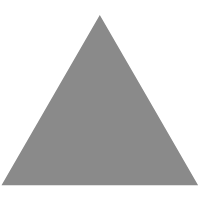
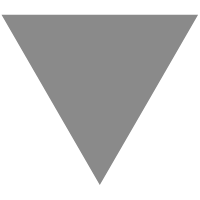
[2311.14648] Calibrated Language Models Must Hallucinate
source link: https://arxiv.org/abs/2311.14648
Go to the source link to view the article. You can view the picture content, updated content and better typesetting reading experience. If the link is broken, please click the button below to view the snapshot at that time.
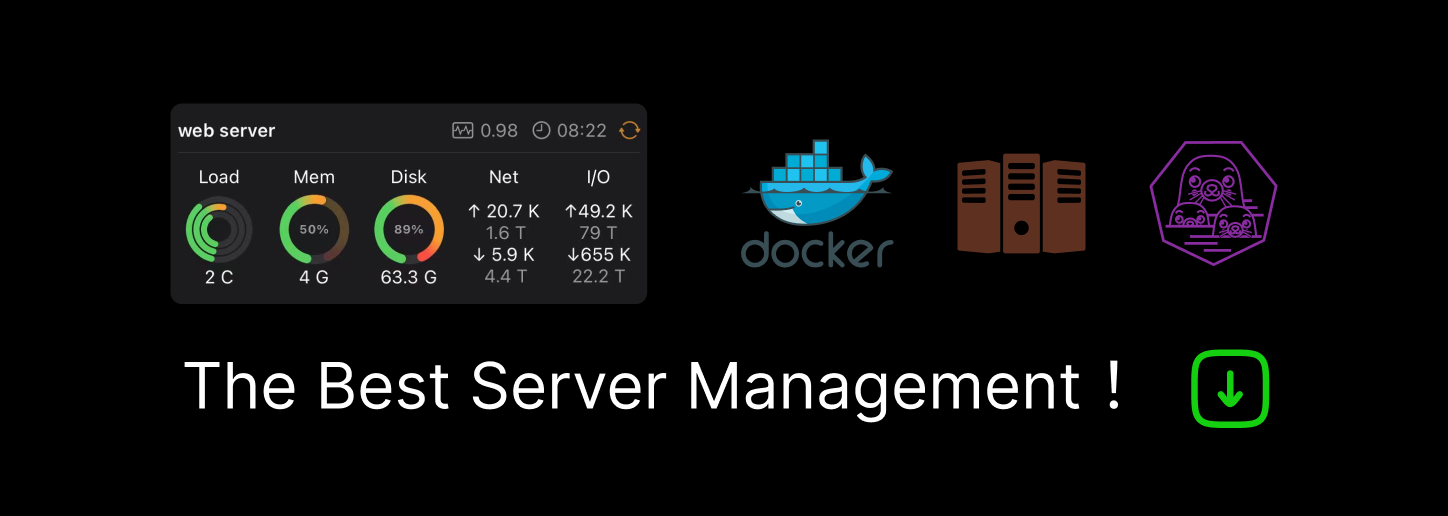
Computer Science > Computation and Language
Calibrated Language Models Must Hallucinate
Recent language models generate false but plausible-sounding text with surprising frequency. Such "hallucinations" are an obstacle to the usability of language-based AI systems and can harm people who rely upon their outputs. This work shows that there is an inherent statistical lower-bound on the rate that pretrained language models hallucinate certain types of facts, having nothing to do with the transformer LM architecture or data quality. For "arbitrary" facts whose veracity cannot be determined from the training data, we show that hallucinations must occur at a certain rate for language models that satisfy a statistical calibration condition appropriate for generative language models. Specifically, if the maximum probability of any fact is bounded, we show that the probability of generating a hallucination is close to the fraction of facts that occur exactly once in the training data (a "Good-Turing" estimate), even assuming ideal training data without errors.
One conclusion is that models pretrained to be sufficiently good predictors (i.e., calibrated) may require post-training to mitigate hallucinations on the type of arbitrary facts that tend to appear once in the training set. However, our analysis also suggests that there is no statistical reason that pretraining will lead to hallucination on facts that tend to appear more than once in the training data (like references to publications such as articles and books, whose hallucinations have been particularly notable and problematic) or on systematic facts (like arithmetic calculations). Therefore, different architectures and learning algorithms may mitigate these latter types of hallucinations.
Comments: | In Proceedings of the 56th Annual ACM Symposium on Theory of Computing (STOC) 2024 |
Subjects: | Computation and Language (cs.CL); Artificial Intelligence (cs.AI) |
Cite as: | arXiv:2311.14648 [cs.CL] |
(or arXiv:2311.14648v3 [cs.CL] for this version) | |
https://doi.org/10.48550/arXiv.2311.14648 |
Submission history
From: Adam Kalai [view email][v1] Fri, 24 Nov 2023 18:29:50 UTC (165 KB)
[v2] Sun, 3 Dec 2023 15:28:02 UTC (168 KB)
[v3] Wed, 20 Mar 2024 02:21:20 UTC (168 KB)
Recommend
-
60
Instead of predicting class values directly for a classification problem, it can be convenient to predict the probability of an observation belonging to each possible class. Predicting probabilities allows some f...
-
11
Followup on yesterday's "well-calibrated interviewer" post Wow! Yesterday's post about being tagged a "well-calibrated interviewer" has attracted quite a bit of attention. T...
-
12
The "well-calibrated interviewer" curse Once, there was a place which did a whole bunch of technical interviewing. They were something of an anomaly in their industry, and they got a reputation as being seriously tough. Everyone...
-
11
Calibrated Achievement Points (CAP) 1 It’s a...
-
15
Find Out How Well Your Machine Learning Model is Calibrated If your machine learning model pr...
-
5
Virtual distro — No Linux? No problem. Just get AI to hallucinate it for you ChatGPT-generated command line can create virtual files, execute code, play games....
-
5
Macbook Air M2 calibrated color profile O
-
9
Both humans and AI hallucinate—but not in the same way by Sarah Vivienne Bentley and Claire Naughtin,...
-
7
Computer Science > Computation and Language [Submitted on 28 Nov 2023 (v1), last revised...
-
6
Computer Science > Data Structures and Algorithms [Submitted on 29 Nov 2023] Sparsifying generalized linear models...
About Joyk
Aggregate valuable and interesting links.
Joyk means Joy of geeK