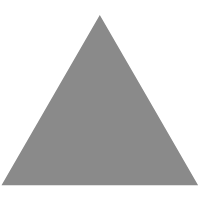
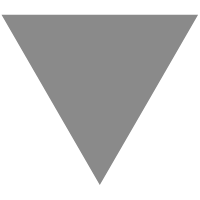
Parameters Optimization Explained
source link: https://towardsdatascience.com/parameters-optimization-explained-876561853de0?gi=fbde5d26f93c
Go to the source link to view the article. You can view the picture content, updated content and better typesetting reading experience. If the link is broken, please click the button below to view the snapshot at that time.
A brief yet descriptive guide to Gradient Descent, ADAM, ADAGRAD, RMSProp, NADAM
May 24 ·8min read
Optimization refers to the task of minimizing/maximizing an objective function f(x) parameterized by x. In machine/deep learning terminology, it’s the task of minimizing the cost/loss function J(w) parameterized by the model’s parameters w ∈ R^d.
Optimization algorithms (in the case of minimization) have one of the following goals:
- Find the global minimum of the objective function. This is feasible if the objective function is convex, i.e. any local minimum is a global minimum.
- Find the lowest possible value of the objective function within its neighborhood. That’s usually the case if the objective function is not convex as the case in most deep learning problems.
Gradient Descent
Gradient Descent is an optimizing algorithm used in Machine/ Deep Learning algorithms. The goal of Gradient Descent is to minimize the objective convex function f(x) using iteration.
Let’s see how Gradient Descent actually works by implementing it on a cost function.
For ease, let’s take a simple linear model.
Error = Y(Predicted)-Y(Actual)
A machine learning model always wants low error with maximum accuracy, in order to decrease error we will intuit our algorithm that you’re doing something wrong that is needed to be rectified, that would be done through Gradient Descent.
We need to minimize our error, in order to get a pointer to minima, we need to walk some steps that are known as alpha(learning rate).
Steps to implement Gradient Descent
- Randomly initialize values.
- Update values.
3. Repeat until slope =0
A derivative is a term that comes from calculus and is calculated as the slope of the graph at a particular point. The slope is described by drawing a tangent line to the graph at the point. So, if we are able to compute this tangent line, we might be able to compute the desired direction to reach the minima.
Learning rate must be chosen wisely as:
1. if it is too small, then the model will take some time to learn.
2. if it is too large, model will converge as our pointer will shoot and we’ll not be able to get to minima.
Vanilla gradient descent, however, does not guarantee good convergence, but offers a few challenges that need to be addressed:
- Choosing a proper learning rate can be difficult. A learning rate that is too small leads to painfully slow convergence, while a learning rate that is too large can hinder convergence and cause the loss function to fluctuate around the minimum or even to diverge.
- Learning rate schedules try to adjust the learning rate during training by e.g. annealing, i.e. reducing the learning rate according to a pre-defined schedule or when the change in objective between epochs falls below a threshold. These schedules and thresholds, however, have to be defined in advance and are thus unable to adapt to a dataset’s characteristics.
- Additionally, the same learning rate applies to all parameter updates. If our data is sparse and our features have very different frequencies, we might not want to update all of them to the same extent, but perform a larger update for rarely occurring features.
- Another key challenge of minimizing highly non-convex error functions common for neural networks is avoiding getting trapped in their numerous suboptimal local minima. The difficulty arises in fact not from local minima but from saddle points, i.e. points where one dimension slopes up and another slopes down. These saddle points are usually surrounded by a plateau of the same error, which makes it notoriously hard for vanilla Gradient Descent to escape, as the gradient is close to zero in all dimensions.
In simple words, every step we take towards minima tends to decrease our slope, now if we visualize, in steep region of curve derivative is going to be large therefore steps taken by our model too would be large but as we will enter gentle region of slope our derivative will decrease and so will the time to reach minima.
