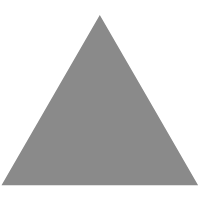
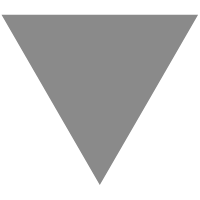
通过ORPO技术微调 llama3大模型(Fine-tune Llama 3 with ORPO) - aehyok
source link: https://www.cnblogs.com/aehyok/p/18151963
Go to the source link to view the article. You can view the picture content, updated content and better typesetting reading experience. If the link is broken, please click the button below to view the snapshot at that time.
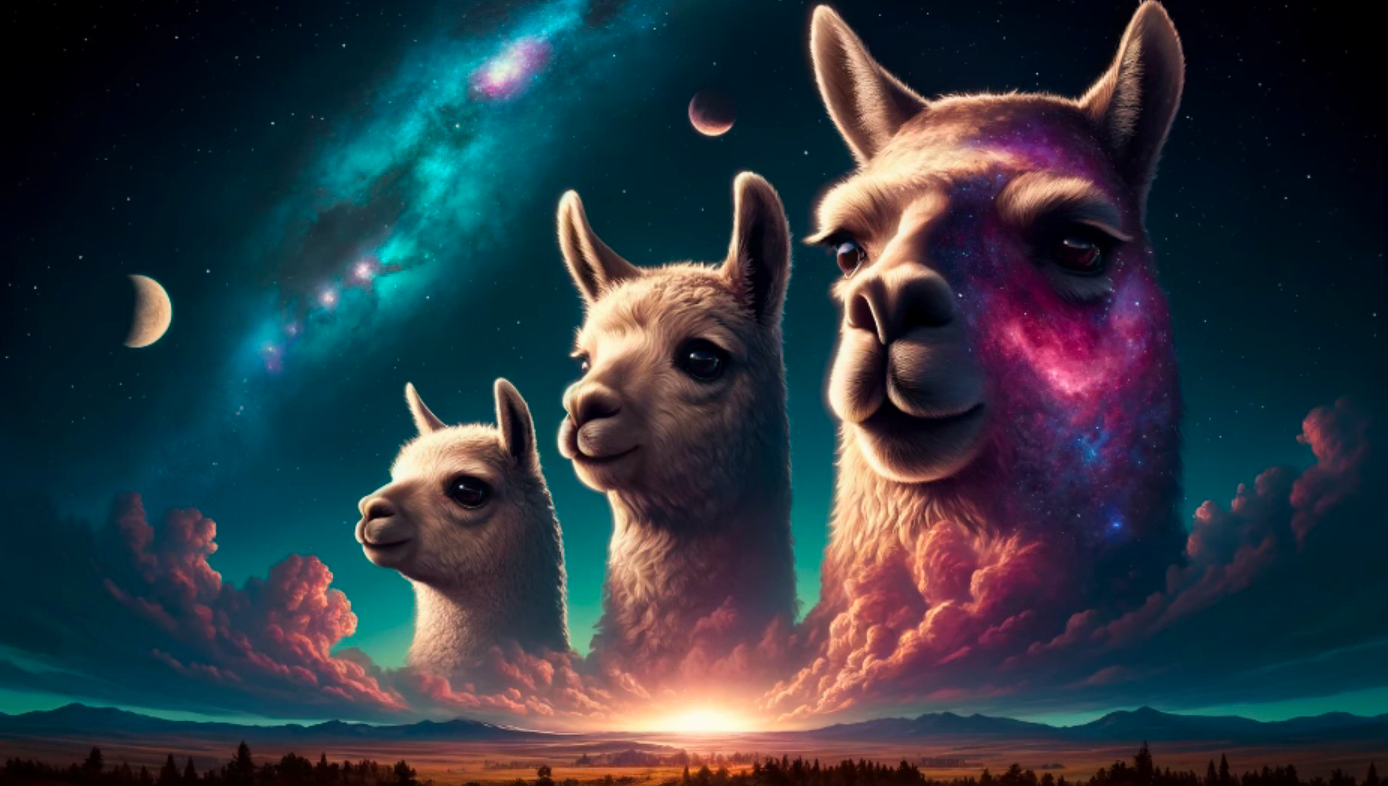
1f45bd1e8577af66a05f5e3fadb0b29
通过ORPO对llama进行微调
ORPO是一种新颖的微调技术,它将传统的监督微调和偏好对齐阶段整合到一个过程中。这减少了训练所需的计算资源和时间。此外,经验结果表明,ORPO在各种模型大小和基准测试中都超过了其他对齐方法。 在本文中,我们将使用ORPO和TRL库来微调新的Llama 3 8B模型。代码可以在Google Colab(https://colab.research.google.com/drive/1eHNWg9gnaXErdAa8_mcvjMupbSS6rDvi?usp=sharing)和GitHub上的LLM(https://github.com/mlabonne/llm-course)课程中找到。
⚖️ ORPO
指令调整和偏好对齐是将大型语言模型(LLMs)适应特定任务的关键技术。传统上,这涉及到一个多阶段的过程:
- 对指令进行监督式微调(SFT)以使模型适应目标领域
- 像人类反馈的强化学习(RLHF)或直接优选优化(DPO)这样的偏好对齐方法,以增加生成优选响应而非被拒绝响应的可能性。
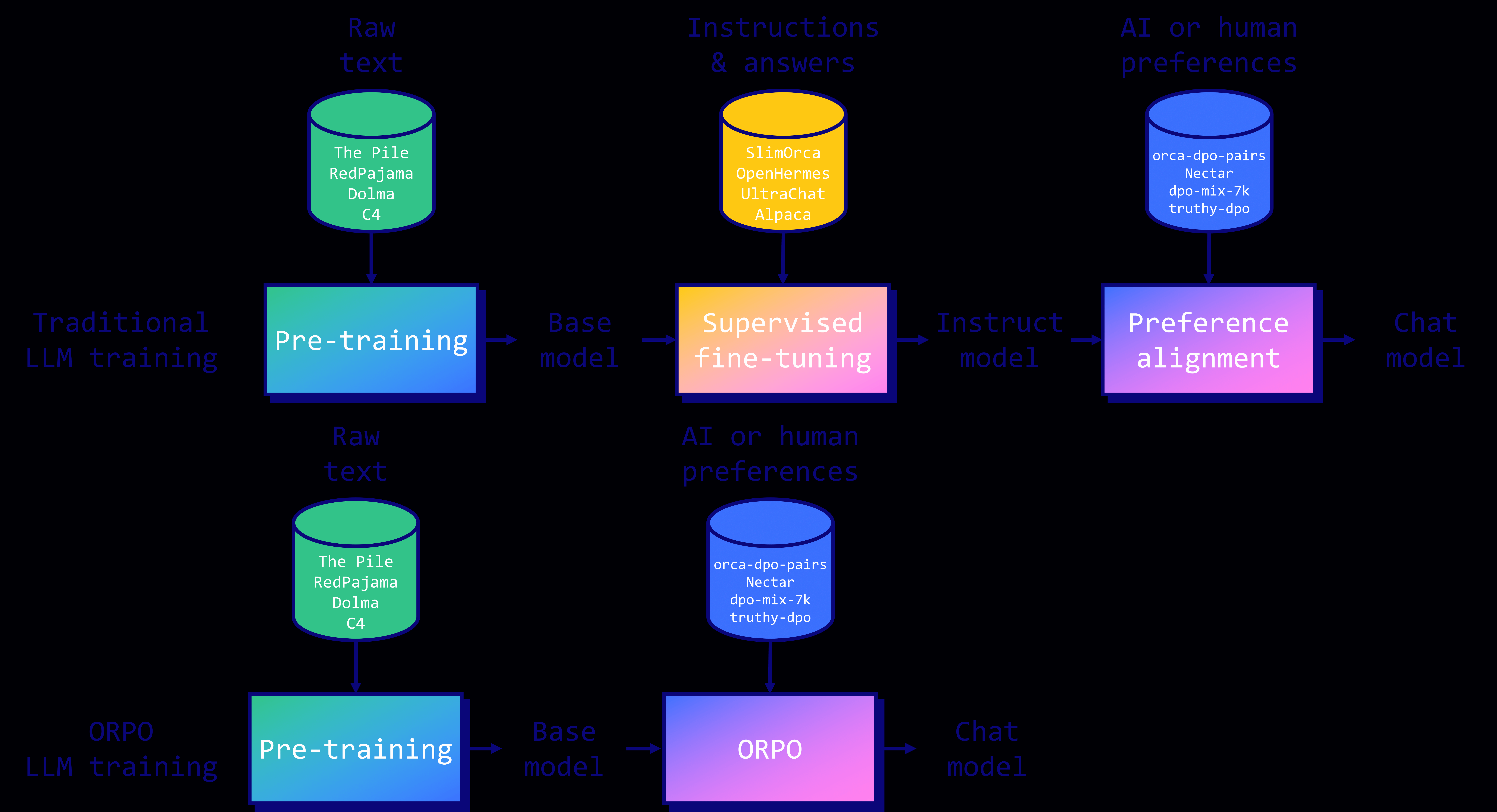
