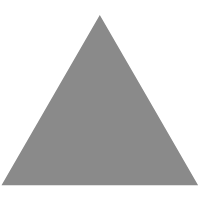
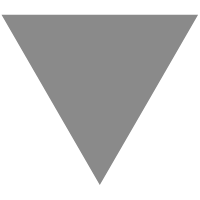
Generating entropy without imports in Go
source link: https://blog.merovius.de/2018/01/15/generating_entropy_without_imports_in_go.html
Go to the source link to view the article. You can view the picture content, updated content and better typesetting reading experience. If the link is broken, please click the button below to view the snapshot at that time.
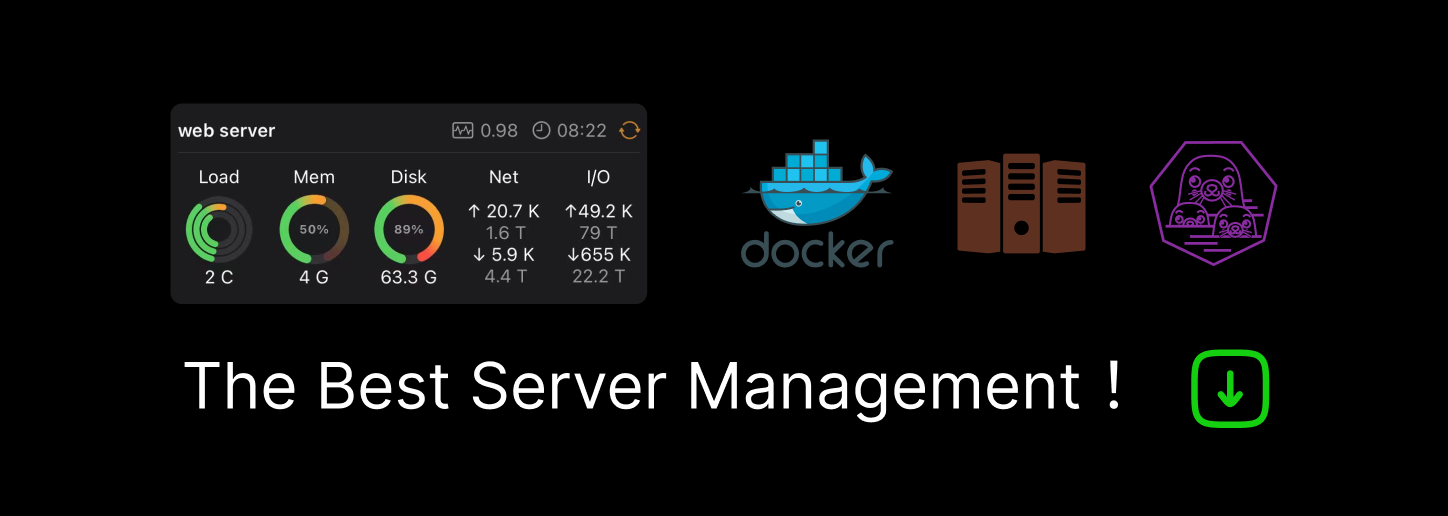
Generating entropy without imports in Go
tl;dr: I come up with a couple of useless, but entertaining ways to generate entropy without relying on any packages.
This post is inspired by a comment on reddit, saying
[…]given the constraints of no imports and the function signature:
func F(map[string]string) map[string]string { ... }
F must use a deterministic algorithm, since it is a deterministic algorithm it can be represented in a finite state machine.
Now, the point of this comment was to talk about how to then compile such a function into a deterministic finite state machine, but it got me thinking about a somewhat different question. If we disallow any imports and assume a standard (gc) Go implementation - how many ways can we find to create a non-deterministic function?
So, the challenge I set to myself was: Write a function func() string
that a)
can not refer to any qualified identifier (i.e. no imports) and b) is
non-deterministic, that is, produces different outputs on each run. To start me
off, I did add a couple of helpers, to accumulate entropy, generate random
numbers from it and to format strings as hex, without any imports:
type rand uint32
func (r *rand) mix(v uint32) {
*r = ((*r << 5) + *r) + rand(v)
}
func (r *rand) rand() uint32 {
mx := rand(int32(*r)>>31) & 0xa8888eef
*r = *r<<1 ^ mx
return uint32(*r)
}
func hex(v uint32) string {
var b []byte
for v != 0 {
if x := byte(v & 0xf); x < 10 {
b = append(b, '0'+x)
} else {
b = append(b, 'a'+x-10)
}
v >>= 4
}
return string(b)
}
Obviously, these could be inlined, but separating them allows us to reuse them for our different functions. Then I set about the actual task at hand.
Method 1: Map iteration#
In Go, the iteration order of maps is not specified:
The iteration order over maps is not specified and is not guaranteed to be the same from one iteration to the next.
But gc
, the canonical Go implementation, actively
randomizes the map iteration order to
prevent programs from depending on it. We can use this, to receive some of
entropy from the runtime, by creating a map and iterating over it:
func MapIteration() string {
var r rand
m := make(map[uint32]bool)
for i := uint32(0); i < 100; i++ {
m[i] = true
}
for i := 0; i < 1000; i++ {
for k := range m {
r.mix(k)
break // the rest of the loop is deterministic
}
}
return hex(r.rand())
}
We first create a map with a bunch of keys. We then iterate over it a bunch of times; each map iteration gives us a different start index, which we mix into our entropy pool.