To know more about Gradient Descent methods and it’s strategies follow: -
ADAGRAD(Adaptive Gradient Algorithm)
The drawback of Gradient Descent was decay of learning rate due to which our pointer wasn’t able to converge. Now to overcome this drawback we intuit to change learning rate for each and every layer, each and every iteration and each and every neuron as well.
Adagrad eliminates the need to manually tune the learning rate.
The reason to adaptively change learning rate is because there are two types of data :-
- Sparse data
- Dense data
In case of sparse data, we would experience sparse ON(1) features and more frequent OFF(0) features, now, most of the time gradient update will be NULL as derivative is zero in most cases and when it will be one, the steps would be too small to reach minima.
For frequent features we require low learning rate, but for high features we require high learning rate.
So, in order to boost our model for sparse nature data, we need to chose adaptive learning rate.
G(t) is the current step and G(t-1) is the previous step, Epsilon is included as if G(t) becomes zero our learning rate will crash so in order to maintain consistency we use epsilon which denotes a random small positive number.
In the denominator, we accumulate the sum of the square of the past gradients. Each term is a positive term so it keeps on growing to make the learning rate η infinitesimally small to the point that algorithm is no longer able learning.
The tag line for ADAGRAD is Fewer Updates, Lesser decay.
If you’re familiar with working of Gradient Descent, you must’ve noticed with every step taken towards minima our learning rate shortens which leads to its decay and more time consumption, that’s fixed using ADAGRAD.
Advantages:-
Parameters corresponding to sparse features gets better updates.
Disadvantages:-
Learning rate decays very aggressively as denominator grows.
RMSProp
The main aim for RMSProp is to prevent overly aggressive behavior of G(t)in ADAGRAD. Now if we go deep in ADAGRAD we can comprehend that G(t) is exploding as we are collectively aggregating square of past gradient from first iteration to last iteration.
To escape blasting of G(t) we intend to average the decay in order to make process more smooth and less time consuming.
Even if our G(t) increases, it will be diminished to small quantity as we are computing its product by (1-Б2) which is itself very small thus cancelling its high effect.
Here, the learning rate is decreasing but not as fast as in case of ADAGRAD thus making convergence more fluid with less time consumption.
In nutshell, Rmsprop is a very clever way to deal with the problem. It uses a moving average of squared gradients to normalize the gradient itself. That has an effect of balancing the step size, decrease the step for large gradient to avoid exploding, and increase the step for small gradient to avoid vanishing.
ADAM
The algorithms leverages the power of adaptive learning rates methods to find individual learning rates for each parameter. It also has advantages of Adagrad, which works really well in settings with sparse gradients, but struggles in non-convex optimization of neural networks, and RMSprop, which tackles to resolve some of the problems of Adagrad and works really well in on-line settings.
Adam can be looked at as a combination of RMSprop and Stochastic Gradient Descent with momentum. It uses the squared gradients to scale the learning rate like RMSprop and it takes advantage of momentum by using moving average of the gradient instead of gradient itself like SGD with momentum.
Adam algorithm first updates the exponential moving averages of the gradient(mt) and the squared gradient(vt) which is the estimates of the first and second moment.
Hyper-parameters β1, β2 ∈ [0, 1) control the exponential decay rates of these moving averages as shown below
Moving averages are initialized as 0 leading to moment estimates that are biased around 0 especially during the initial timesteps. This initialization bias can be easily counteracted resulting in bias-corrected estimates
Finally, we update the parameter as shown below

Adam implements the exponential moving average of the gradients to scale the learning rate instead of a simple average as in Adagrad. It keeps an exponentially decaying average of past gradients.
Adam is computationally efficient and has very little memory requirement.
NADAM(Nesterov-accelerated Adaptive Moment Estimation)
- NADAM combines NAG and Adam
- NADAM is employed for noisy gradients or for gradients with high curvatures
- The learning process is accelerated by summing up the exponential decay of the moving averages for the previous and current gradient
Conclusion
Hopefully, this article has increased your understanding of optimization techniques.
As always, thank you so much for reading, and please share this article if you found it useful! :)
Recommend
About Joyk
Aggregate valuable and interesting links.
Joyk means Joy of geeK