微信图片_20240423001958
然而,研究人员发现这种方法的一个局限性。就是监督微调(SFT)可以有效地让模型适应特定领域,这也就是为什么需要偏好对齐阶段RLHF,扩大受欢迎输出和不受欢迎输出之间概率的差距。
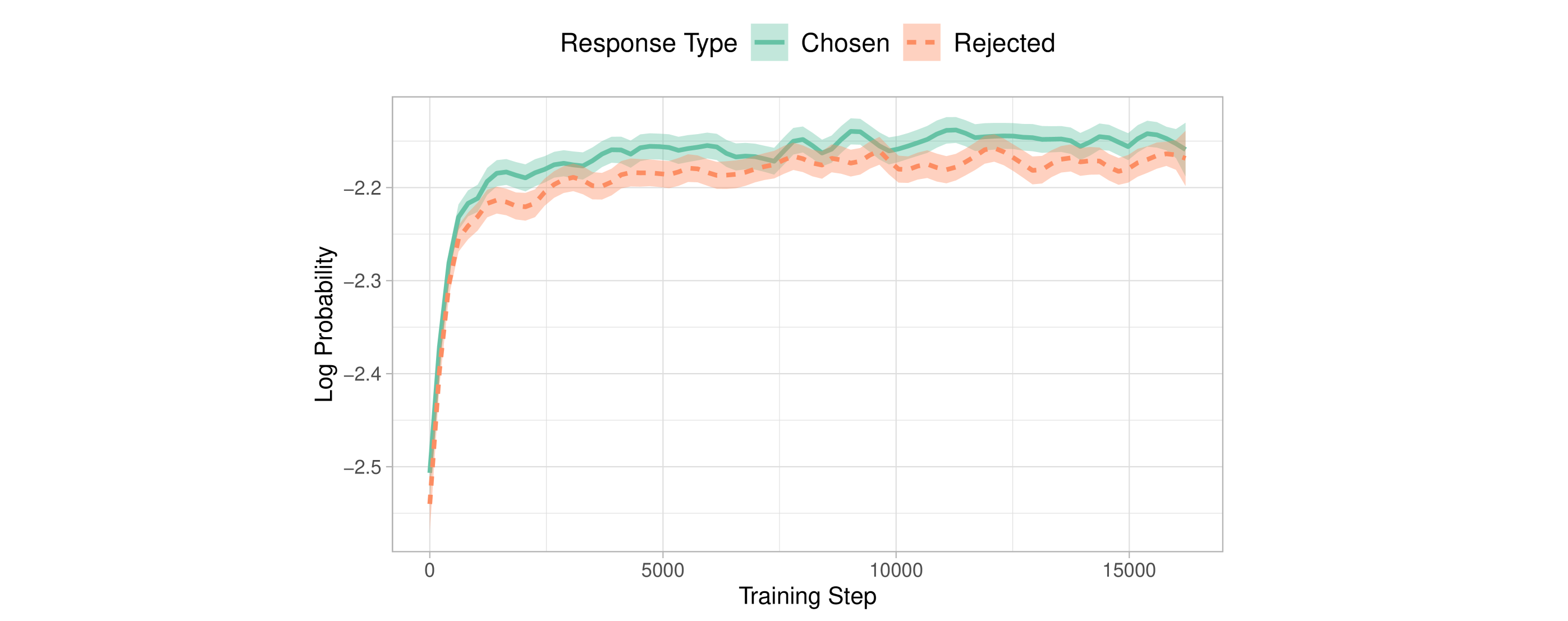
image
SFT过程中,不受欢迎概率增加实证 from ORPO论文
2024年Hong和Lee提出的ORPO通过将SFT和RLHF统一为一个完整训练过程,为这个问题提供了一个优雅的解决方案。ORPO修改了标准language model的训练目标,将负对数似然损失与odds ratio(OR)项结合起来。这种OR损失对不受欢迎的输出施加了轻微的惩罚,同时加大奖励受欢迎的输出,允许模型同时学习目标任务并与人类偏好对齐。

91e1091deacae95fb17f1b6995b94c2
ORPO已经在主要的微调库中得到实现,比如TRL、Axolotl和LLaMA-Factory。在下一节中,我们将看到如何使用TRL进行操作。
💻 开始通过ORPO进行微调
Llama3是Meta开发的最新一代大型语言模型(LLM)。这些模型是在15万亿token的广泛数据集上训练的(相比之下,Llama2的训练数据集为2万亿token)。发布了两种模型尺寸:一个700亿参数的模型和一个更小的80亿参数的模型。700亿参数的模型已经展示了令人印象深刻的性能,在MMLU基准测试中得分为82,在HumanEval基准测试中得分为81.7。
Llama3模型还增加了上下文长度,最多可达8192个token(Llama2为4096个token),并且可能通过RoPE扩展到32k。此外,这些模型使用了一个带有128K-token词汇表的新分词器,减少了编码文本所需token数量的15%。这个词汇表也解释了从70亿到80亿参数的增长。
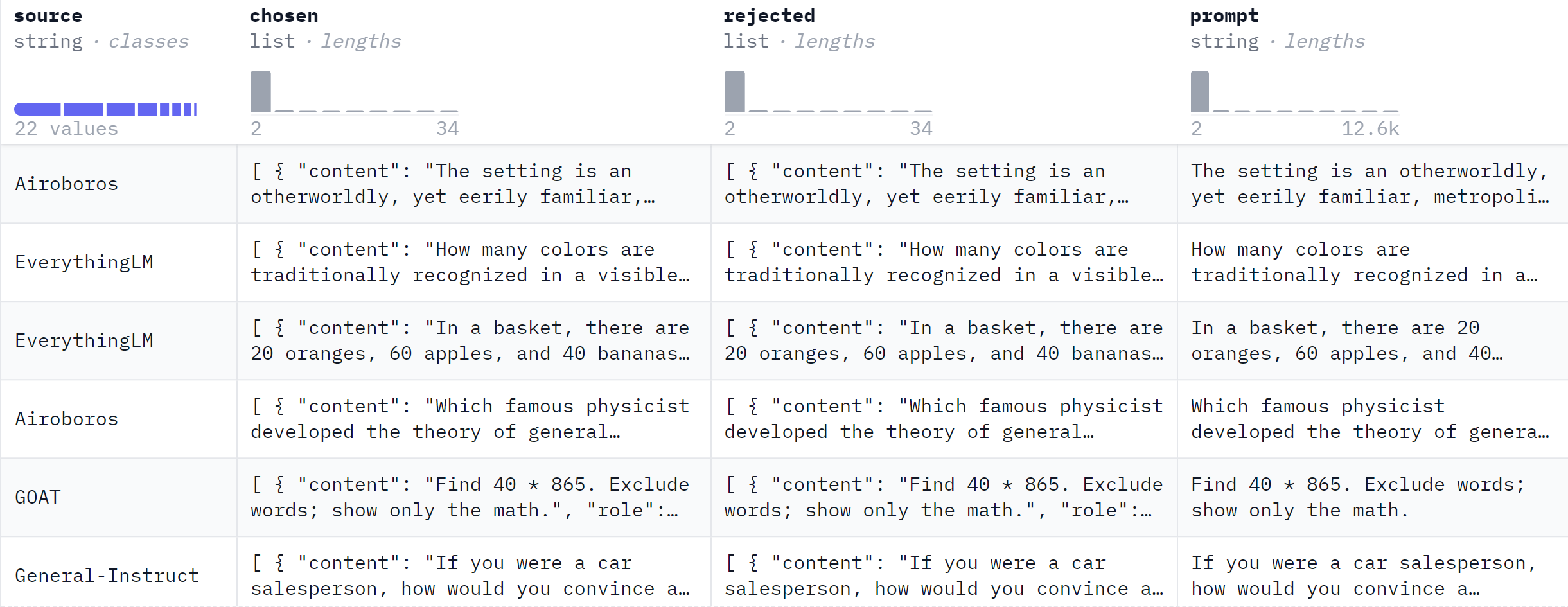
image
ORPO需要一个偏好数据集,包括一个提示、一个被选择的答案和一个被拒绝的答案。在这个例子中,我们将使用mlabonne/orpo-dpo- mix-40k,这是一个由以下高质量DPO数据集组合而成的数据集:
argilla/distilabel-capybara-dpo-7k-binarized
