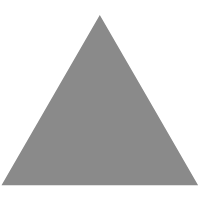
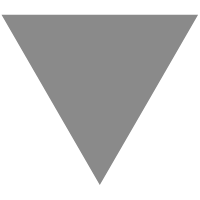
Azeem’s Picks: Demis Hassabis on DeepMind’s Journey from Games to Fundamental Sc...
source link: https://hbr.org/podcast/2023/05/azeems-picks-demis-hassabis-on-deepminds-journey-from-games-to-fundamental-science
Go to the source link to view the article. You can view the picture content, updated content and better typesetting reading experience. If the link is broken, please click the button below to view the snapshot at that time.
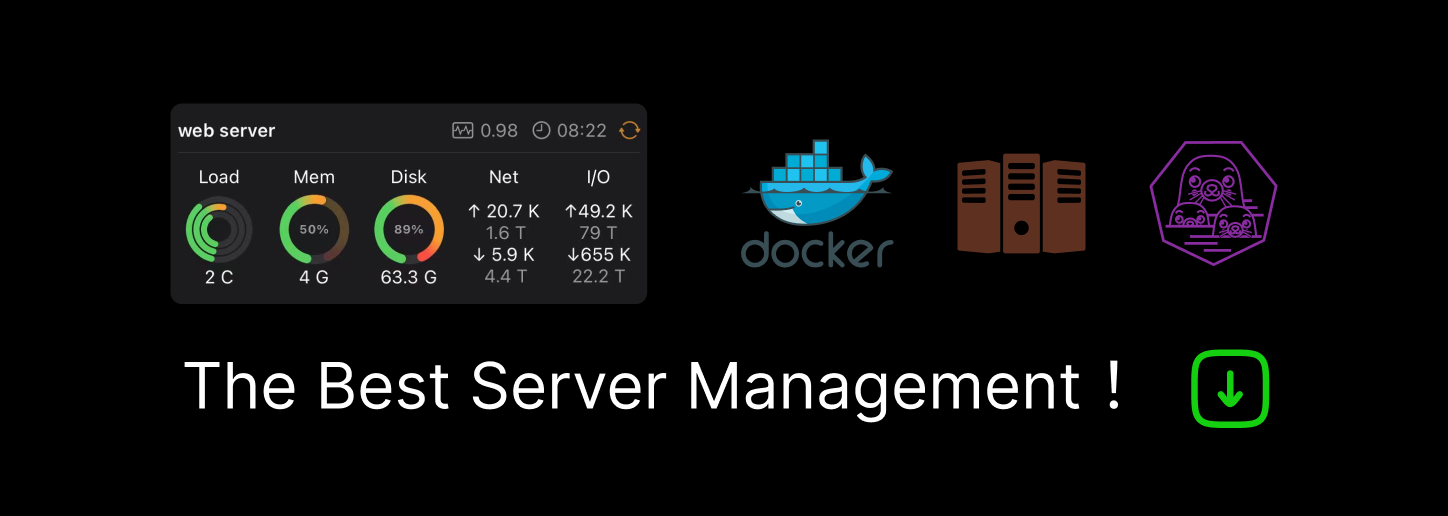
Demis Hassabis on DeepMind’s Journey from Games to Fundamental Science May 05, 2023
Artificial intelligence (AI) is dominating the headlines, but it’s not a new topic here on Exponential View. This week and next, Azeem Azhar shares his favorite conversations with AI pioneers. Their work and insights are more relevant than ever.
Demis Hassabis, CEO and co-founder of DeepMind, dreams of using AI to solve fundamental problems in science. In 2020, he joined Azeem to explore his own journey from world champion gamer to neuroscientist to building AI systems that can train themselves to solve real-world engineering challenges and, eventually, make Nobel-prize winning discoveries.
In 2023, DeepMind’s parent company Alphabet announced consolidation of its biggest research units, DeepMind and Google Brain, into a new division led by Demis.
