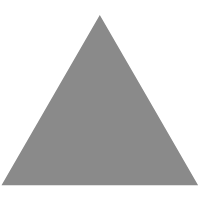
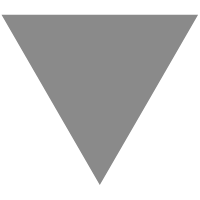
Apache Flink:Keyed Window与Non-keyed Window
source link: http://shiyanjun.cn/archives/1775.html?amp%3Butm_medium=referral
Go to the source link to view the article. You can view the picture content, updated content and better typesetting reading experience. If the link is broken, please click the button below to view the snapshot at that time.
Apache Flink:Keyed Window与Non-Keyed Window
Apache Flink中,Window操作在流式数据处理中是非常核心的一种抽象,它把一个无限流数据集分割成一个个有界的Window(或称为Bucket),然后就可以非常方便地定义作用于Window之上的各种计算操作。本文我们主要基于Apache Flink 1.4.0版本,说明Keyed Window与Non-Keyed Window的基本概念,然后分别对与其相关的WindowFunction与WindowAllFunction的类设计进行分析,最后通过编程实践来应用。
基本概念
Flink将Window分为两类,一类叫做Keyed Window,另一类叫做Non-Keyed Window。为了说明这两类Window的不同,我们看下Flink官网给出的,基于这两种类型的Window编写代码的结构说明。
基于Keyed Window进行编程,用户代码基本结构如下所示:
stream .keyBy(...) <- keyed versus Non-Keyed windows .window(...) <- required: "assigner" [.trigger(...)] <- optional: "trigger" (else default trigger) [.evictor(...)] <- optional: "evictor" (else no evictor) [.allowedLateness(...)] <- optional: "lateness" (else zero) [.sideOutputLateData(...)] <- optional: "output tag" (else no side output for late data) .reduce/aggregate/fold/apply() <- required: "function" [.getSideOutput(...)] <- optional: "output tag" |
基于Non-Keyed Window进行编程,用户代码基本结构如下所示:
stream .windowAll(...) <- required: "assigner" [.trigger(...)] <- optional: "trigger" (else default trigger) [.evictor(...)] <- optional: "evictor" (else no evictor) [.allowedLateness(...)] <- optional: "lateness" (else zero) [.sideOutputLateData(...)] <- optional: "output tag" (else no side output for late data) .reduce/aggregate/fold/apply() <- required: "function" [.getSideOutput(...)] <- optional: "output tag" |
上面两种编程结构的区别在于:
从编程API上看,Keyed Window编程结构,可以直接对输入的stream按照Key进行操作,输入的stream中识别Key,即输入stream中的每个数据元素哪一部分是作为Key来关联这个数据元素的,这样就可以对stream中的数据元素基于Key进行相关计算操作,如keyBy,可以根据Key进行分组(相同的Key必然可以分到同一组中去)。如果输入的stream中没有Key,比如就是一条日志记录信息,那么无法对其进行keyBy操作。而对于Non-Keyed Window编程结构来说,无论输入的stream具有何种结构(比如是否具有Key),它都认为是无结构的,不能对其进行keyBy操作,而且如果使用Non-Keyed Window函数操作,就会对该stream进行分组(具体如何分组依赖于我们选择的WindowAssigner,它负责将stream中的每个数据元素指派到一个或多个Window中),指派到一个或多个Window中,然后后续应用到该stream上的计算都是对Window中的这些数据元素进行操作。
从计算上看,Keyed Window编程结构会将输入的stream转换成Keyed stream,逻辑上会对应多个Keyed stream,每个Keyed stream会独立进行计算,这就使得多个Task可以对Windowing操作进行并行处理,具有相同Key的数据元素会被发到同一个Task中进行处理。而对于Non-Keyed Window编程结构,Non-Keyed stream逻辑上将不能split成多个stream,所有的Windowing操作逻辑只能在一个Task中进行处理,也就是说计算并行度为1。
在实际编程过程中,我们可以看到DataStream的API也有对应的方法timeWindow()和timeWindowAll(),他们也分别对应着Keyed Window和Non-Keyed Window。
WindowFunction与AllWindowFunction
Flink中对输入stream进行Windowing操作后,将到达的数据元素指派到指定的Window中,或者基于EventTime/ProcessingTime,或者基于Count,或者混合EventTime/ProcessingTime/Count,来对数据元素进行分组。那么,在对分配的Window进行操作时,就需要使用Flink提供的函数(Function),而对于Window的操作,分别基于Keyed Window、Non-Keyed Window提供了WindowFunction、AllWindowFunction,通过实现特定的Window函数,能够访问Window相关的元数据,来满足实际应用需要。下面,我们从类设计的角度,来看下对应的继承层次结构:
