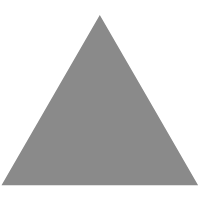
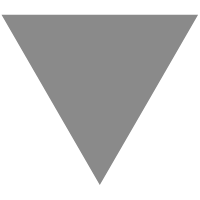
GitHub - facebookresearch/fastMRI: A large-scale dataset of both raw MRI measure...
source link: https://github.com/facebookresearch/fastMRI
Go to the source link to view the article. You can view the picture content, updated content and better typesetting reading experience. If the link is broken, please click the button below to view the snapshot at that time.
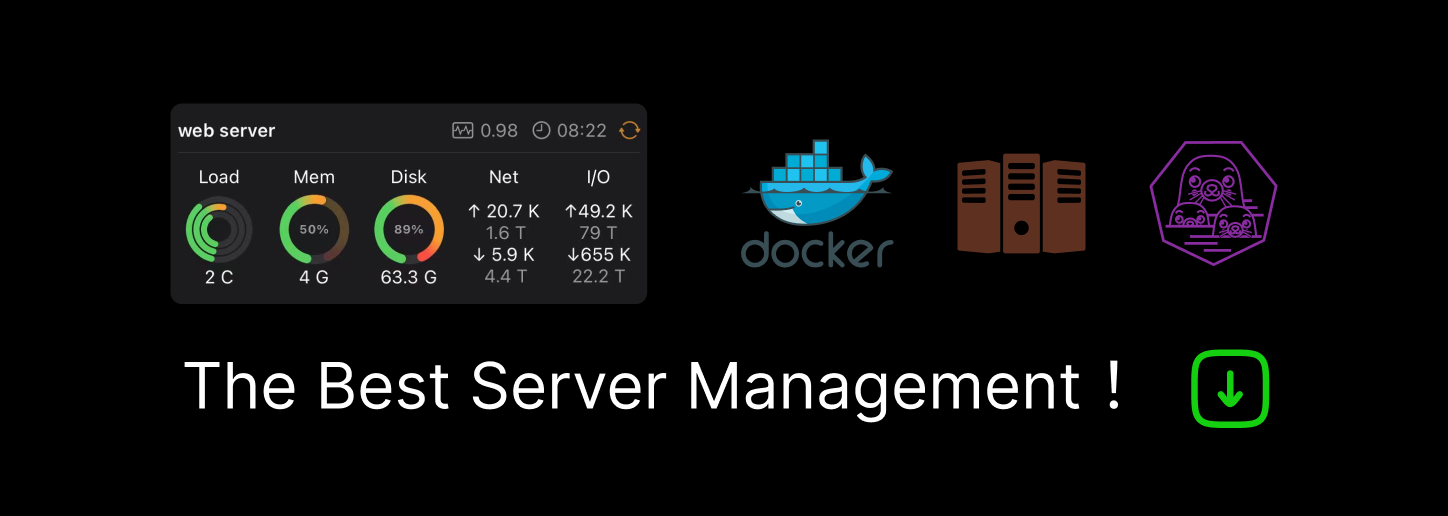
README.md
fastMRI
Accelerating Magnetic Resonance Imaging (MRI) by acquiring fewer measurements has the potential to reduce medical costs, minimize stress to patients and make MR imaging possible in applications where it is currently prohibitively slow or expensive.
fastMRI is collaborative research project from Facebook AI Research (FAIR) and NYU Langone Health to investigate the use of AI to make MRI scans faster. NYU Langone Health has released fully anonymized Knee MRI datasets that can be downloaded from the fastMRI dataset page.
This repository contains convenient PyTorch data loaders, subsampling functions, evaluation metrics, and reference implementations of simple baseline methods.
Citing
If you use the fastMRI data or this code in your research, please consider citing the fastMRI dataset paper:
@inproceedings{zbontar2018fastMRI,
title={fastMRI: An Open Dataset and Benchmarks for Accelerated MRI},
author={Jure Zbontar and Florian Knoll and Anuroop Sriram et. al.},
journal = {ArXiv e-prints},
archivePrefix = "arXiv",
eprint = {1811.08839},
year={2018}
}
Dependencies
We have tested this code using:
- Ubuntu 18.04
- Python 3.6
- CUDA 9.0
- CUDNN 7.0
You can find the full list of Python packages needed to run the code in the
requirements.txt
file. These can be installed using:
pip install -r requirements.txt
Directory Structure & Usage
common
: Contains several utility functions and classes that can be used to create subsampling masks, evaluate results and create submission files.data
: Contains PyTorch data loaders for loading the fastMRI data and PyTorch data transforms useful for working with MRI data. Seedata/README.md
for more information about using the data loaders.models
: Contains the baseline models.
Testing
Run pytest
.
Submitting to Leaderboard
Run your model on the provided test data and create a zip file containing your predictions. Upload this to any publicly accessible cloud storage (e.g. Amazon S3, Dropbox etc) and then create a JSON file with your information.
The common/utils.py
file has some convenience functions to help with this:
save_reconstructions
function saves the data in the correct format.create_submission_file
creates a JSON file for submission.
Submit the JSON file on the EvalAI page.
License
fastMRI is MIT licensed, as found in the LICENSE file.
Recommend
-
30
Highly complex services, such as those here at Facebook, have large source code bases in order to deliver a wide range of features and functionality. Even after the machine code for one of these services is compiled, it...
-
59
The aim of this piece was to produce a piece of3D art that's ready to go straight from the frame buffer, with little or no post-production. Zagreb-based Studio Niskota (now Polyma...
-
39
README.md QMNIST The exact preprocessing steps used to construct the MNIST dataset have long been lost....
-
11
Exploratory Data Analysis on a large dataset using ROOT.
-
9
March 25, 2021 Engineering How GitHub Actions renders large-scale logs
-
13
CO3D: Common Objects In 3D This repository contains a set of tools for working with the Common Objects in 3D (CO3D) dataset. The dataset has been introduced in our ICCV'21 paper: Comm...
-
9
How would you weigh an elephant without using a scale? If we are not allowed to use a weighing machine then what are our options? Well, there aren’t very many. It helps to think of natural things of which...
-
13
SAHI: Slicing Aided Hyper Inference A lightweight vision library for performing large scale object detection & instance segmentation Overview Object detection and instance segme...
-
9
SentinelOne launches DataSet to manage live data at scale Did you miss a session at the Data Summit? Watch On-Demand
-
9
Fine tune LLAMA3 on million scale dataset in consumer GPU using QLora, Deepspeed8 min read4 hours agoHighlights,
About Joyk
Aggregate valuable and interesting links.
Joyk means Joy of geeK