They also discuss:
@demishassabis @azeem @exponentialview
AZEEM AZHAR: Hello, I’m Azeem Azhar. Eight years ago I started Exponential View to explore the underlying forces driving the development of exponential technologies. I’m a child of the Exponential Age, born the year after Intel released the 4,004, the world’s first single chip microprocessor. I got my first computer in 1981. I’m holding it as I speak. And as I moved into adulthood, the internet was growing exponentially, transforming from a tool of academia to the worldwide utility it is today. I, at least, did not grow exponentially with it. Now, back in 2015 when I started writing Exponential View, I’d noticed something curious was going on. You could feel the pace picking up. New technologies were clearly on some kind of accelerating trend. One of those technologies is artificial intelligence. So today I’m bringing back one of my all-time favorite discussions on AI and that was with the brilliant Demis Hassabis, the co-founder and CEO of DeepMind. And he’s recently taken on a new role within Google as the head of Google DeepMind, which combines both his old DeepMind organization and the Google Brain Organization. Now, in the conversation I had with Demis, a couple of years ago, we talked about AI and the scientific revolution that it’s sparking. It really captures his vision and it continues to inspire me years later. For those of you who might not be familiar, DeepMind is a powerhouse AI lab which brought us groundbreaking projects like AlphaGo, the first computer program to beat a go world champion and AlphaFold, a system for predicting protein 3D structures. That’s transforming our understanding of one of the eternal mysteries of science, protein discovery. Of course, DeepMind does so much more and is also at the forefront of large language models and many, many other AI techniques. It’s a fantastic conversation with an amazing human. Please enjoy this rerun. Demis, welcome to Exponential View.
DEMIS HASSABIS: Thanks for having me.
AZEEM AZHAR: Now, you’ve got a long history with games and game playing. You were a chess prodigy, a video games designer, a mind sports champion, the Bjorn Borg of mind games, I like to think of you as. How did your love affair with games get started and what is it about them that thrills you?
DEMIS HASSABIS: It started very early actually. So, I was about four years old when, I don’t remember this, but my father tells me about this that I saw him play my uncle … neither of them are very good at chess, but they were just sort of playing for fun. And apparently I asked them could they teach me and they humored me and taught me chess. And then a couple of weeks later, I was beating both of them. So then I think my dad just thought, well, maybe he should take me to the local club. And I remember very vividly, it was in a sort of old shack, and went there and started winning lots of the junior tournaments there, representing London and the county and then eventually nationals. And sort of took off from there. So, games have always been a part of my life. And the reason I like them is, I think it’s great training for the mind. So board games especially, I think of it as a little bit like going to the gym. But in this case you’re training the mind. It’s just a muscle in a way. And so, I think I actually would be in favor of putting on the school curriculum where I think it teaches you about planning, strategizing, visualization, imagination. All these things, I think are sort of trained, dealing with pressure, time pressure. For example, things like exams become quite easy because the stress of them seems actually a lot, weirdly, it’s a lot less than it was when you were playing for the championship in the final of one of these chess tournaments. And then, of course I got into game design. So there I combined my love of computer games, which I’d started playing and programming, along with my love of sort of board games. And it was a natural kind of connection. And the cool thing about video games is, especially in the nineties when I was heavily involved in making them, is that they’re often, at the most cutting edge of technology-
AZEEM AZHAR: That’s right.
DEMIS HASSABIS: So, hardware and software techniques actually often are applied first in games, even GPUs for example, which we use now in AI, were invented for games first.
AZEEM AZHAR: Let’s go back to that crucial period where you were at school and you did something that, I guess, for someone whose family is sort of reasonably new to the UK, it’s quite a brave thing. Because you didn’t go straight to university, you actually went into games design. You worked with the legendary British games designer, Peter Monahue. You worked on a game that nearly knocked my A Level revision off course, which was called Populous. I still remember the soundtrack, that soundtrack in the background. How did that decision come about?
DEMIS HASSABIS: I finished A Levels at 16, so I had quite a lot of time between then and going to Cambridge. They wouldn’t let me go till you were the right age to 18. And instead of traveling around the world for a year or two, I decided to straight away go and do some work, literally the day after my A Levels. And the reason I did this, is this game Populace, which you got obsessed with it sounds like as well was my favorite game. And I was about 12, 13 I think when it came out. And it was a fascinating game because it was a simulation game. It was all these little people in the game and they had AI behind them controlling what they did. And it was a fascinating insight into what might be possible with games. And I tried writing my own things that were like that. And then when I got the chance to work at the place that built that game, I couldn’t resist. I spent nearly two years there and we ended up writing Theme Park, which was the biggest game I’ve sort of worked on as a main contributor and the lead programmer. And that went on to sell 10 billion copies and sort of spawn a whole genre of sandbox simulation games they’re called. So it was a fascinating moment, but yeah, it was very important as my formative years, but my parents, I think, by that point had completely lost track of what I was doing, to be honest.