- Keyed Window对应的WindowFunction
Keyed Window对应的WindowFunction类图,如下所示:

/** * Base abstract class for functions that are evaluated over keyed (grouped) * windows using a context for retrieving extra information. * * @tparam IN The type of the input value. * @tparam OUT The type of the output value. * @tparam KEY The type of the key. * @tparam W The type of the window. */ @ PublicEvolving abstract class ProcessWindowFunction[IN, OUT, KEY, W < : Window] extends AbstractRichFunction |
对Keyed stream的Window进行操作,上面泛型对应4个类型参数:
IN表示进入到该ProcessWindowFunction的数据元素的类型,例如stream中上一个操作的输出是包含两个String类型的元组,则IN类型对应为(String, String);
OUT表示该ProcessWindowFunction处理后的输出数据元素的类型,例如输出一个String和一个Long的元组,则OUT类型对应为(String, Long);
KEY有一点不同,需要注意,它并不是面向应用编程用户使用的,而且该值不会提供有意义的业务应用含义,在Keyed Window中它是用来跟踪该Window的,一般应用开发中只需要将其作为输出的Key即可,后面我们会有对应的编程实践;
W类型表示该ProcessWindowFunction作用的Window的类型,例如TimeWindow、GlobalWindow。
下面,我们看一下继承自ProcessWindowFunction需要实现的方法,方法签名如下所示:
/** * Evaluates the window and outputs none or several elements. * * @param key The key for which this window is evaluated. * @param context The context in which the window is being evaluated. * @param elements The elements in the window being evaluated. * @param out A collector for emitting elements. * @throws Exception The function may throw exceptions to fail the program and trigger recovery. */ @ throws[Exception] def process(key : KEY, context : Context, elements : Iterable[IN], out : Collector[OUT]) |
进入到该Window,对应着其中一个Keyed stream。属于某个Window的数据元素都在elements这个集合中,我们可以对这些数据元素进行处理。通过context可以访问Window对应的元数据信息,比如TimeWindow的开始时间(start)和结束时间(end)。out是一个Collector,负责收集处理后的数据元素并发送到stream下游进行处理。
- Non-Keyed Window对应的AllWindowFunction
Non-Keyed Window对应的WindowFunction类图,如下所示:

/** * Base abstract class for functions that are evaluated over keyed (grouped) * windows using a context for retrieving extra information. * * @tparam IN The type of the input value. * @tparam OUT The type of the output value. * @tparam W The type of the window. */ @ PublicEvolving abstract class ProcessAllWindowFunction[IN, OUT, W < : Window] extends AbstractRichFunction |
可以同ProcessWindowFunction对比一下,发现ProcessAllWindowFunction的泛型参数中没有了用来跟踪Window的KEY,因为Non-Keyed Window只在一个Task中进行处理,其它的OUT和W与前面ProcessWindowFunction类相同,不再累述。
继承自ProcessAllWindowFunction,需要实现的方法,如下所示:
/** * Evaluates the window and outputs none or several elements. * * @param context The context in which the window is being evaluated. * @param elements The elements in the window being evaluated. * @param out A collector for emitting elements. * @throws Exception The function may throw exceptions to fail the program and trigger recovery. */ @ throws[Exception] def process(context : Context, elements : Iterable[IN], out : Collector[OUT]) |
