer Singh, and Carlos Guestrin. “Why should I trust you? : Explaining the predictions of any classifier.” (2016) Proceedings of the 22nd ACM SIGKDD. ACM.
Recommend
-
38
In machine learning, classification is the task of predicting the class of an object out of a finite number of classes, given some input labeled dataset. For example, you might have a list of 10,000 emails and the need to...
-
51
-
10
Programmatically interpretable reinforcement learning , Verma et al., ICML 2018 Being able to trust (interpret, verify) a controller learned...
-
40
A step-by-step tutorial from data import to accuracy evaluation
-
10
Create an image classification model with ML.NET with Windows Machine Learning
-
6
Discovering Symbolic Models from Deep Learning with Inductive Biases Paper Code
-
3
0:00 / 57:12 ...
-
8
论文标题:Interpretable and Generalizable Graph Learning via Stochastic Attention Mechanism论文作者:Siqi Miao, Mia Liu, Pan Li论文来源:2022,ICML论文地址:d...
-
12
This article was published as a part of the Data Science Blogathon. Introduction In this article, we will train a classification model which uses the fea...
-
3
This article was published as a part of the Data Science Blogathon. Introduction In the 21st century, the world is rapidly moving towards Artificial Inte...
About Joyk
Aggregate valuable and interesting links.
Joyk means Joy of geeK