: 高分选择的答案 >=5(2,882个样本) https://huggingface.co/datasets/argilla/distilabel-capybara-dpo-7k-binarizedargilla/distilabel-intel-orca-dpo-pairs
: 高分选择的答案 >=9,不在GSM8K中(2,299个样本) https://huggingface.co/datasets/argilla/distilabel-intel-orca-dpo-pairsargilla/ultrafeedback-binarized-preferences-cleaned
: 高分选择的答案 >=5(22,799个样本) https://huggingface.co/datasets/argilla/ultrafeedback-binarized-preferences-cleanedargilla/distilabel-math-preference-dpo
: 高分选择的答案 >=9(2,181个样本) https://huggingface.co/datasets/argilla/distilabel-math-preference-dpounalignment/toxic-dpo-v0.2
(541个样本) https://huggingface.co/datasets/unalignment/toxic-dpo-v0.2M4-ai/prm_dpo_pairs_cleaned
(7,958个样本) https://huggingface.co/datasets/M4-ai/prm_dpo_pairs_cleanedjondurbin/truthy-dpo-v0.1
(1,016个样本) https://huggingface.co/datasets/jondurbin/truthy-dpo-v0.1 感谢argilla、unalignment、M4-ai和jondurbin提供了源数据集。
开始安装所需的库:
pip install -U transformers datasets accelerate peft trl bitsandbytes wandb
一旦安装完成,我们可以导入必要的库,并登录到W&B(可选):