AZEEM AZHAR: Right. Games have been an important part of the development of artificial intelligence all the way back to the first Checkers draft playing program back in the fifties or perhaps even earlier with the mechanical Turk, a couple of hundred years before that. What is it about games that makes them relevant to the field of AI research?
DEMIS HASSABIS: Games is part of the AI field. It’s as old as the AI field is itself. I mean if you look back at Turing or Shannon or the sort of founding people that kind of created the field, they all wrote chess programs. In fact, there’s a famous one by Turing where he wrote his first chess program on a piece of paper and he had to be the computer. He executed the instructions and played the game. I think it took him a week to play one game. But so there was sort of chess programs before there were even computers in a way. And really for me, it’s like the third chapter of using games in my life. So the first one was learning how to play it, training the mind. Second was designing them, and third, it was obvious to me, we should go back to that heritage when we started DeepMind of using games as a platform, a convenient platform to quickly test out AI algorithmic ideas. And one reason, I mean there are many reasons why it’s convenient. One is that obviously most games have a clear objective. They have a score. So it’s quite a nice reward signal for your reinforcement learning system, your AI system, to learn from and to optimize. And so it’s pretty convenient from that perspective. Another thing is, when we were a small startup, we didn’t have access to a lot of data from applications or whatever. And so, we had to synthesize our own data and obviously if we use games you can … whether that’s board games go or simulations like computer games, video games, you can run them for as long as you like and generate as much synthetic data as you want. So for example, with AlphaGo, AlphaGo played itself, I think over 10 million times. So you don’t have to get that data from anyway. It can generate that data yourself. And we felt that if we didn’t give the AI sort of privileged access to the insides of the game, but it had to learn for itself just like a human would have to do, then we felt that you could make genuine progress with AI using games. And I think that the danger with using games is always that you could delude yourself into learning about something when in fact you’ve you’ve given the AI system all the underlying details that the game’s using. So you have to be very careful about that.
AZEEM AZHAR: And so that’s a distinction, in a sense, between the approach that DeepMind took with its game playing and say Deep Blue, which had beaten Gary Kasparov of in 1997, where it had millions and millions of previously played human games and a set of quite well-crafted rules and decision systems that would work its way through. Whereas, your systems in a sense, learnt from the experience that they had had of playing games.
DEMIS HASSABIS: That’s right. That’s the big distinction between what we’ve been doing with DeepMind since 2010, and things like AlphaGo and as you say, very famous programs like Deep Blue where Deep Blue was an amalgamation of chess grand masters and very smart programmers with a super computer. And instead of that, if you look at AlphaGo, it completely learns everything from first principles. So by playing against itself many thousands or hundreds of thousands of times, and then learning the strategies for itself. So it’s a lot more like how a human would learn how to play chess or go. And then the other thing is there was narrow AI systems, of course, I used to program them for computer games, you have bots and opponents and so on, in computer games. What we were after, with our systems, is actually inferring where you are standing in a room by what you can see around you. So much more like a biological system, an animal or human would do and that we felt would be, would then generalize to all sorts of other things that you could do with it beyond games.
AZEEM AZHAR: I think that distinction is quite an important one. If we think about the AI’s that you programmed, what kind of knowledge of its environment did those systems have? What kind of discretion did they actually have? The way it’s often joked about that sort of before deep learning came along, AI was really lots of if then, else statements.
DEMIS HASSABIS: Yeah, of course that’s an oversimplification, but it’s definitely the case that it was full of logic systems. It’s really big databases joined together by a lot of logic rules. So the idea is that, in this case, you don’t give the machine anything that is sort of privileged information, let’s call it. So we just gave it the pixels on the screen. So 30,000 numbers, and then the score, and that was it. And then the system was not told how to play the rules of the game, what the controls did, nothing like that, what the pixel on the screen represented. You had to work out all of that from first principles, with the only objective being to, in this case, to maximize the score, as the only guide being principle. And what was fascinating, I think what we proved, which was a big breakthrough, with combining deep learning, which in effect you can think of as figuring out what’s on the screen, and then the reinforcement learning part, which is a goal optimization algorithm which effectively tries to hill climb towards being better and better at the objective, whatever objective you’ve given it. Back in 2012, when we were first doing this, we were worried that … let’s take the classic game pong … we were worried could we even get one point against the inbuilt AI system and for ages, for months, we couldn’t even get one point. And so it was a huge challenge at the time. No one was sure it’d be possible to do.
