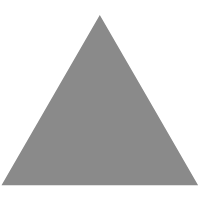
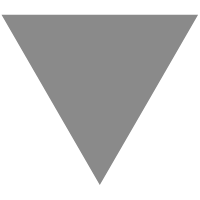
TVM-MLC LLM 调优方案 - 青铜时代的猪
source link: https://www.cnblogs.com/wanger-sjtu/p/17497249.html
Go to the source link to view the article. You can view the picture content, updated content and better typesetting reading experience. If the link is broken, please click the button below to view the snapshot at that time.
本文地址:https://www.cnblogs.com/wanger-sjtu/p/17497249.html
LLM 等GPT大模型大火以后,TVM社区推出了自己的部署方案,支持Llama,Vicuna,Dolly等模型在iOS、Android、GPU、浏览器等平台上部署运行。
https://github.com/mlc-ai/mlc-llm
本文在之前作者介绍的基础上,简要介绍一下mlc的调优部署方案。
pipeline
在正式介绍TVM mlc.ai部署LLM方案之前,首先简要介绍一下当前主流LLM的一个工作流程。
需要说明一点的是,上图中的prefill跟Decode指的的同一个模型,只是输入的shape存在差异。
这里的示意图省略了很多,只是大致描述一下pipeline。
在处理用户输入时,此时长度大小是不能确定的,这时候是完全的是一个完全的动态shape的。但在decode过程中由于是token by token的,这时候网络中的中除了kv cache相关几个部分,其他大多数的操作都是固定shape的,就可以用已有的算法调优了。
MLC.AI 部署调优方案
以下以RedPajama3B模型的tuning跟build过程介绍一下mlc的方案。
pipeline 组成
在已经支持的几个模型里面均有get_model
这个函数,在这个函数里面会创建下面4个IRModel。
- encoding_func
- decoding_func
- create_kv_cache_func
- create_softmax_func
- create_metadata_func
encoding_func
这对应了上图中的prefill过程,在每次用户输入后调用。由于用户输入的不确定性,所以这个过程基本上都是动态shape的,很难确定到底输入是多大,也不适合搜索调优。
decoding_func
这是上图中decode过程的一部分,因为这个过程是token by token的,在计算过程中大部分的计算是固定shape的。
create kv cache func
这里是直接调用的relax.vm
中的函数,创建的是kv cache的存储相关。
create softmax func
这个也是解码过程的一部分,确切的说是采样过程中计算的一部分
** create_metadata_func **
模型的meta信息,比如model_name
、stop_tokens
等
构建完以后,就进入到优化的阶段了。下面根据build.py过程描述一下过程。
-
API构图构建了相关的模型,读取权重
-
优化PASS
- FuseTransposeMatmul
- FuseDecodeMatmulEwise
- DeadCodeElimination
- LiftTransformParams
- split_transform_deploy_mod
-
Codegen 生成代码
- DispatchTIROperatorAdreno/DispatchTIROperator/DefaultGPUSchedule 手动优化的sch
- MetaScheduleApplyDatabase搜索的log生成固定shape的sch
Tuning
在MLC-LLM的代码仓里面已经提供了tuning的脚本,有一点需要先做一下,先调用build.py的文件,把静态shape的相关的函数分离出来。就得到了tuning文件中需要的mod_tir_static.py
__EOF__
Recommend
About Joyk
Aggregate valuable and interesting links.
Joyk means Joy of geeK