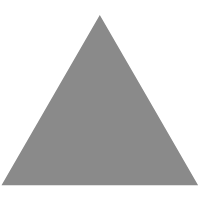
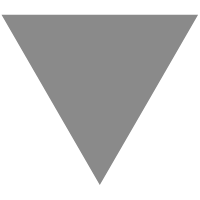
吴恩达来信:智能体如何优化LLM性能
source link: https://zhuanlan.zhihu.com/p/688226963
Go to the source link to view the article. You can view the picture content, updated content and better typesetting reading experience. If the link is broken, please click the button below to view the snapshot at that time.
吴恩达来信:智能体如何优化LLM性能
Dear friends,
I think AI agent workflows will drive massive AI progress this year — perhaps even more than the next generation of foundation models. This is an important trend, and I urge everyone who works in AI to pay attention to it.
Today, we mostly use LLMs in zero-shot mode, prompting a model to generate final output token by token without revising its work. This is akin to asking someone to compose an essay from start to finish, typing straight through with no backspacing allowed, and expecting a high-quality result. Despite the difficulty, LLMs do amazingly well at this task!
With an agent workflow, however, we can ask the LLM to iterate over a document many times. For example, it might take a sequence of steps such as:
- Plan an outline.
- Decide what, if any, web searches are needed to gather more information.
- Write a first draft.
- Read over the first draft to spot unjustified arguments or extraneous information.
- Revise the draft taking into account any weaknesses spotted.
- And so on.
This iterative process is critical for most human writers to write good text. With AI, such an iterative workflow yields much better results than writing in a single pass.
Devin’s splashy demo recently received a lot of social media buzz. My team has been closely following the evolution of AI that writes code. We analyzed results from a number of research teams, focusing on an algorithm’s ability to do well on the widely used HumanEval coding benchmark. You can see our findings in the diagram below.
GPT-3.5 (zero shot) was 48.1% correct. GPT-4 (zero shot) does better at 67.0%. However, the improvement from GPT-3.5 to GPT-4 is dwarfed by incorporating an iterative agent workflow. Indeed, wrapped in an agent loop, GPT-3.5 achieves up to 95.1%.
Open source agent tools and the academic literature on agents are proliferating, making this an exciting time but also a confusing one. To help put this work into perspective, I’d like to share a framework for categorizing design patterns for building agents. My team AI Fund is successfully using these patterns in many applications, and I hope you find them useful.
- Reflection: The LLM examines its own work to come up with ways to improve it.
- Tool use: The LLM is given tools such as web search, code execution, or any other function to help it gather information, take action, or process data.
- Planning: The LLM comes up with, and executes, a multistep plan to achieve a goal (for example, writing an outline for an essay, then doing online research, then writing a draft, and so on).
- Multi-agent collaboration: More than one AI agent work together, splitting up tasks and discussing and debating ideas, to come up with better solutions than a single agent would.
Next week, I’ll elaborate on these design patterns and offer suggested readings for each.
Keep learning!
Andrew
P.S. Build an optimized large language model (LLM) inference system from the ground up in our new short course “Efficiently Serving LLMs,” taught by Predibase CTO Travis Addair.
- Learn techniques like KV caching, continuous batching, and quantization to speed things up and optimize memory usage.
- Benchmark LLM optimizations to explore the trade-offs between latency and serving many users at once.
- Use low-rank adaptation (LoRA) to serve hundreds of custom fine-tuned models on a single device efficiently.
亲爱的朋友们,
我认为AI智能体工作流将在今年推动人工智能的巨大进步——甚至可能超过下一代基础模型。这是一个重要的趋势,我希望所有从事人工智能工作的人都能关注它。
目前,我们主要在zero-shot模式下使用LLM,提示模型在不修改其工作的情况下逐token生成最终输出。这类似于要求某人从头到尾写一篇文章,直接输入、不允许空白,并期望得到高质量的结果。尽管困难重重,但LLM在这项任务上表现非常好!
然而,对于智能体工作流,我们可以要求LLM对文档进行多次迭代。例如,它可能采取一系列步骤,如:
● 拟定大纲。
● 如果需要的话,决定需要进行哪些网络搜索来收集更多信息。
● 撰写初稿。
● 通读初稿,找出不合理的论点或无关的信息。
● 根据找到的薄弱点修改初稿。
● 等等。
这种反复的过程对于大多数作家写出好的文本是至关重要的。使用AI,这样的迭代工作流程产生的结果会比单次编写好得多。
Devin引人注目的演示最近在社交媒体上引起了很多关注。我的团队一直在密切关注编写代码的人工智能的发展。我们分析了来自多个研究团队的结果,重点关注算法在广泛使用的HumanEval编程基准上表现良好的能力。你可以在下面的图表中看到我们的发现。
GPT-3.5 (zero shot) 正确率为48.1%。GPT-4 (zero shot) 的表现更好,为67.0%。然而,从GPT-3.5到GPT-4的提升与合并迭代智能体工作流相比显得微不足道。实际上,在智能体循环里运行,GPT-3.5可以达到95.1%的正确率。
开源智能体工具和关于智能体的学术文献正在激增,这是一个令人兴奋的时刻,但也是一个令人困惑的时刻。为了更好地理解这项工作,我想分享一个对搭建智能体的设计模式进行分类的框架。我的团队AI Fund在许多应用中成功地使用了这些模式,我希望你觉得它们有用。
● Reflection:LLM审查自己的工作,提出改进的方法。
● Tool use:LLM被赋予工具,如网络搜索,代码执行,或任何其他功能,以帮助它收集信息,采取行动,或处理数据。
● Planning:LLM提出并执行一个多步骤计划来实现一个目标(例如,编写一篇文章的大纲,然后进行在线研究、编写草稿,等等)。
● Multi-agent collaboration:多个AI智能体一起工作,分解任务、讨论和辩论想法,提出比单个智能体更好的解决方案。
下周,我将详细介绍这些设计模式,并为每种模式提供建议阅读材料。
请不断学习!
吴恩达
附注:学习由Predibase首席技术官Travis Addair教授的新短期课程“Efficiently Serving LLMs”,从头开始构建优化后的LLM推理系统。
● 学习KV缓存、连续批处理和量化等技术来加快速度并优化内存使用。
● 基准LLM优化,以探索延迟和同时为许多用户服务之间的权衡。
● 使用低秩适配(low-rank adaptation , LoRA)在单个设备上高效地服务数百个自定义微调模型。
欢迎点此注册学习~
Recommend
About Joyk
Aggregate valuable and interesting links.
Joyk means Joy of geeK