import gc
import os
import torch
import wandb
from datasets import load_dataset
from google.colab import userdata
from peft import LoraConfig, PeftModel, prepare_model_for_kbit_training
from transformers import (
AutoModelForCausalLM,
AutoTokenizer,
BitsAndBytesConfig,
TrainingArguments,
pipeline,
)
from trl import ORPOConfig, ORPOTrainer, setup_chat_format
wb_token = userdata.get('wandb')
wandb.login(key=wb_token)
如果你有一块较新的GPU,你还应该能够使用Flash Attention库来替换默认的热切关注实现,以一个更有效的方式来实现。
if torch.cuda.get_device_capability()[0] >= 8:
!pip install -qqq flash-attn
attn_implementation = "flash_attention_2"
torch_dtype = torch.bfloat16
else:
attn_implementation = "eager"
torch_dtype = torch.float16
接下来,我们将使用bitsandbytes以4位精度加载Llama 3 8B模型。然后,我们使用PEFT为QLoRA设置LoRA配置。我还使用了方便的setup_chat_format()函数来修改模型和为ChatML支持的分词器。它会自动应用这个聊天模板,添加特殊的令牌,并调整模型的嵌入层的大小以匹配新的词汇表大小。 请注意,你需要提交请求才能访问meta-llama/Meta-Llama-3-8B,并且要登录到你的Hugging Face账户。或者,你可以加载未封闭的模型副本,如NousResearch/Meta--Llama-3-8B。