AZEEM AZHAR: You’ve introduced a number of really interesting concepts there, and let me see if I can slowly break them back down. So in a sense, you’ve got an intelligent agent and if it takes a good action that helps, it gets to its objective, it gets some kind of positive reinforcement. And if it takes the wrong kind of action, it gets a negative reinforcement perhaps, meaning that the game ends. And that you described two different systems you needed. One was a deep learning system that was the way in which the agent understood its environment, looking at the pixels on screen. And then the second was the reinforcement learning mechanism, which is a kind of Pavlovian trigger that would say to the algorithm, you climb that hill and the bones that you want to know on at the top of the hill.
DEMIS HASSABIS: That’s exactly right. In fact, one of the things that Shane Leg, my co-founder and chief scientist did for his PhD was to analyze a system called AIXI, Aixi, it’s sometimes pronounced. And it’s actually an amazing mathematical framework for what intelligence is and it’s still not that well known. It’s fascinating. And what it shows you, is that what you need for intelligence, in theory, is a system that can model the world around you and that’s the deep learning system. And then you need some sort of optimization algorithm that will kind of hill climb towards whatever goal you’ve got in mind. And that’s the reinforcement learning part. And of course, there are all sorts of caveats that you have to have infinite compute, infinite memory and so on. It’s not as that’s solved AI, but at least in the limit, we know that that’s enough, fully generalized intelligence. And then the other way we can look at it is from a more biological point of view. We know that the brain uses reinforcement learning. It’s the dopamine neurons in the brain. You say Pavlovian, all animals do that, including humans. We learn from reward, and if it’s something that’s painful, we shy away from doing those actions. And of course it’s a very powerful learning algorithm. And then on top of that, a big part of our brain like visual cortex, auditory cortex and so on … that’s a bit like the deep learning systems today in the sense of, that’s how we process this stream of sensory to input that we get all the time and we try and make sense of it and find patterns in that. And our brains are extraordinarily good at doing that. And then combining it with this reinforcement learning kind of goal optimization mechanism. So if you look at neuroscience or you look at mathematics, you find that they’re sort of pointing at the same answer, which is why we then were so confident that deep reinforcement learning, as we call it, would ultimately work out.
AZEEM AZHAR: You’ve had such great success with it. You’ve also created some amazing demonstrators that help illustrate some of the issues that we will face with artificial intelligence. So one of my favorites is in one of your Atari games. It’s the breakout game, and just for listeners, this is the game where you have a set of bricks at the top and you bounce a ball against a paddle. It was available on the Atari system and on Black Breeze and you have to break your way through the blocks and it gets faster and faster. And Demis’ system learnt that the thing to do is to make a tiny little tunnel on the left-hand side, fire the ball up through that, and then let it just iterate between the ceiling and the bricks and you sort of win and it’s superhuman. I mean literally it’s superhuman because humans can’t play that way.
DEMIS HASSABIS: Yeah, I think that actually shows the creative potential of AI in this particular case. For us, that was really the first wow moment because we’d … it was the first time that I would say that the AI system discovered something that we hadn’t anticipated or thought was possible and then realized that what it had found was a more efficient solution to the problem. And so, I think that is exactly what we want from our AI systems and I think it’s the kind of thing that only the types of learning algorithms that we are building now and the now in vogue are capable of doing. Because if you contrast that to the old school logic based systems that we discussed earlier, one of the problems with them, is that they can’t really go beyond what we as the human creators of those systems know how to do because obviously we’ve had to completely encapsulate that knowledge in a whole bunch of quite rigid rules. In effect, it’s constrained. It can only brute force execute those logic rules. It can’t ever learn anything new or add to that knowledge base. You can’t get out of that system anything more than what you already put in. But that’s what I think the promise of these kinds of new AI systems is, we could use it to make breakthroughs that we ourselves don’t know how to do.
