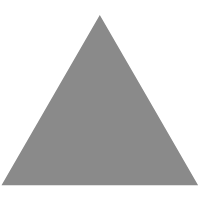
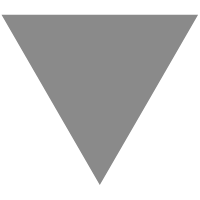
基于Kmeans算法的文档聚类(包含Java代码及数据格式)
source link: http://blog.csdn.net/qy20115549/article/details/80530117?amp%3Butm_medium=referral
Go to the source link to view the article. You can view the picture content, updated content and better typesetting reading experience. If the link is broken, please click the button below to view the snapshot at that time.
本文作者:合肥工业大学 管理学院 钱洋 email:[email protected] 内容可能有不到之处,欢迎交流。
未经本人允许禁止转载。
介绍
给定多篇文档,如何对文档进行聚类。本博客使用的是k-means聚类方法。关于k-means网络上有很多资料介绍其算法思想和其数学公式。
针对文档聚类,首先要讲文档进行向量化,也就是说要对文档进行编码。可以使用one-hot编码,也可以使用TF-IDF编码,也可以使用doc2vec编码等,总之,要将其向量化。
本人最近做文本分类时,使用的一个baseline就是k-means文档聚类。其借鉴的源码地址为: https://github.com/Hazoom/documents-k-means
在该源码基础上做了改进。
输入数据结构

该输入文本的第一列为文本的标题,第二列是经过去高频词、停用词、低频词之后的数据。
源码
首先,我修改的是文档的表示,因为我的数据和作者的json数据并不同。
package com.clustering; import java.io.BufferedReader; import java.io.File; import java.io.FileInputStream; import java.io.IOException; import java.io.InputStreamReader; import java.util.ArrayList; import java.util.Collections; import java.util.Iterator; import java.util.List; import java.util.StringTokenizer; /** Class for storing a collection of documents to be clustered. */ public class DocumentList implements Iterable<Document> { private final List<Document> documents = new ArrayList<Document>(); private int numFeatures; /** Construct an empty DocumentList. */ public DocumentList() { } /** * Construct a DocumentList by parsing the input string. The input string may contain multiple * document records. Each record must be delimited by curly braces {}. */ /*public DocumentList(String input) { StringTokenizer st = new StringTokenizer(input, "{"); int numDocuments = st.countTokens() - 1; String record = st.nextToken(); // skip empty split to left of { for (int i = 0; i < numDocuments; i++) { record = st.nextToken(); Document document = Document.createDocument(record); if (document != null) { documents.add(document); } } }*/ public DocumentList(String input) throws IOException { BufferedReader reader = new BufferedReader( new InputStreamReader( new FileInputStream( new File(input)),"gbk")); String s = null; int i = 0; while ((s=reader.readLine())!=null) { String arry[] =s.split("\t"); String content = s.substring(arry[0].length()).trim(); String title =arry[0]; Document document = new Document(i, content, title); documents.add(document); i++; } reader.close(); } /** Add a document to the DocumentList. */ public void add(Document document) { documents.add(document); } /** Clear all documents from the DocumentList. */ public void clear() { documents.clear(); } /** Mark all documents as not being allocated to a cluster. */ public void clearIsAllocated() { for (Document document : documents) { document.clearIsAllocated(); } } /** Get a particular document from the DocumentList. */ public Document get(int index) { return documents.get(index); } /** Get the number of features used to encode each document. */ public int getNumFeatures() { return numFeatures; } /** Determine whether DocumentList is empty. */ public boolean isEmpty() { return documents.isEmpty(); } @Override public Iterator<Document> iterator() { return documents.iterator(); } /** Set the number of features used to encode each document. */ public void setNumFeatures(int numFeatures) { this.numFeatures = numFeatures; } /** Get the number of documents within the DocumentList. */ public int size() { return documents.size(); } /** Sort the documents within the DocumentList by document ID. */ public void sort() { Collections.sort(documents); } @Override public String toString() { StringBuilder sb = new StringBuilder(); for (Document document : documents) { sb.append(" "); sb.append(document.toString()); sb.append("\n"); } return sb.toString(); } }
其次,针对KMeansClusterer,我们做了如下修改,因为我想要自定义k,而源码作者提供了自动调节k值的方法。