# Model
base_model = "meta-llama/Meta-Llama-3-8B"
new_model = "OrpoLlama-3-8B"
# QLoRA config
bnb_config = BitsAndBytesConfig(
load_in_4bit=True,
bnb_4bit_quant_type="nf4",
bnb_4bit_compute_dtype=torch_dtype,
bnb_4bit_use_double_quant=True,
)
# LoRA config
peft_config = LoraConfig(
r=16,
lora_alpha=32,
lora_dropout=0.05,
bias="none",
task_type="CAUSAL_LM",
target_modules=['up_proj', 'down_proj', 'gate_proj', 'k_proj', 'q_proj', 'v_proj', 'o_proj']
)
# Load tokenizer
tokenizer = AutoTokenizer.from_pretrained(base_model)
# Load model
model = AutoModelForCausalLM.from_pretrained(
base_model,
quantization_config=bnb_config,
device_map="auto",
attn_implementation=attn_implementation
)
model, tokenizer = setup_chat_format(model, tokenizer)
model = prepare_model_for_kbit_training(model)
现在模型已经准备好进行训练,我们可以处理数据集。我们加载mlabonne/orpo-dpo-mix-40k,并使用apply_chat_template()函数将“chosen”和“rejected”列转换为ChatML格式。请注意,我只使用了1,000个样本,而不是整个数据集,因为运行起来会花费太长时间。
dataset_name = "mlabonne/orpo-dpo-mix-40k"