AZEEM AZHAR: So, in that sense, these old-fashioned AI systems were exceptional at exploitation, but what they weren’t so good at was this question of exploration.
DEMIS HASSABIS: But it’s a bigger issue than that. It turns out, that we’re quite bad at expressing what we already intuitively understand, but it’s not a natural state for us to create mathematical rules for lots of very complex, messy things in the world. So I’ll give you an example. Computer vision. There were lots of handcrafted systems that were built on theories of how vision worked and were trying to kind of classify … well maybe we see shapes and then perhaps we see parts of objects and then lines and then people tried to effectively code those up. And it turns out that we’re not actually that good at describing how we see in logic statements.
AZEEM AZHAR: Before deep learning came around, machine vision systems, I think the technical terminals were terrible. And then in about 2010, 2011, the first deep learning systems showed up running on these GPU’s, graphics processing units that had come out of the games industry and the potential of this technology became visible, in the sense it’s what kicked off the deep learning investment wave that we’ve sort of seen take hold of the world over the last de decade. I’m curious though, about this question that you raise about learning in the environment and things that can’t be codified. So that raises two different questions. So one is, I can understand within a game how you can give a machine many, many different experiences of the world. But in the real world, where we operate, it’s quite difficult to simulate that in any great detail. And it’s also expensive. So I have this question about the extent to which you feel that we need to physically locate our artificial intelligence for them to be able to learn a useful class of intelligence or whether we can get to useful classes of intelligence without doing that.
DEMIS HASSABIS: So this is a sort of quite heavily debated point actually in the field of AI. So you’re right, that might be expensive or slow or just not feasible to generate the kind of data that you might need. And that’s why data efficiency and things like transfer learning where you transfer your knowledge from one domain to a new domain. Humans do this all the time. When we learn a new task, we bring to bear our library of knowledge from other tasks, and we make analogies-
AZEEM AZHAR: That’s right.
DEMIS HASSABIS: And computers, or AI systems are pretty bad still at doing that. That’s one of the big things we’re working on. Transfer learning. And today these deep learning systems and deep reinforcement learning systems often need millions of examples before they’ll be good at something. Whereas, really what we would like is some is a system that can learn from perhaps a few dozen examples and then humans certainly can do that. So we have a lot of projects that are working on that. So it’s a very active topic at the moment. The other question about embodiment. We feel like AI systems have to learn from first principles. They’d have to be grounded in the sensory environment that they find themselves in. And we think that’s important because what they used to do is build databases where they said a dog had four legs and a dog barks and a dog chases cats. And these are all logic rules. But what you need is actually the AI system to learn about these concepts about a dog directly from the experience because that way you are connected all the way through. You’re grounded all the way through. Now that’s sometimes called embodied intelligence. Now there were a lot of people that subscribed to this point of view back in the nineties and early two thousands and almost all of them worked on robots because of course, a physical robot by definition, is embodied, is situated in its environment physically like we are. Now but the problem with robots and why we went for virtual games rather than physical robots is that they’re expensive and they’re slow. And if you talk to any roboticists, certainly back in the early two thousands, they’ll tell you, they spend all their time fixing the motors and the wheels and they never even get to address the question of intelligence because they’re dealing with the physical difficulties of building the robot. And we wanted to avoid all of that, but still get the benefit of being grounded in a sensory motor stream. So for us, again, the perfect solution was games, virtual games, and virtual environments. So they were embodied but in this virtual world.
AZEEM AZHAR: Clearly you’ve come from this set set of disciplines that is actually quite interdisciplinary. You’ve talked about neuroscience and you’ve talked about computer science and traditional AI and bringing them together. You’ve talked about your experience of using games as the sandboxes where you developed these intelligences. But of course you’ve taken the work beyond problems of gaming. The company was acquired by Google a few years ago and then within a couple of years we heard that DeepMind systems were being used to improve power efficiency within Google’s data centers. How do you go from beating the world’s best go player to power management within a data center?