package com.clustering; import java.util.Random; /** A Clusterer implementation based on k-means clustering. */ public class KMeansClusterer implements Clusterer { private static final Random RANDOM = new Random(); private final double clusteringThreshold; private final int clusteringIterations; private final DistanceMetric distance; /** * Construct a Clusterer. * * @param distance the distance metric to use for clustering * @param clusteringThreshold the threshold used to determine the number of clusters k * @param clusteringIterations the number of iterations to use in k-means clustering */ public KMeansClusterer(DistanceMetric distance, double clusteringThreshold, int clusteringIterations) { this.distance = distance; this.clusteringThreshold = clusteringThreshold; this.clusteringIterations = clusteringIterations; } /** * Allocate any unallocated documents in the provided DocumentList to the nearest cluster in the * provided ClusterList. */ private void allocatedUnallocatedDocuments(DocumentList documentList, ClusterList clusterList) { for (Document document : documentList) { if (!document.isAllocated()) { Cluster nearestCluster = clusterList.findNearestCluster(distance, document); nearestCluster.add(document); } } } /** * Run k-means clustering on the provided documentList. Number of clusters k is set to the lowest * value that ensures the intracluster to intercluster distance ratio is below * clusteringThreshold. */ @Override public ClusterList cluster(DocumentList documentList) { ClusterList clusterList = null; for (int k = 1; k <= documentList.size(); k++) { clusterList = runKMeansClustering(documentList, k); if (clusterList.calcIntraInterDistanceRatio(distance) < clusteringThreshold) { break; } } return clusterList; } /** Create a cluster with the unallocated document that is furthest from the existing clusters. */ private Cluster createClusterFromFurthestDocument(DocumentList documentList, ClusterList clusterList) { Document furthestDocument = clusterList.findFurthestDocument(distance, documentList); Cluster nextCluster = new Cluster(furthestDocument); return nextCluster; } /** Create a cluster with a single randomly seelcted document from the provided DocumentList. */ private Cluster createClusterWithRandomlySelectedDocument(DocumentList documentList) { int rndDocIndex = RANDOM.nextInt(documentList.size()); Cluster initialCluster = new Cluster(documentList.get(rndDocIndex)); return initialCluster; } /** Run k means clustering on the provided DocumentList for a fixed number of clusters k. */ public ClusterList runKMeansClustering(DocumentList documentList, int k) { ClusterList clusterList = new ClusterList(); documentList.clearIsAllocated(); clusterList.add(createClusterWithRandomlySelectedDocument(documentList)); while (clusterList.size() < k) { clusterList.add(createClusterFromFurthestDocument(documentList, clusterList)); } for (int iter = 0; iter < clusteringIterations; iter++) { allocatedUnallocatedDocuments(documentList, clusterList); clusterList.updateCentroids(); if (iter < clusteringIterations - 1) { clusterList.clear(); } } return clusterList; } }
package com.clustering; /** * An interface defining a Clusterer. A Clusterer groups documents into Clusters based on similarity * of their content. */ public interface Clusterer { /** Cluster the provided list of documents. */ public ClusterList cluster(DocumentList documentList); public ClusterList runKMeansClustering(DocumentList documentList, int k); }
针对接口Clusterer ,其包含两类实现方法,其一是自动确定k数目的方法;其二是用户自定义k值的方法。
结果输出部分
该部分,是自己写的一个类,用于输出聚类结果,以及类单词出现的概率(这里直接计算的是单词在该类中的频率),可自行定义输出topk个单词。具体代码如下:
package com.clustering; import java.io.BufferedWriter; import java.io.File; import java.io.FileOutputStream; import java.io.IOException; import java.io.OutputStreamWriter; import java.util.ArrayList; import java.util.Collections; import java.util.Comparator; import java.util.Hashtable; import java.util.List; import java.util.Map; import java.util.Map.Entry; public class OutPutFile { public static void outputdocument(String strDir,ClusterList clusterList) throws IOException{ BufferedWriter Writer = new BufferedWriter( new OutputStreamWriter( new FileOutputStream( new File(strDir)),"gbk")); for (Cluster cluster : clusterList) { // System.out.println(cluster1.getDocuments()); String text = ""; for (Document doc: cluster.getDocuments()) { text +=doc.getContents()+" "; } Writer.write(text+"\n"); } Writer.close(); } public static void outputcluster(String strDir,ClusterList clusterList) throws IOException{ BufferedWriter Writer = new BufferedWriter( new OutputStreamWriter( new FileOutputStream( new File(strDir)),"gbk")); Writer.write(clusterList.toString()); Writer.close(); } public static void