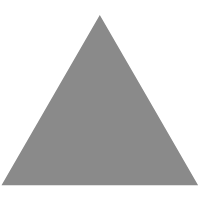
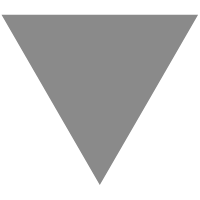
Year in review: Learnings and predictions for generative AI in the enterprise
source link: https://venturebeat.com/ai/year-in-review-learnings-and-predictions-for-generative-ai-in-the-enterprise/
Go to the source link to view the article. You can view the picture content, updated content and better typesetting reading experience. If the link is broken, please click the button below to view the snapshot at that time.
Year in review: Learnings and predictions for generative AI in the enterprise

Presented by Samsara
It’s been a little over a year since ChatGPT exploded onto the public scene. And if you’ve been watching the ChatGPT phenomenon from the world of B2B technology, don’t let the word “consumer” lull you into a sense of complacency. Consumer technology can have a tremendous influence on the B2B tech landscape. For example, ride-share apps with real-time location tracking have set an expectation for real-time delivery ETAs for commercial food and beverage distribution.
That said, it’s still early days when it comes to understanding the impact of this technology in an enterprise environment. LLMs are already widely used in some functions — like marketing and human resources — but determining how to integrate these tools into industries like construction, manufacturing, trucking and others that are undergoing digital transformation is still an open question.
Here are some insights about how enterprises can expect to use generative AI now, as well as how the technology will continue to evolve in 2024 and the years to come.
When humans and AI collaborate, it makes more time for strategic work
Most workers spend an inordinate amount of time on administrative tasks, such as data entry or checking messages. In fact, a survey by Zapier recently found that 76% of employees spend less than three hours per week on strategic work. We need to streamline administrative tasks to reclaim that time for more impactful work, and conversational AI will be a powerful tool to help us do just that.
AI could be applied to any use case where people spend time manually entering, retrieving or delivering data, from generated customer support responses to content for social media. One thing these use cases have in common, however, is the need for close collaboration between humans and AI systems. AI tools excel at generating content, but human oversight is crucial to ensure accuracy, ethical use and context-appropriate responses.
LLMs aren’t a silver bullet for every industry, including physical operations
LLMs are undoubtedly very good at many things — summarizing information, for example, or generating content — but there are also problems these models are not designed to solve on their own. In the world of physical operations, like trucking and construction, many of the complex challenges our customers face will likely require a combination of technologies. For example, an LLM that interfaces with various capabilities around data processing, automatic validation, querying and more.
The size and complexity of the underlying data landscape also matters. In physical operations, we deal with petabyte-scale, multimodal data, including video data, sensor data and location data. Even the largest LLMs won’t be able to uncover insights from this information on their own.
What’s next: Explainable AI will drive trust, acceptance and adoption of AI
For sectors like physical operations, the next frontier in AI is the synergy between AI, IoT and real-time insights across a diversity of data. The value of those insights, however, will depend on how humans understand what that data is, where it comes from, and what it actually means.
Leaders will seek new ways to gain deeper insights into model predictions and modernize their tech stack. To do this effectively, I expect organizations to become more interested in explainable AI, or XAI. XAI sheds light on the black-box nature of AI systems by providing deeper insights into model predictions. It will give users a better understanding of how their AI systems are interacting with their data, and it’s essential for earning user trust. Ultimately, more trust will foster a greater sense of reliability and predictability.
Here’s an example: Say you have an intelligent AI agent that executes workflows on behalf of users. In this case, instead of a user simply entering an instruction and getting a result, XAI can reveal more of the decision-making process that the AI agent uses to obtain that result. This will empower users to better steer the agent toward desired behaviors and outcomes.
AI for vertical industries will drive competition for specialized talent
AI models can call on a vast amount of information, but that doesn’t mean that one tool can be all things to all organizations. That’s why in 2024 I anticipate a continuous maturation of generative AI technologies, with an emphasis on domain-specific knowledge and real-time adaptation to evolving scenarios. For example, the details that an AI model needs to “know” for a company in the oil and gas sector may be vastly different than for a logistics company. The convergence of generative AI with domain expertise will facilitate more nuanced and valuable insights, making AI a quintessential partner in decision-making processes across industries.
As AI becomes more focused and integrated into not only products but also operational frameworks, demand for specialized AI talent will continue to surge in 2024. In addition to foundational skills in machine learning, statistics and programming, I expect to see an increased demand for expertise in domain-specific AI applications and AI governance.
Beyond technical roles, organizations will need to focus on reskilling across a wide range of job functions to ensure they can use AI tools effectively — for example, teaching your human resources staff to use an AI assistant. And companies that start reskilling now will have an advantage: A recent McKinsey & Co. survey found that companies that are AI “high-performers” are more than three times more likely to reskill a larger portion of their workforce than AI laggards.
It’s clear that generative AI promises a lot of exciting innovations for 2024 and the years ahead. However, as powerful and transformative as AI can be, gains will be hard to come by unless we remember that humans are always at the center of technological advances. The right prompts and the right data can do a lot for AI to effectively solve problems, but prioritizing your people is the only way to ensure success.
To learn more about AI for physical operations, visit: https://samsara.com/guides/make-more-possible/build-for-the-future.
Evan Welbourne is Head of AI and Data at Samsara.
Sponsored articles are content produced by a company that is either paying for the post or has a business relationship with VentureBeat, and they’re always clearly marked. For more information, contact [email protected].
Recommend
About Joyk
Aggregate valuable and interesting links.
Joyk means Joy of geeK