DEMIS HASSABIS: It was never, obviously, our intent to just focus only on games. Games were the gateway for us to building and improving out these general purpose algorithms that could learn in AlphaZero’s case for any, to play a game, from scratch without any of the rules or anything. And so we thought once we were to, if we were to crack games in that general way, we should be able to apply that algorithm to real world problems that have the same type of properties, if you like. And we’ve started looking for that type of problem, both within Google and externally. One of the earliest ones was the one you mentioned about actually controlling all the cooling systems in a data center. It’s a large amount of the cost of running a data center and we were able to save 30% of the energy used by just more efficiently controlling hundreds of different mechanisms there are in a modern day data center. Since then, we’ve taken that further to building controls through various partners through Google Cloud. Things like air conditioning and lifts and heating and all of that stuff. We can make them a lot more efficient, too. I even think it could be applied at a grid scale and we’d love to try that at some point and save energy at a national scale. So that was a huge big project for us and since, but since then we’ve put it into many other things. So android battery life, like saving your battery life near when it starts getting low by running things on your phone in more efficient ways. Very similar actually to the algorithm for the data center. So there’s a vast number of … I think we’re now in dozens and dozens of products, sort of under the hood within Google, where actually there’s some DeepMind technology powering some aspect of it.
AZEEM AZHAR: One of the interesting areas that you have written about has been how these techniques can be applied to the general problem of scientific discovery. And you even wrote this phrase, will this usher in a new wave of scientific discovery help us connect the dots between maximizing the efficiency of the systems in a data center through to something that is going to take us beyond bacon and the scientific method.
DEMIS HASSABIS: So, this is actually my absolute passion and in fact the reason, my personal reason why I’ve been working on AI is from my whole career, is that getting to the point actually, which is very exciting, where we have powerful enough algorithms that we might be within reach now of using them on the scientific method itself and actually helping accelerate scientific discovery. And I think in the next 10 years, that’s where I’m really excited on a personal level, to see what’s going to happen. And I predict there’s going to be a lot of big major, perhaps Nobel prize level type of breakthroughs, in all sorts of domains. And I think there’s two keys to it. One is there’s the sort of pattern matching that we’ve discussed earlier. So in modern day science, if you look at the large hat on Collider or genomics or any of these domains, the amount of data that is sort of generated experimentally is just mind-boggling and it’s so big, no single human or even the smartest scientists, they can’t hold all of that in mind at once. So, it’s very difficult to see all the patterns and the structures that might be hidden in that amount of data. And so, I definitely think AI, clearly can help with that. That’s what deep learning’s amazing at, is finding those patterns. Effectively, I see building AI as a general purpose Hubble telescope. Something that allows us as scientists to see further and deeper into the mysteries of the universe. That’s on a high level of what I actually think the endeavor that we’re on at DeepMind is about. And I can’t think of anything more exciting. I think in the end, AI will be this phenomenal tool for understanding the world around us. But the second part that’s, I think, going to come after that, is the bit we discussed about breakout and also AlphaGo, where it discovered all sorts of new motifs in this 3000 year old game go. If you think about that, that’s the germ of an idea that you get in a scientific breakthrough. It’s like here’s the orthodoxy of how to look at something or deal with a particular domain and then, the AI system was able to come up with a totally new idea, that turned out afterwards when the human experts analyzed it to be a breakthrough idea. And so, I just feel like there’s no reason we couldn’t have that kind of moment in scientific and medical fields in particular domains. And that’s what we are working towards.
AZEEM AZHAR: So, at the moment we talk about AI helping build better tools, which may be a better research assistant that makes me think of Stanley Edington who took his telescopes to bits of the earth to observe an eclipse, to provide empirical validation for an idea that Albert Einstein who didn’t have the tools, but have his imagination had come up with sort of 25 years ago. And what I’m hearing from you though is that we’re just starting to figure out those Einstein moments.
DEMIS HASSABIS: I think that what humans are … we’re tool making animals. I think that’s actually the core distinction about what we do. And we’ve always used these tools from fire onwards to shape the world around us and create modern civilization. AI though, it could be sort of the ultimate tool because it’s a general purpose tool that could be applied to many different fields of science. And so that’s the potential power is generality.
AZEEM AZHAR: I’m curious about how you think we get there. There’s this phrase, you can’t build a ladder to the moon, which is this notion that essentially getting to the moon requires a really different paradigm and that paradigm itself has a whole set of sub-components that need independent development to your ladder building skills. A lot of AI research, especially as it’s happening within the larger companies, whether it’s Google or Facebook or the sort of Microsoft partnership with Open Ai seems to me, to be happening in similar directions. We’ve got deep neural networks strung together in different ways and we’ve got lots of compute. It seems like everyone is using the same set of ingredients. Will those ingredients give us that breakthrough that we need or do you think we need a new paradigm?