dataset = load_dataset(dataset_name, split="all")
dataset = dataset.shuffle(seed=42).select(range(10))
def format_chat_template(row):
row["chosen"] = tokenizer.apply_chat_template(row["chosen"], tokenize=False)
row["rejected"] = tokenizer.apply_chat_template(row["rejected"], tokenize=False)
return row
dataset = dataset.map(
format_chat_template,
num_proc= os.cpu_count(),
)
dataset = dataset.train_test_split(test_size=0.01)
首先,我们需要设置一些超参数: 学习率:与传统的SFT或者DPO相比,ORPO使用的学习率非常低。这个值8e-6来自原始论文,大致对应于SFT的学习率1e-5和DPO的学习率5e-6。我建议在真正的微调中将其增加到大约1e-6。 beta:它是论文中的参数,其默认值为0.1。来自原始论文的一个附录显示了如何通过消融研究选择它。 其他参数,如最大长度和批量大小,都设置为尽可能多地使用VRAM(在此配置中约为20 GB)。理想情况下,我们将对模型进行3-5个周期的训练,但这里我们将坚持1个周期。 最后,我们可以使用ORPOTrainer来训练模型,它充当一个包装器。
orpo_args = ORPOConfig(
learning_rate=8e-6,
beta=0.1,
lr_scheduler_type="linear",
max_length=1024,
max_prompt_length=512,
per_device_train_batch_size=2,
per_device_eval_batch_size=2,
gradient_accumulation_steps=4,
optim="paged_adamw_8bit",
num_train_epochs=1,
evaluation_strategy="steps",
