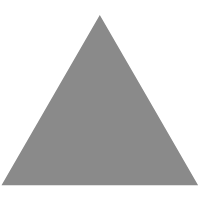
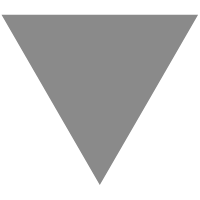
GitHub - google/uis-rnn: This is the library for the Unbounded Interleaved-State...
source link: https://github.com/google/uis-rnn
Go to the source link to view the article. You can view the picture content, updated content and better typesetting reading experience. If the link is broken, please click the button below to view the snapshot at that time.
README.md
UIS-RNN
Overview
This is the library for the Unbounded Interleaved-State Recurrent Neural Network (UIS-RNN) algorithm. UIS-RNN solves the problem of segmenting and clustering sequential data by learning from examples.
This algorithm was originally proposed in the paper Fully Supervised Speaker Diarization.
Disclaimer
This open source implementation is slightly different than the internal one which we used to produce the results in the paper, due to dependencies on some internal libraries.
This is NOT an official Google product.
Dependencies
This library depends on:
- python 3.5+
- numpy 1.15.1
- pytorch 0.4.0
Tutorial
Run the demo
To get started, simply run this command:
python3 demo.py --train_iteration=20000
This will train a UIS-RNN model using data/training_data.npz
,
then store the model on disk, perform inference on data/testing_data.npz
,
print the inference results, and save the approximate accuracy in a text file.
Core APIs
All algorithms are implemented as the UISRNN
class. First, construct a
UISRNN
object by:
model = UISRNN(args)
Next, train the model by calling the fit()
function:
model.fit(train_sequence, train_cluster_id, args)
Once we are done with the training, we can run the trained model to perform
inference on new sequences by calling the predict()
function:
predicted_label = model.predict(test_sequence, args)
The definitions of the args are described in model/arguments.py
.
Run the tests
You can also verify the correctness of this library by running:
sh run_tests.sh
If you fork this library and make local changes, be sure to use these tests as a sanity check. Besides, these tests are also great examples for learning the APIs.
Citations
Our paper is cited as:
@article{zhang2018fully,
title={Fully Supervised Speaker Diarization},
author={Zhang, Aonan and Wang, Quan and Zhu, Zhenyao and Paisley, John and Wang, Chong},
journal={arXiv preprint arXiv:1810.04719},
year={2018}
}
Recommend
About Joyk
Aggregate valuable and interesting links.
Joyk means Joy of geeK