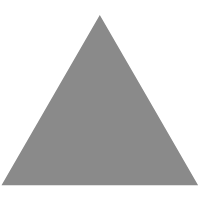
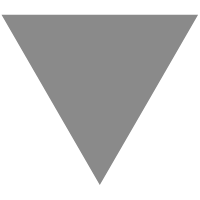
Keras – Hyperparameter Tuning for Humans
source link: https://www.tuicool.com/articles/Zn6bIvf
Go to the source link to view the article. You can view the picture content, updated content and better typesetting reading experience. If the link is broken, please click the button below to view the snapshot at that time.
Keras Tuner
An hyperparameter tuner for Keras
, specifically for tf.keras
with TensorFlow 2.0.
Basic example
Here's how to perform hyperparameter tuning for a single-layer dense neural network using random search.
First, we define a model-building function. It takes an argument hp
from which you can
sample hyperparameters, such as hp.Range('units', min_value=32, max_value=512, step=32)
(an integer from a certain range).
This function returns a compiled model.
from tensorflow import keras from tensorflow.keras import layers from kerastuner.tuners import RandomSearch def build_model(hp): model = keras.Sequential() model.add(layers.Dense(units=hp.Range('units', min_value=32, max_value=512, step=32), activation='relu')) model.add(layers.Dense(10, activation='softmax')) model.compile( optimizer=keras.optimizers.Adam( hp.Choice('learning_rate', values=[1e-2, 1e-3, 1e-4])), loss='sparse_categorical_crossentropy', metrics=['accuracy']) return model
Next, instantiate a tuner. You should specify the model-building function,
the name of the objective to optimize (whether to minimize or maximize is automatically inferred
for built-in metrics), the total number of trials ( max_trials
) to test, and the number
of models that should be built and fit for each trial ( executions_per_trial
).
Available tuners are RandomSearch
and Hyperband
.
Note:the purpose of having multiple executions per trial is to reduce results variance
and therefore be able to more accurately assess the performance of a model. If you want to get
results faster, you could set executions_per_trial=1
(single round of training for each model configuration).
tuner = RandomSearch( build_model, objective='val_accuracy', max_trials=5, executions_per_trial=3, directory='my_dir', project_name='helloworld')
You can print a summary of the search space:
tuner.search_space_summary()
Then, start the search for the best hyperparameter configuration.
The call to search
has the same signature as model.fit()
.
tuner.search(x, y, epochs=5, validation_data=(val_x, val_y))
Finally, retrieve the best model(s):
models = tuner.get_best_models(num_models=2)
Or print a summary of the results:
tuner.results_summary()
Recommend
About Joyk
Aggregate valuable and interesting links.
Joyk means Joy of geeK