Method 2: Select#
Go actually defines a way in which the runtime is giving us access to entropy directly:
If one or more of the communications can proceed, a single one that can proceed is chosen via a uniform pseudo-random selection.
So the spec guarantees that if we have multiple possible communications in a select, the case has to be chosen non-deterministically. We can, again, extract that non-determinism:
func Select() string {
var r rand
ch := make(chan bool)
close(ch)
for i := 0; i < 1000; i++ {
select {
case <-ch:
r.mix(1)
case <-ch:
r.mix(2)
}
}
return hex(r.rand())
}
We create a channel and immediately close it. We then create a select-statement with two cases and depending on which was taken, we mix a different value into our entropy pool. The channel is closed, to guarantee that communication can always proceed. This way, we extract one bit of entropy per iteration.
Note, that there is no racing or concurrency involved here: This is simple, single-threaded Go code. The randomness comes directly from the runtime. Thus, this should work in any compliant Go implementation. The playground, however, is not compliant with the spec in this regard, strictly speaking. It is deliberately deterministic.
Method 3: Race condition#
This method exploits the fact, that on a multi-core machine at least, the Go scheduler is non-deterministic. So, if we let two goroutines race to write a value to a channel, we can extract some entropy from which one wins this race:
func RaceCondition() string {
var r rand
for i := 0; i < 1000; i++ {
ch := make(chan uint32, 2)
start := make(chan bool)
go func() {
<-start
ch <- 1
}()
go func() {
<-start
ch <- 2
}()
close(start)
r.mix(<-ch)
}
return hex(r.rand())
}
The start
channel is there to make sure that both goroutines become runnable
concurrently. Otherwise, the first goroutine would be relatively likely to
write the value before the second is even spawned.
Method 4: Allocation/data races#
Another thought I had, was to try to extract some entropy from the allocator or GC. The basic idea is, that the address of an allocated value might be non-deterministic - in particular, if we allocate a lot. We can then try use that as entropy.
However, I could not make this work very well, for the simple reason that Go does not allow you to actually do anything with pointers - except dereferencing and comparing them for equality. So while you might get non-deterministic values, those values can’t be used to actually generate random numbers.
I thought I might be able to somehow get a string or integer representation of some pointer without any imports. One way I considered was inducing a runtime-panic and recovering that, in the hope that the error string would contain a stacktrace or offending values. However, none of the error strings created by the runtime actually seem to contain any values that could be used here.
I also tried a workaround to interpret the pointer as an integer, by exploiting race conditions to do unsafe operations:
func DataRace() string {
var r rand
var data *uintptr
var addr *uintptr
var i, j, k interface{}
i = (*uintptr)(nil)
j = &data
done := false
go func() {
for !done {
k = i
k = j
}
}()
for {
if p, ok := k.(*uintptr); ok && p != nil {
addr = p
done = true
break
}
}
data = new(uintptr)
r.mix(uint32(*addr))
return hex(r.rand())
}
It turns out, however, that at least this particular instance of a data race has been fixed since Russ Cox wrote that blog post. In Go 1.9, this code just loops endlessly. I tried it in Go 1.5, though, and it works there - but we don’t get a whole lot of entropy (addresses are not that random). With other methods, we could re-run the code to collect more entropy, but in this case, I believe the escape analysis gets into our way by stack-allocating the pointer, so it will be the same one on each run.
I like this method, because it uses several obscure steps to work, but on the other hand, it’s the least reliable and it requires an old Go version.
Your Methods?#
These are all the methods I could think of; but I’m sure I missed a couple. If you can think of any, feel free to let me know on Twitter, reddit or hackernews :) I also posted the code in a gist, so you can download and run it yourself, but keep in mind, that the last method busy-loops in newer Go versions.
Recommend
-
53
Information theory is a subfield of mathematics concerned with transmitting data across a noisy channel. A cornerstone of information theory is the idea of quantifying how much information there is in a message....
-
32
Cross-entropy is commonly used in machine learning as a loss function. Cross-entropy is a measure from the field of information theory, building upon entropy and generally calculating the difference between two p...
-
34
Detection of malware is a constant battle between the technologies designed to detect and prevent malware and the authors creating them. One common technique adversaries leverage is packing binaries. Packing an executable...
-
13
[][][ Thread Prev ][ Thread Next ][
-
21
Use Information Theory to understand NLP Metrics Ravi Cha...
-
20
Implementation of the Cross-Entropy Training Loop Jordi...
-
13
Defining Entropy Entropy is a measure of chaos, or disorder, in a system. My college physics professor described entropy using two shoe closets. Imagine a clean shoe closet, where all shoes are pa...
-
14
As the first digital computers were being invented in the 1940s and researchers like Alan Turing were formalizing their capabilities, the scientific and engineering communities needed a way to quantify previous intuitive...
-
10
What Is Entropy In Cryptography? February 14, 2020 ...
-
10
When We first made our own implementation of URL Imports for Ree.js we learnt a simple hack from here...
About Joyk
Aggregate valuable and interesting links.
Joyk means Joy of geeK