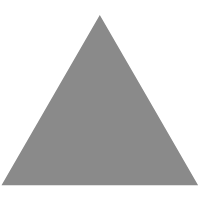
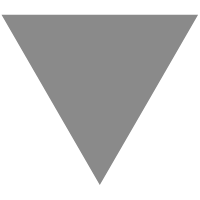
AI search and data privacy considerations
source link: https://www.algolia.com/blog/ai/ai-search-and-data-privacy-considerations/
Go to the source link to view the article. You can view the picture content, updated content and better typesetting reading experience. If the link is broken, please click the button below to view the snapshot at that time.
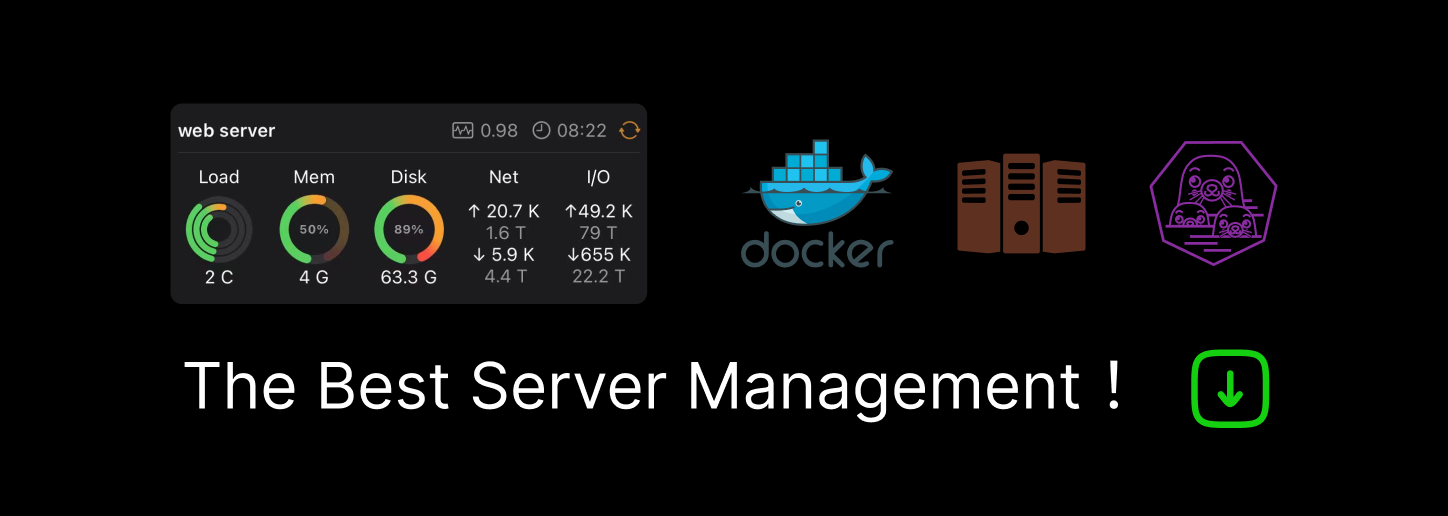
Artificial Intelligence search algorithms have revolutionized the way we access information, providing personalized and efficient search results.
However, the use of AI in search raises significant concerns regarding data privacy. There is a fine balance between AI search algorithms and data privacy, how user data is handled, the potential risks involved, and the measures required to protect privacy rights.
Data collection and usage
AI search algorithms rely on vast amounts of data to provide accurate and personalized search results. When users interact with search engines, they generate valuable information about their preferences, interests, and online behavior. This data, often referred to as user data or personal data, is collected and analyzed to improve the search experience. However, this data collection raises concerns about the potential misuse or mishandling of sensitive information.
One primary concern is the potential for user profiling. AI algorithms can analyze user data to create detailed profiles that encompass personal characteristics, preferences, and online behavior. These profiles can then be used to deliver personalized advertisements. While personalized ads can enhance the user experience, there is a fine line between personalization and invasion of privacy. Users may feel uneasy knowing that their data is being utilized to manipulate their decisions or exploit their vulnerabilities.
Technological safeguards
In addition to regulatory frameworks, technological safeguards need to be created for preserving data privacy in AI search algorithms. Privacy-enhancing technologies can be integrated into search systems to provide stronger protections.
- Privacy-preserving algorithms: Privacy-preserving algorithms are designed to perform data analysis while preserving the privacy of individual data. These algorithms employ cryptographic techniques and mathematical models to ensure that sensitive information remains secure and inaccessible to unauthorized parties. An interesting exemple is Federated learning. Instead of sending raw user data to a central server, the learning algorithm is brought to the data on local servers. The devices perform model training using their local data while only sharing model updates with the central server.
- Differential privacy mechanisms: Differential privacy mechanisms are privacy protection techniques that aim to add noise or randomization to query responses or data releases. By injecting controlled randomness into the data, these mechanisms prevent the identification of specific individuals while still allowing for meaningful statistical analysis.
- Data anonymization: Data anonymization refers to the process of transforming personal data in a way that makes it impossible or extremely difficult to identify individuals. Techniques such as data masking, generalization, or aggregation are applied to remove direct identifiers and protect sensitive information, reducing the risk of re-identification. The goal is to achieve a high level of anonymity while maintaining the usefulness of the data for analysis or research purposes.
- Synthetic data: Synthetic data, also known as simulated or artificial data, offers a promising solution to the privacy concerns associated with real user data. Synthetic data is generated using algorithms and statistical models, mimicking the statistical characteristics of real data while ensuring that it contains no personally identifiable information (PII). This synthetic data can be used for training AI search algorithms and conducting research without the risks associated with real user data.
By incorporating these safeguards into AI search systems, organizations can ensure that user data is treated with the utmost care while maintaining the effectiveness of search results.
Engineering privacy
So much of the news today focuses on how AI may be trampling individual’s and corporation’s digital sovereignty. Algolia takes this very seriously and we are currently evaluating additional methods to protect privacy that go above and beyond the things mentioned above. This includes:
- Ways to implement additional safeguards that keep our customers’ data from being cross-pollinated by other customer’s data.
- Providing additional controls for our customers’ end users; for example, ways to give end-users control over personalization preferences.
The future of privacy requires that we take these (and more) seriously and work to develop robust personalization capabilities that also safeguard customer information.
Recommend
About Joyk
Aggregate valuable and interesting links.
Joyk means Joy of geeK