该ProcessAllWindowFunction作用于原始输入的stream,所有的数据元素经过Windowing后,都会经过该方法进行处理,在该方法具体处理逻辑与ProcessWindowFunction.process()类似。
编程实践
现在,我们模拟这样一个场景:某个App开发商需要从多个渠道(Channel)推广App,需要通过日志来分析对应的用户行为(安装、打开、浏览、点击、购买、关闭、卸载),我们假设要实时(近实时)统计分析每个时间段内(如每隔5秒)来自不同渠道的用户的行为。
首先,创建一个模拟生成数据的SourceFunction,实现代码如下所示:
class SimulatedEventSource extends RichParallelSourceFunction[(String, String)] { val LOG = LoggerFactory.getLogger(classOf[SimulatedEventSource]) @ volatile private var running = true val channelSet = Seq( "a" , "b" , "c" , "d" ) val behaviorTypes = Seq( "INSTALL" , "OPEN" , "BROWSE" , "CLICK" , "PURCHASE" , "CLOSE" , "UNINSTALL" ) val rand = Random override def run(ctx : SourceContext[(String, String)]) : Unit = { val numElements = Long.MaxValue var count = 0 L while (running && count < numElements) { val channel = channelSet(rand.nextInt(channelSet.size)) val event = generateEvent() LOG.info( "Event: " + event) val ts = event( 0 ).toLong ctx.collectWithTimestamp((channel, event.mkString( "\t" )), ts) count + = 1 TimeUnit.MILLISECONDS.sleep( 5 L) } } private def generateEvent() : Seq[String] = { val dt = readableDate val id = UUID.randomUUID().toString val behaviorType = behaviorTypes(rand.nextInt(behaviorTypes.size)) // (ts, readableDT, id, behaviorType) Seq(dt. _ 1 .toString, dt. _ 2 , id, behaviorType) } private def readableDate = { val df = new SimpleDateFormat( "yyyy-MM-dd HH:mm:ss" ) val ts = System.nanoTime val dt = new Date(ts) (ts, df.format(dt)) } override def cancel() : Unit = running = false } |
有了该数据源,我们就可以基于该SimulatedEventSource来构建Flink Streaming应用程序了。下面,也分别面向Keyed Window和Non-Keyed Window来编程实践,并比较它们不同之处。
- Keyed Window编程
我们基于Sliding Window(WindowAssigner)来在stream上生成Window,Window大小size=5s,silde=1s,即每个Window计算5s之内的数据元素,每个1s启动一个Window(查看提交该Flink程序的命令行中指定的各个参数值)。同时,基于上面自定义实现的SimulatedEventSource作为输入数据源,创建Flink stream,然后后续就可以对stream进行各种操作了。
处理stream数据,我们希望能够获取到每个Window对应的起始时间和结束时间,然后输出基于Window(起始时间+结束时间)、渠道(Channel)、行为类型进行分组统计的结果,最后将结果数据实时写入到指定Kafka topic中。
我们实现的Flink程序类为SlidingWindowAnalytics,代码如下所示:
def main(args : Array[String]) : Unit = { val params = ParameterTool.fromArgs(args) checkParams(params) val windowSizeMillis = params.getRequired( "window-size-millis" ).toLong val windowSlideMillis = params.getRequired( "window-slide-millis" ).toLong val env = StreamExecutionEnvironment.getExecutionEnvironment env.setStreamTimeCharacteristic(TimeCharacteristic.ProcessingTime) val stream : DataStream[(String, String)] = env.addSource( new SimulatedEventSource) // create a Kafka producer for Kafka 0.9.x val kafkaProducer = new FlinkKafkaProducer 09 ( params.getRequired( "window-result-topic" ), new SimpleStringSchema, params.getProperties ) stream .map(t = > { val channel = t. _ 1 val eventFields = t. _ 2 .split( "\t" ) val