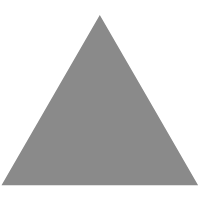
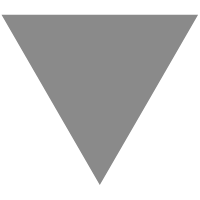
Gradient-Free Optimization for GLMNET Parameters
source link: https://www.tuicool.com/articles/hit/zUJbYnZ
Go to the source link to view the article. You can view the picture content, updated content and better typesetting reading experience. If the link is broken, please click the button below to view the snapshot at that time.
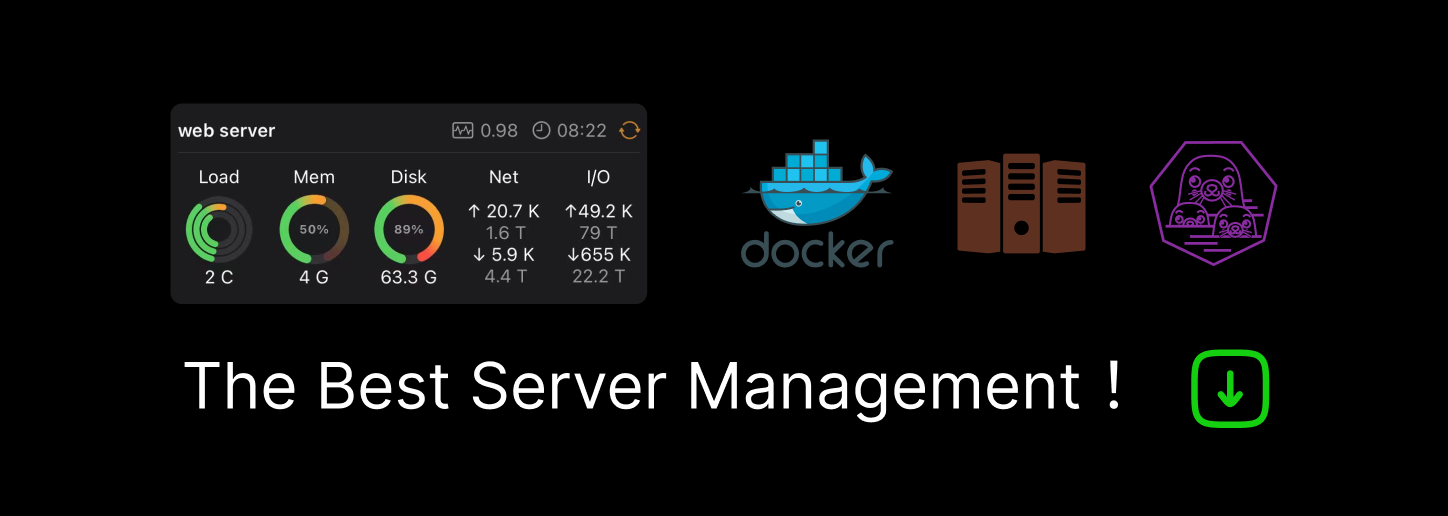
In the post https://statcompute.wordpress.com/2017/09/03/variable-selection-with-elastic-net , it was shown how to optimize hyper-parameters, namely alpha and gamma, of the glmnet by using the built-in cv.glmnet() function. However, following a similar logic of hyper-parameter optimization shown in the post https://statcompute.wordpress.com/2019/02/10/direct-optimization-of-hyper-parameter , we can directly optimize alpha and gamma parameters of the glmnet by using gradient-free optimizations, such as Nelder–Mead simplex or particle swarm. Different from traditional gradient-based optimizations, gradient-free optimizations are often able to find close-to-optimal solutions that are considered “good enough” from an empirical standpoint in many cases that can’t be solved by gradient-based approaches due to noisy and discontinuous functions.
It is very straight-forward to set up the optimization work-flow. All we need to do is writing an objective function, e.g. to maximize the AUC statistic in this specific case, and then maximizing this objective function by calling the optimizer. In the demonstration below, Nelder–Mead simplex and particle swarm optimizers are employed to maximize the AUC statistic defined in the glmnet.optim() function based on a 10-fold cross validation. As shown in the result, both approaches gave very similar outcomes. For whoever interested, please feel free to compare the demonstrated method with the cv.glmnet() function.
Recommend
-
90
Status: Maintenance (expect bug fixes and minor updates) Saving memory using gradient-checkpointing Training very deep neural networks requires a lot of memory. Using the tools in this package, developed jointly by...
-
103
-
95
README.md Pastel ? Gradient animation effect like Instagram
-
85
GitHub is where people build software. More than 27 million people use GitHub to discover, fork, and contribute to over 80 million projects.
-
42
-
31
For optimum performance, a PostgreSQL database depends on the operating system parameters being defined correctly. Poorly configured OS kernel...
-
29
Gradient Descent is an iterative optimization algorithm for finding the (local) minimum of a function. It is one of the most popular techniques in optimization, very commonly used in training neural networks. It is intui...
-
17
A brief yet descriptive guide to Gradient Descent, ADAM, ADAGRAD, RMSProp, NADAM
-
9
-
14
Optimization of multiple parameters with many local minima advertisements I'm looking for algorithms to find a "best" set of parameter values....
About Joyk
Aggregate valuable and interesting links.
Joyk means Joy of geeK