eval_steps=0.2,
logging_steps=1,
warmup_steps=10,
report_to="wandb",
output_dir="./results/",
)
trainer = ORPOTrainer(
model=model,
args=orpo_args,
train_dataset=dataset["train"],
eval_dataset=dataset["test"],
peft_config=peft_config,
tokenizer=tokenizer,
)
trainer.train()
trainer.save_model(new_model)
在L4 GPU上对这1000个样本进行模型训练大约需要2个小时。让我们查看W&B的图:
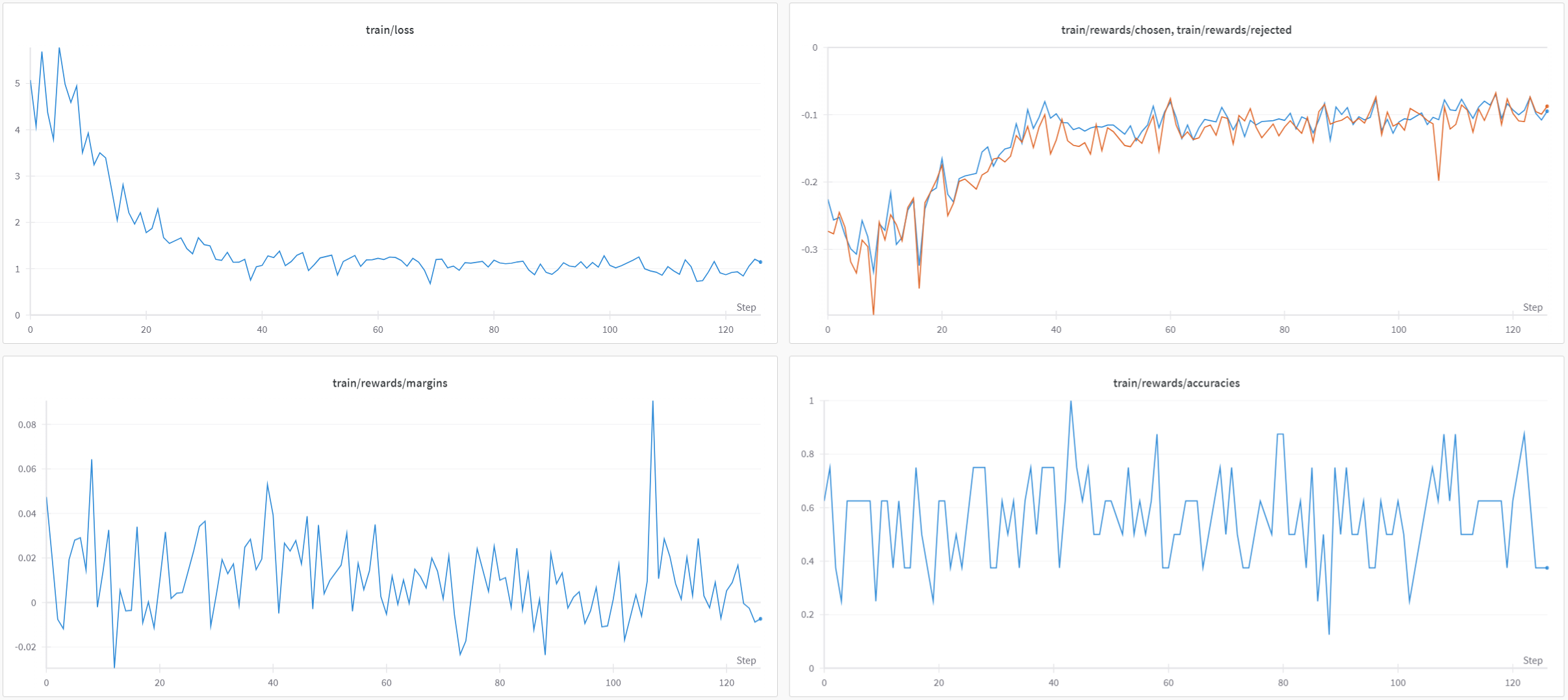
image
当loss降低时,受欢迎输出和不受欢迎输出之间的差异并不明显:平均边界和准确度分别仅略高于0和0.5。
在原始论文中,作者们在 Anthropic/hh-rlhf
数据集(161k个样本)上训练模型进行了10个epochs,这比我们现在运行的时间要长得多。他们还对Llama3进行了实验,并且友好地与我分享了他们的日志(感谢Jiwoo Hong)。
在本教程的结尾,让我们将QLoRA适配器与基础模型合并,并将其推送到Hugging Face Hub。
# Flush memory
del trainer, model
gc.collect()
torch.cuda.empty_cache()
# Reload tokenizer and model
tokenizer = AutoTokenizer.from_pretrained(base_model)
model = AutoModelForCausalLM.from_pretrained(
base_model,
low_cpu_mem_usage=True,
return_dict=True,
torch_dtype=torch.float16,
device_map="auto",
)
model, tokenizer = setup_chat_format(model, tokenizer)
