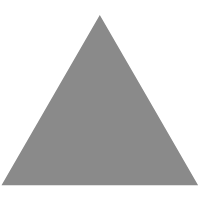
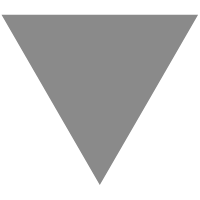
GitHub - TheHedgeify/DagstuhlGAN
source link: https://github.com/TheHedgeify/DagstuhlGAN
Go to the source link to view the article. You can view the picture content, updated content and better typesetting reading experience. If the link is broken, please click the button below to view the snapshot at that time.
README.md
MarioGAN
This project allows for the unsupervised learning of a Generative Adversarial Network (GAN) that understands the structure of Super Mario Bros. levels. The model is trained on actual Mario levels from the Video Game Level Corpus. The trained model is capable of generating new level segments with the input of a latent vector, and these segments can be stitched together to make complete levels. In order to find the best level segments within this latent space, the evolutionary algorithm Covariance Matrix Adaptation Evolution Strategy (CMA-ES) is used to find latent vectors producing level segments that either optimize some sort of tile distribution or result in a particular level of performance by an artificial agent. The resulting system helps discover new levels in the space of examples created by human experts.
For more information, please see the following publication. This publication should also be cited if code from this project is used in any way:
@inproceedings{volz:gecco2018,
title={Evolving Mario Levels in the Latent Space of a Deep Convolutional Generative Adversarial Network},
author={Volz, Vanessa and Schrum, Jacob and Liu, Jialin and Lucas, Simon M. and Smith, Adam M. and Risi, Sebastian},
year={2018},
booktitle={Proceedings of the Genetic and Evolutionary Computation Conference (GECCO 2018)},
month={July},
numpages = {8},
url = {http://doi.acm.org/10.1145/3205455.3205517},
doi = {10.1145/3205455.3205517},
publisher = {ACM},
address = {New York, NY, USA},
location={Kyoto, Japan}
}
Installing
TODO: How to setup Pytorch? Sebastian?
python -m pip install cma
Using the Code
There are two separate aspects to this codebase:
- The GAN code written in Python and trained with Pytorch
- The Mario and CMA-ES code written in Java
Training the GAN
An already trained Pytorch model is part of this repository. It is in pytorch/netG_epoch_5000.pth. However, if you would like to re-train the GAN yourself from scratch here is how you would do it.
TODO: Ask Sebastian.
Evolving Levels Based on Static Features
The trained GAN model can be used to generate Mario levels that optimize certain tile distributions.
TODO: Ask Sebastian where these files are and how to run them.
Evolving Levels Based on Agent Performance
The trained GAN model can also be used to evolve levels based on how a Java-based agent performs in them. This approach uses the Java version of CMA-ES, though the Java code still executes the Python Pytorch model. The Java class to execute is cmatest.CMAMarioSolver. This code will evaluate the levels by playing them with Robin Baumgarten's A* Agent that won the 2009 Mario AI Competition.
The level is specified by a latent vector of modifiable size. The values need to be between -1 and 1 and should otherwise be mapped to that value range. To generate an image of the generated level, the class to execute is cmatest.MarioLevelViewer. To do this, the class cmatest.MarioEvalFunction has the function levelFromLatentVector that returns a level, which can be played by an agent (human or artifical) via the BasicSimulator ch.idsia.mario.simulation.BasicSimulator.
Alternatively, the level can created from a .json file (as produced by the GAN) that describes the level with nested arrays and encodes the different tiles available according to the video level corpus. To do this, use function marioLevelsFromJson in cmatest.MarioEvalFunction
Recommend
About Joyk
Aggregate valuable and interesting links.
Joyk means Joy of geeK