behaviorType = eventFields( 3 ) ((channel, behaviorType), 1 L) }) .keyBy( 0 ) .timeWindow(Time.of(windowSizeMillis, MILLISECONDS), Time.of(windowSlideMillis, MILLISECONDS)) .process( new MyReduceWindowFunction) .map(t = > { val key = t. _ 1 val count = t. _ 2 val windowStartTime = key. _ 1 val windowEndTime = key. _ 2 val channel = key. _ 3 val behaviorType = key. _ 4 Seq(windowStartTime, windowEndTime, channel, behaviorType, count).mkString( "\t" ) }) .addSink(kafkaProducer) env.execute(getClass.getSimpleName) } |
首先,对输入stream进行一个map操作,处理输出 ((渠道, 行为类型), 计数)。
其次,基于该结果进行一个keyBy操作,指定Key为(渠道, 行为类型),得到了多个Keyed stream。
接着,对每个Keyed stream应用Sliding Window操作,设置Sliding Window的size和slide值。
然后,因为我们想要获取到Window对应的起始时间和结束时间,所以需要对Windowing后的stream进行一个ProcessWindowFunction操作,这个是我们自定义实现的,在其中获取到Window起始时间和结束时间,并对Windowing的数据进行分组统计(groupBy),然后输出带有Window起始时间和结束时间,以及渠道、行为类型、统计计数这些信息,对应的实现类为MyReduceWindowFunction,代码如下所示:
class MyReduceWindowFunction extends ProcessWindowFunction[((String, String), Long), ((String, String, String, String), Long), Tuple, TimeWindow] { override def process(key : Tuple, context : Context, elements : Iterable[((String, String), Long)], collector : Collector[((String, String, String, String), Long)]) : Unit = { val startTs = context.window.getStart val endTs = context.window.getEnd for (group <- elements.groupBy( _ . _ 1 )) { val myKey = group. _ 1 val myValue = group. _ 2 var count = 0 L for (elem <- myValue) { count + = elem. _ 2 } val channel = myKey. _ 1 val behaviorType = myKey. _ 2 val outputKey = (formatTs(startTs), formatTs(endTs), channel, behaviorType) collector.collect((outputKey, count)) } } private def formatTs(ts : Long) = { val df = new SimpleDateFormat( "yyyyMMddHHmmss" ) df.format( new Date(ts)) } } |
上面对应于ProcessWindowFunction的泛型参数的值,分别为:IN=((String, String), Long)、OUT=((String, String, String, String), Long)、KEY=Tuple、W=TimeWindow,这样可以对照方法process()中的各个参数的类型来理解。上述代码中,elements中可能存在多个相同的Key的值,但是具有同一个Key的数据元素一定会在同一个Window中(即elements),我们需要对elements进行一个groupBy的内存计算操作,再对每个group中的数据进行汇总计数,输出为((Window开始时间, Window结束时间, 渠道, 行为类型), 累加计数值)。这样,即可有调用stream上的process方法,将该MyReduceWindowFunction实现的示例作为参数值传进去即可。
最后,通过map操作将结果格式化,输出保存到Kafka中。
运行上面我们实现的Flink程序,执行如下命令:
bin /flink run --parallelism 2 --class org.shirdrn.flink.windowing.SlidingWindowAnalytics flink-demo-assembly-0.0.1-SNAPSHOT.jar \ --window-result-topic windowed-result-topic \ --zookeeper.connect 172.16.117.63:2181,172.16.117.64:2181,172.16.117.65:2181 \ --bootstrap.servers ali-bj01-tst-cluster-002.xiweiai.cn:9092,ali-bj01-tst-cluster-003.xiweiai.cn:9092,ali-bj01-tst-cluster-004.xiweiai.cn:9092 \ --window-size-millis 5000 \ --window-slide-millis 1000 |
提交运行后,可以通过Flink Web Dashboard查看Job运行状态。可以在Kafka中查看最终结果数据,对应的输出数据示例如下所示:
20180106174726 20180106174731 b CLOSE 69 20180106174726 20180106174731 b UNINSTALL 86 20180106174726 20180106174731 a CLICK 64 20180106174726 20180106174731 a PURCHASE 72 20180106174727 20180106174732 b BROWSE 61 20180106174727 20180106174732 d INSTALL 67 20180106174727 20180106174732 c CLICK 74 20180106174727 20180106174732 c INSTALL 61 20180106174727 20180106174732 c PURCHASE 66 20180106174728 20180106174733 c CLICK 79 20180106174728 20180106174733 a BROWSE 58 20180106174728 20180106174733 a UNINSTALL 73 20180106174728 20180106174733 c OPEN 68 20180106174728 20180106174733 d INSTALL 55 20180106174728 20180106174733 b INSTALL 60 20180106174728 20180106174733 c PURCHASE 64 20180106174728 20180106174733 b PURCHASE 78 20180106174728 20180106174733 d UNINSTALL 58 20180106174728 20180106174733 d BROWSE 69 |
通过结果可以看到,采用Sliding Window来指派Window,随着时间流逝各个Window之间存在重叠的现象,这正是我们最初想要的结果。
- Non-Keyed Window编程
这里,我们基于Tumbling Window(WindowAssigner)来在stream上生成Non-Keyed Window。Tumbling Window也被称为固定时间窗口(Fixed Time Window),各个Window的时间长度相同,Window之间没有重叠。
我们想要达到的目标和前面类似,也希望获取到每个Window对应的起始时间和结束时间,所以需要实现一个ProcessWindowAllFunction,但因为是Non-Keyed Window,只有一个Task来负责对所有输入stream中的数据元素指派Window,这在编程实现中并没有感觉到有太大的差异。实现的Flink程序为TumblingWindowAllAnalytics,代码如下所示:
object TumblingWindowAllAnalytics { var MAX _ LAGGED _ TIME = 5000 L def checkParams(params : ParameterTool) = { if (params.getNumberOfParameters < 5 ) { println( "Missing parameters!\n" + "Usage: Windowing " + "--window-result-topic <windowed_result_topic> " + "--bootstrap.servers <kafka_brokers> " + "--zookeeper.connect <zk_quorum> " + "--window-all-lagged-millis <window_all_lagged_millis> " + "--window-all-size-millis <window_all_size_millis>" ) System.exit(- 1 ) } } def main(args : Array[String]) : Unit = { val params = ParameterTool.fromArgs(args) checkParams(params) MAX _ LAGGED _ TIME = params.getLong( "window-all-lagged-millis" , MAX _ LAGGED _ TIME) val windowAllSizeMillis = params.getRequired( "window-all-size-millis" ).toLong val env = StreamExecutionEnvironment.getExecutionEnvironment env.setStreamTimeCharacteristic(TimeCharacteristic.ProcessingTime) val stream : DataStream[(String, String)] = env.addSource( new SimulatedEventSource) // create a Kafka producer for Kafka 0.9.x val kafkaProducer = new FlinkKafkaProducer 09 ( params.getRequired( "window-result-topic" ), new SimpleStringSchema, params.getProperties ) stream .map(t = > { val channel = t. _ 1 val eventFields = t. _ 2 .split( "\t" ) val ts = eventFields( 0 ).toLong val behaviorType = eventFields( 3 ) (ts, channel, behaviorType) }) .assignTimestampsAndWatermarks( new TimestampExtractor(MAX _ LAGGED _ TIME)) .map(t = > (t. _ 2 , t. _ 3 )) .timeWindowAll(Time.milliseconds(windowAllSizeMillis)) .process( new MyReduceWindowAllFunction()) .map(t = > { val key = t. _ 1 val count = t. _ 2 val windowStartTime = key. _ 1 val windowEndTime = key. _ 2 val channel = key. _ 3 val behaviorType = key. _ 4 Seq(windowStartTime, windowEndTime, channel, behaviorType, count).mkString( "\t" ) }) .addSink(kafkaProducer) env.execute(getClass.getSimpleName) } class TimestampExtractor( val maxLaggedTime : Long) extends AssignerWithPeriodicWatermarks[(Long, String, String)] with Serializable { var currentWatermarkTs = 0 L override def getCurrentWatermark : Watermark = { if (currentWatermarkTs < = 0 ) { new Watermark(Long.MinValue) } else { new Watermark(currentWatermarkTs - maxLaggedTime) } } override def extractTimestamp(element : (Long, String, String), previousElementTimestamp : Long) : Long = { val ts = element. _ 1 Math.max(ts, currentWatermarkTs) } } } |
上面代码中,我们在输入stream开始处理时,调用DataStream的assignTimestampsAndWatermarks方法为stream中的每个数据元素指派时间戳,周期性地生成WaterMark来控制stream的处理进度(Progress),用来提取时间戳和生成WaterMark的实现参考实现类TimestampExtractor。有关WaterMark相关的内容,可以参考后面的参考链接中给出的介绍。
另外,我们实现了Flink的ProcessWindowAllFunction抽象类,对应实现类为MyReduceWindowAllFunction,用来处理每个Window中的数据,获取对应的Window的起始时间和结束时间,实现代码如下所示:
class MyReduceWindowAllFunction extends ProcessAllWindowFunction[(String, String), ((String, String, String, String), Long), TimeWindow] { override def process(context : Context, elements : Iterable[(String, String)], collector : Collector[((String, String, String, String), Long)]) : Unit = { val startTs = context.window.getStart val endTs = context.window.getEnd val elems = elements.map(t = > { ((t. _ 1 , t. _ 2 ), 1 L) }) for (group <- elems.groupBy( _ . _ 1 )) { val myKey = group. _ 1 val myValue = group. _ 2 var count = 0 L for (elem <- myValue) { count + = elem. _ 2 } val channel = myKey. _ 1 val behaviorType = myKey. _ 2 val outputKey = (formatTs(startTs), formatTs(endTs), channel, behaviorType) collector.collect((outputKey, count)) } } private def formatTs(ts : Long) = { val df = new SimpleDateFormat( "yyyyMMddHHmmss" ) df.format( new Date(ts)) } } |
与Keyed Window实现中的ProcessWindowFunction相比,这里没有了对应的泛型参数KEY,因为这种情况下只有一个Task处理stream输入的所有数据元素,ProcessAllWindowFunction的实现类对所有未进行groupBy(也无法进行,因为数据元素的Key未知)操作得到的Window中的数据元素进行处理,处理逻辑和前面基本相同。
提交Flink程序TumblingWindowAllAnalytics,执行如下命令行:
bin /flink run --parallelism 2 --class org.shirdrn.flink.windowing.TumblingWindowAllAnalytics flink-demo-assembly-0.0.1-SNAPSHOT.jar \ --window-result-topic windowed-result-topic \ --zookeeper.connect 172.16.117.63:2181,172.16.117.64:2181,172.16.117.65:2181 \ --bootstrap.servers ali-bj01-tst-cluster-002.xiweiai.cn:9092,ali-bj01-tst-cluster-003.xiweiai.cn:9092,ali-bj01-tst-cluster-004.xiweiai.cn:9092 \ --window-all-lagged-millis 3000 \ --window-all-size-millis 10000 |
成功运行,可以看到输出结果,和前面类似。
参考链接
本文基于署名-非商业性使用-相同方式共享 4.0许可协议发布,欢迎转载、使用、重新发布,但务必保留文章署名时延军(包含链接:http://shiyanjun.cn),不得用于商业目的,基于本文修改后的作品务必以相同的许可发布。如有任何疑问,请与我联系。
Post navigation
Recommend
About Joyk
Aggregate valuable and interesting links.
Joyk means Joy of geeK