outputclusterwprdpro(String strDir,ClusterList clusterList,int topword) throws IOException{ BufferedWriter Writer = new BufferedWriter( new OutputStreamWriter( new FileOutputStream( new File(strDir)),"gbk")); Hashtable<Integer,String> clusterdocumentlist = new Hashtable<Integer,String>(); int clusterid=0; for (Cluster cluster : clusterList) { String text = ""; for (Document doc: cluster.getDocuments()) { text +=doc.getContents()+" "; } clusterdocumentlist.put(clusterid,text); clusterid++; } for (Integer key : clusterdocumentlist.keySet()) { Writer.write("Topic" + new Integer(key) + "\n"); List<Entry<String, Double>> list=oneclusterwprdpro(clusterdocumentlist.get(key)); int count=0; for (Map.Entry<String, Double> mapping : list) { if (count<=topword) { Writer.write("\t" + mapping.getKey() + " " + mapping.getValue()+ "\n"); count++; }else { break; } } } Writer.close(); } //词频统计并排序 public static List<Entry<String, Double>> oneclusterwprdpro(String text){ Hashtable<String, Integer> wordCount = new Hashtable<String, Integer>(); String arry[] =text.split("\\s+"); //词频统计 for (int i = 0; i < arry.length; i++) { if (!wordCount.containsKey(arry[i])) { wordCount.put(arry[i], Integer.valueOf(1)); } else { wordCount.put(arry[i], Integer.valueOf(wordCount.get(arry[i]).intValue() + 1)); } } //频率计算 Hashtable<String, Double> wordpro = new Hashtable<String, Double>(); for (java.util.Map.Entry<String, Integer> j : wordCount.entrySet()) { String key = j.getKey(); double value = 1.0*j.getValue()/arry.length; wordpro.put(key, value); } //将map.entrySet()转换成list List<Map.Entry<String, Double>> list = new ArrayList<Map.Entry<String, Double>>(wordpro.entrySet()); Collections.sort(list, new Comparator<Map.Entry<String, Double>>() { //降序排序 public int compare(Entry<String, Double> o1, Entry<String, Double> o2) { //return o1.getValue().compareTo(o2.getValue()); return o2.getValue().compareTo(o1.getValue()); } }); return list; } }
主方法
package web.main; import java.io.IOException; import com.clustering.ClusterList; import com.clustering.Clusterer; import com.clustering.CosineDistance; import com.clustering.DistanceMetric; import com.clustering.DocumentList; import com.clustering.Encoder; import com.clustering.KMeansClusterer; import com.clustering.OutPutFile; import com.clustering.TfIdfEncoder; /** * Solution for Newsle Clustering question from CodeSprint 2012. This class implements clustering of * text documents using Cosine or Jaccard distance between the feature vectors of the documents * together with k means clustering. The number of clusters is adapted so that the ratio of the * intracluster to intercluster distance is below a specified threshold. */ public class ClusterDocumentsArgs { private static final int CLUSTERING_ITERATIONS = 30; private static final double CLUSTERING_THRESHOLD = 0.5; private static final int NUM_FEATURES =10000; private static final int k = 30; //自行定义k /** * Cluster the text documents in the provided file. The clustering process consists of parsing and * encoding documents, and then using Clusterer with a specific Distance measure. */ public static void main(String[] args) throws IOException { String fileinput = "/home/qianyang/kmeans/webdata/content"; DocumentList documentList = new DocumentList(fileinput); Encoder encoder = new TfIdfEncoder(NUM_FEATURES); encoder.encode(documentList); System.out.println(documentList.size()); DistanceMetric distance = new CosineDistance(); Clusterer clusterer = new KMeansClusterer(distance, CLUSTERING_THRESHOLD, CLUSTERING_ITERATIONS); ClusterList clusterList = clusterer.runKMeansClustering(documentList, k); // ClusterList clusterList = clusterer.cluster(documentList); //输出聚类结果 OutPutFile.outputcluster("/home/qianyang/kmeans/result/cluster"+k,clusterList); //输出topk个单词 OutPutFile.outputclusterwprdpro("/home/qianyang/kmeans/result/wordpro"+k+"and10", clusterList, 10); OutPutFile.outputclusterwprdpro("/home/qianyang/kmeans/result/wordpro"+k+"and15", clusterList, 15); OutPutFile.outputclusterwprdpro("/home/qianyang/kmeans/result/wordpro"+k+"and20", clusterList, 20); OutPutFile.outputclusterwprdpro("/home/qianyang/kmeans/result/wordpro"+k+"and25", clusterList, 25); } }
如下图所示为结果,我们可以看出每个簇下面的所聚集的文档有哪些。

如下图所示为簇下单词的频率。

Recommend
About Joyk
Aggregate valuable and interesting links.
Joyk means Joy of geeK