DEMIS HASSABIS: I think one of the reasons it looks from the outside that these sort of industrial labs or academic labs are all going in the same direction, is because currently that’s the thing that works … those sorts of deep learning and reinforcement learning and it’s still relatively new. So everyone’s scaling that up and applying it and seeing the limits of that. And, of course, once some people start pioneering that, others quickly follow and that’s how science works. Now there are some people, including some eminent scientists, that feel like we have all we need. We just scale that lab and we’ll get to the moon. Right? There are definitely people who believe that, yeah, I’m not really in that camp. I suspect that when we look back on all this in many years’ time, that some other breakthroughs were required perhaps in some of the things we’ve discussed, to get transfer learning or data efficient learning, this kind of thing. There may be other computational aspects that are needed and at DeepMind, at least we are a very broad church. In fact, if you take AlphaGo, for us that was like 20 people. We have many hundreds of researchers. So if you can see just proportionally even. That’s still tip of the iceberg. What happens underneath, below the sea is the majority of the work going on and a lot of that is very, very exploratory and very interdisciplinary. We have physicists and neuroscientists and mathematicians and psychologists, as well as obviously machine learning people and engineers. We intentionally make that a very rich interdisciplinary environment so that we don’t fall into groupthink. So in fact, we are in the fortunate position at deep mine being big enough, we can push the sort of exploration of new components whilst scaling up to the limit, the current components. And obviously they’re complimentary because if you push to the current components, that tells you where you really need to focus your sort of exploratory efforts.
AZEEM AZHAR: So, you have to explore and exploit at the same time. What does that balance look like? And how do you go about assessing whether you are getting that balance between scaling the stuff that works and these high risk sort of potentially high payouts explorations?
DEMIS HASSABIS: Yeah, it’s a tricky thing to get right as you might imagine. And it’s funny. What you are talking about, this exploitation, exploration problem is actually the crux of the problem of AI, in a sense like face this issue. And a company that likewise is a sort of an organism. And so what we’ve done is, we sort of have a loosely a 70-20-10 rule, which is that 70% of the work we do and people and resources are on the core sort of multi-year objectives and quite clear sort of demonstrations of that. 20% is sort of more free form exploration, but still in the kind of approved set of topics let’s say. And then we have 10% that’s just literally almost orthogonal bets. And we have people like that who don’t like deep learning and think it’s just a sort of flash in the pan. And they work on all sorts of other exotic things and we let them do that. We encourage that. And every now and again, a brilliant idea comes out of that and we incorporate that into the main body. So loosely speaking, it’s not hard and fast sort of rules, but that’s sort of how we look at it as a portfolio of things.
AZEEM AZHAR: So, when you started DeepMind it was you and Mustafa Solomon and Shane Leg and you are now 300 times the size. You’ve been a very successful organism in that sense. How have you gone through the process of growing your own style? Because presumably now, you lead people who lead people who write papers and do research.
DEMIS HASSABIS: It is very different. And that is a challenge, actually growing with that. And managing scientific innovation at this scale is hard. And a lot of the skills as you can imagine that required early days are different from the ones you need when you are at the scale we’re at now, a thousand plus researchers and engineers. So, I don’t think many people or many organizations have ever attempted to do sort of cutting edge research, let’s say, at this scale. So a lot of the time we’re inventing our own processes to manage that and project management systems and protocols. And so what we tried to do is synthesize the drive and energy you get from the best startups, and then combining that with blue sky creativity that you get in the best academic labs. And what we wanted to do with DeepMind, was kind of combine the best of those two worlds together. And I feel like we’ve done that. There are some places in history that we’ve taken some inspiration from Bell Labs in its heyday. Pixar, Apple in its glory days, and the Apollo project. And we’ve sort of tried to incorporate aspects of all of that into the environment at DeepMind. We have this sort of spirit we call kaizen of continually striving to improve. And so we’re always learning. So the whole organism, including myself, we are never stationary. We’re always dynamically trying to learn as well as building systems. They’re also trying to do that.
AZEEM AZHAR: When we look at some of these antecedent labs that have been very successful, they’re all largely American. They come from a kind of particular cultural background. We live in a much more global world, and one of the questions that is raised around AI, is how does it fit within the context of different cultures, different epistemologies, different pedagogies.
