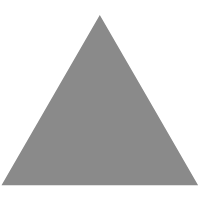
3
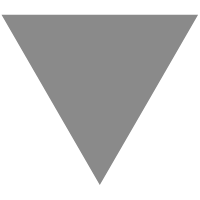
[2311.13771] Work-Efficient Parallel Derandomization II: Optimal Concentrations...
source link: https://arxiv.org/abs/2311.13771
Go to the source link to view the article. You can view the picture content, updated content and better typesetting reading experience. If the link is broken, please click the button below to view the snapshot at that time.
Computer Science > Data Structures and Algorithms
[Submitted on 23 Nov 2023]
Work-Efficient Parallel Derandomization II: Optimal Concentrations via Bootstrapping
We present an efficient parallel derandomization method for randomized algorithms that rely on concentrations such as the Chernoff bound. This settles a classic problem in parallel derandomization, which dates back to the 1980s. Consider the \textit{set balancing} problem where m sets of size at most s are given in a ground set of size n, and we should partition the ground set into two parts such that each set is split evenly up to a small additive (discrepancy) bound. A random partition achieves a discrepancy of O(slogm−−−−−−√) in each set, by Chernoff bound. We give a deterministic parallel algorithm that matches this bound, using near-linear work and polylogarithmic depth. The previous results were weaker in discrepancy and/or work bounds: Motwani, Naor, and Naor [FOCS'89] and Berger and Rompel [FOCS'89] achieve discrepancy sε⋅O(slogm−−−−−−√) with work O~(m+n+∑mi=1|Si|)⋅mΘ(1/ε) and polylogarithmic depth; the discrepancy was optimized to O(slogm−−−−−−√) in later work, e.g. by Harris [Algorithmica'19], but the work bound remained high at O~(m4n3). Ghaffari, Grunau, and Rozhon [FOCS'23] achieve discrepancy s/poly(log(nm))+O(slogm−−−−−−√) with near-linear work and polylogarithmic-depth. Notice that this discrepancy is barely sublinear with respect to the trivial bound of s. Our method relies on a novel bootstrapping idea that uses crude partitioning algorithms as a subroutine. In particular, we solve the problem recursively, by using the crude partition in each iteration to split the variables into many smaller parts, and then we find a constraint for the variables in each part such that we reduce the overall number of variables in the problem. The scheme relies on an interesting application of the multiplicative weights update method to control the variance losses in each iteration.
Subjects: | Data Structures and Algorithms (cs.DS) |
Cite as: | arXiv:2311.13771 [cs.DS] |
(or arXiv:2311.13771v1 [cs.DS] for this version) | |
https://doi.org/10.48550/arXiv.2311.13771 |
Recommend
About Joyk
Aggregate valuable and interesting links.
Joyk means Joy of geeK