# Merge adapter with base model
model = PeftModel.from_pretrained(model, new_model)
model = model.merge_and_unload()
model.push_to_hub(new_model, use_temp_dir=False)
tokenizer.push_to_hub(new_model, use_temp_dir=False)
恭喜,我们完成了Llama3:mlabonne/OrpoLlama-3-8B的快速微调。你可以使用这个Hugging Face Space(这里有一个notebook,让你自己来实践)来使用它。尽管模型训练不足,正如W&B曲线所强调的那样,我还是使用LLM AutoEval在Nous的基准测试套件上进行了一些评估。
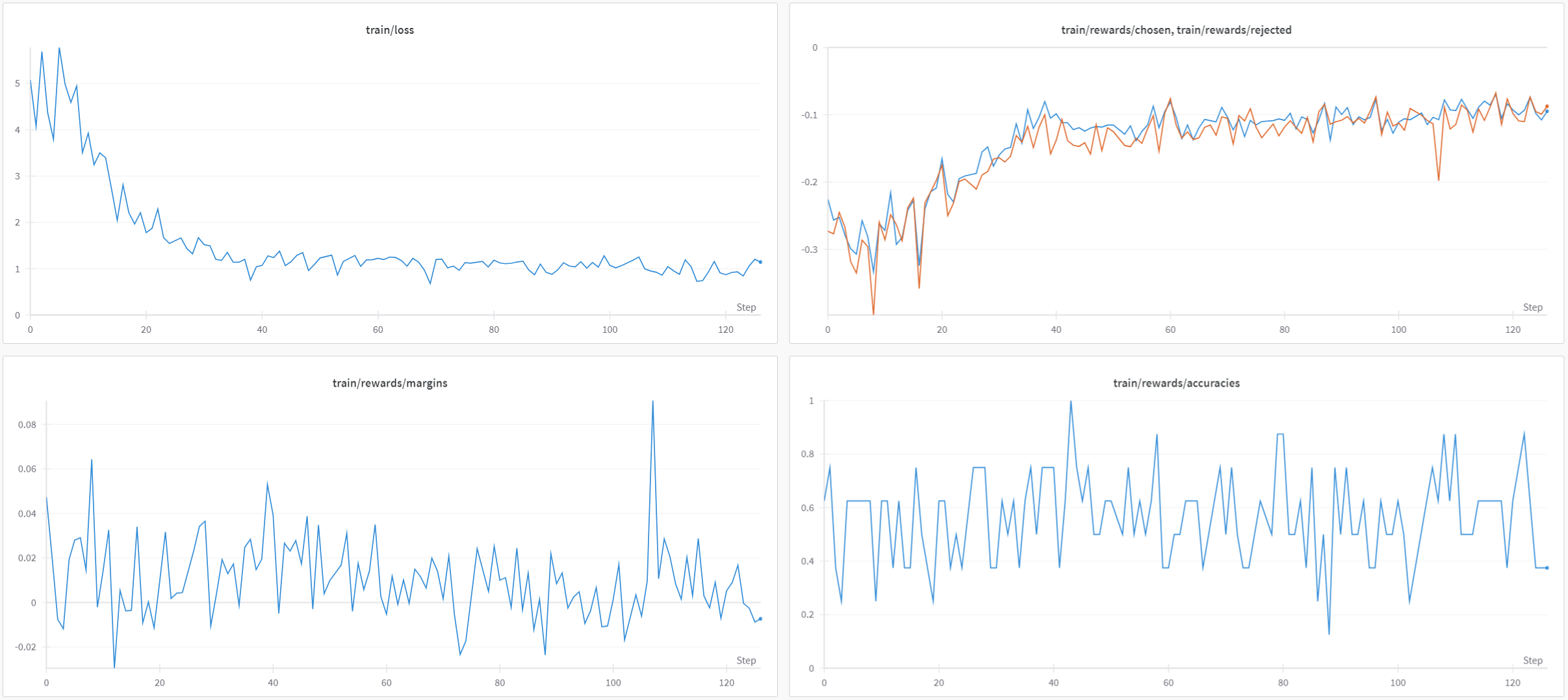
image
我们的ORPO微调实际上相当不错,并且提高了基础模型在每个基准测试上的性能。这是令人鼓舞的,并且很可能意味着在整个40k样本上进行微调将带来很好的结果。
对于开源社区来说,这是一个激动人心的时刻,越来越多的高质量开放权重模型被发布。闭源和开放权重模型之间的差距正在逐渐缩小,而微调是获取您用例最佳性能的重要工具。
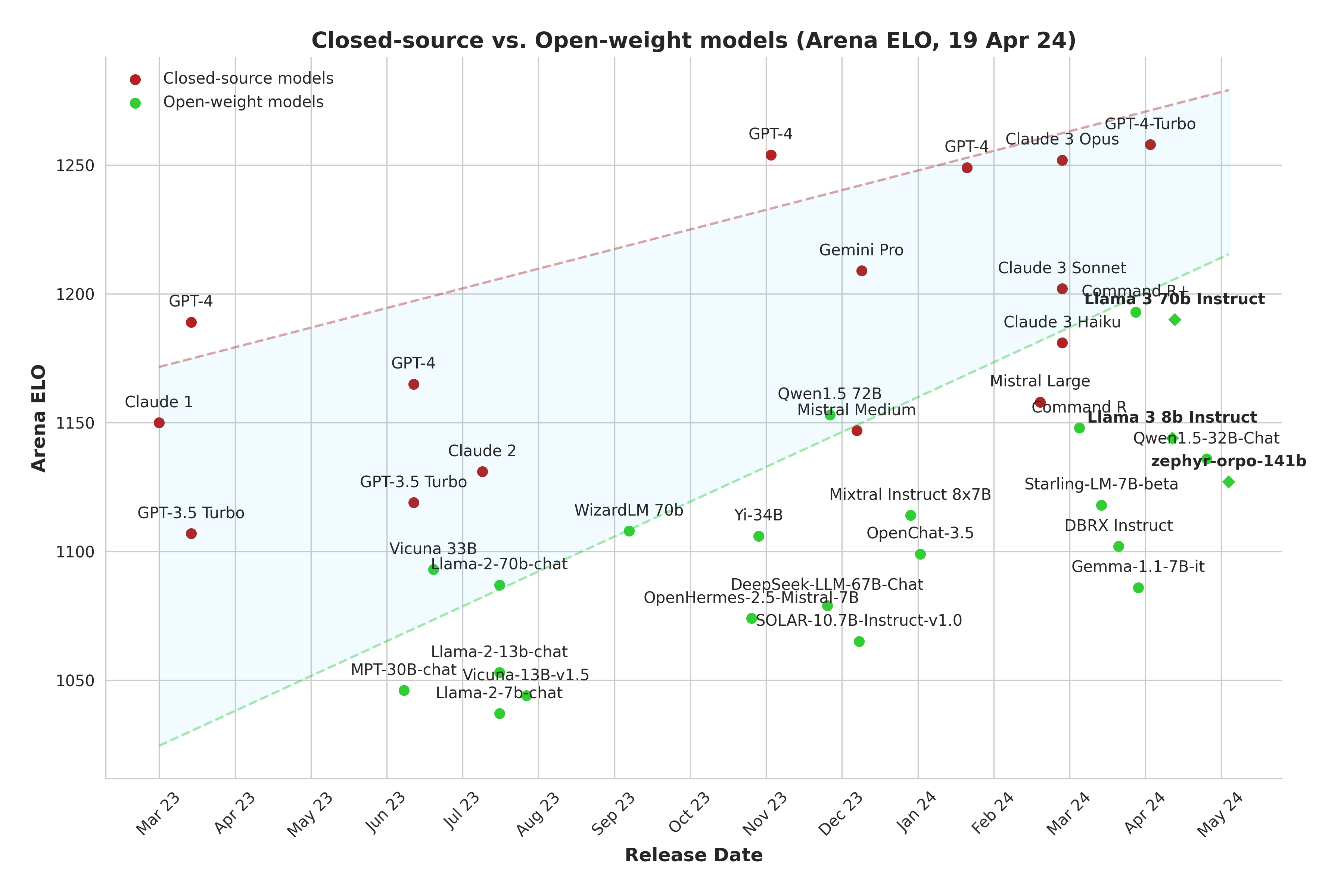
image
在这篇教程中,我们介绍了ORPO算法,并解释了它如何将SFT(监督式微调)和RLHF统一为单一的过程。然后,我们使用TRL(Transformer Reinforcement Learning)对一个定制的偏好数据集上的Llama3-8B进行微调。最终模型展示了令人鼓舞的结果,并突显了ORPO作为新的微调范式的潜力。
我希望这很有帮助,并推荐你运行Colab笔记本来微调你自己的Llama3模型。在将来的文章中,我们将看到如何创建高质量的数据集——这是一个经常被忽视的点。
最后文章参考自:https://huggingface.co/blog/mlabonne/orpo-llama-3
Recommend
About Joyk
Aggregate valuable and interesting links.
Joyk means Joy of geeK