DEMIS HASSABIS: This is something we actually think about a lot, and we have from the beginning of DeepMind, is the sort of ethics and responsibility issues around AI. We always planned to be successful, even back in 2010 when no one was working on AI, especially not in industry. And we still even then thought, well look, if we’re successful with our mission, then it’s going to be one of the most important technologies ever invented. And like all technologies, we have to make sure that it’s used responsibly. And I think this is even more pressing for AI because it has the potential to be so general. One of the big things we’ve been focusing on, actually, for last few years is diversity and inclusion. And of course, that’s important as a moral thing anyway. Also, there’s been lots of studies on how more diverse environments are more creative and more innovative. So, clearly as a business that’s involved in innovation, we need to have that. Because of the mission that we’re on of building an artificial general intelligence, that no matter how you build those systems and how much they learn for themselves, there’s always going to be some imprint of the designers in that system, whether that’s the values or the goal, or there’ll be some input. And so therefore we need to make sure that when we come to design those systems, the there’s as wide a number of voices around the table as possible. So thinking about how those systems should be used and deployed, how they’ll affect different groups of people, and what kind of underlying values we should imbue these systems with. And that means both the system, but also society.
AZEEM AZHAR: So, how should the wider elements of society engage in with the developments that go on within DeepMind?
DEMIS HASSABIS: So, I’ve talked to lots of different government officials at various times about come to us and discuss this of all sorts of different countries, actually. And the thing I would say is, the best place to intercept that, is at when research is about to become applications. And that’s also, conveniently, the easiest point to encapsulate what you want to do. Existing regulations and rules. They’re already very good. Say around health or transport, or whatever and just make sure they are fit for purpose, for this coming new technology. And it’s been done before. Those regulations had to be updated for Internet, digital, mobile. So that’s happened in the last 20 years, and it just needs to happen again to deal with an AI systems. And this includes, by the way, educating the general users of these systems to make sure that they understand that these are not magic devices that can just do anything and what are the right checks and balances on those systems. I’m a big advocate of human in the loop for the final decision making step.
AZEEM AZHAR: Normally when you look at those sorts of situations, what you do is, you have a set of rules that have to be complied with, the obligation of compliance resides with the institution that produces them, and then there is some enforcement mechanism that exists, whether it’s a sort of a regulator or something else. So do you imagine, and would you be supportive of a structure like that emerging within the AI domain?
DEMIS HASSABIS: I think it’s still too early to say. I think it needs to be very thoughtfully done, whatever happens. So, the worst thing that could happen is some kind of knee-jerk reaction because AI is a global endeavor now, and every major country is investing in research in it. They’re all going as fast as possible. So there are a lot of complications that come with that. And one of the things I would say, is if you are going to agree something, some kind of rules of the road, it needs to be global really, otherwise it’s sort of meaningless because especially as these things are digital. Applications can be used anywhere around the world. So, it doesn’t help if the UK does something on its own, but the US doesn’t do that, or Canada doesn’t do that, or China doesn’t do that. So, that then that obviously is a huge geopolitical challenge, which we have on many levels is that’s problematic. And so I don’t know how to answer that. But that’s the note of caution I would say. And the other thing is, we need to make sure that we don’t stifle that innovation while still being cautious about how these things should be applied to make sure that this technology benefits everyone in society, which is obviously what I believe is going to happen and hope’s going to happen. And that’s why I spent my whole career and whole life working on AI.
AZEEM AZHAR: Which brings me to the last question then, which is that if you had a dream about the most positive impact that DeepMind could realize within 10 years for the UK or for the world, what would that be?
DEMIS HASSABIS: My dream, actually, I dream about every night is, every day is that that we in the next 10 years, DeepMind is part of cracking a really fundamental problem in science, scientific area, be that biology or chemistry or physics, that unlocks a whole new era of possibilities. So that would be my dream result over the next decade. Some really fundamental kind of Nobel Prize level, long-standing ground challenge in science, that then has this knock on effect of unlocking a whole bunch of potential for the good of society.
AZEEM AZHAR: Demis, it’s been fantastic to talk to you today. Thanks for taking the time.
DEMIS HASSABIS: A real pleasure. Thank you.
AZEEM AZHAR: Well, thank you for listening. If you enjoyed this conversation, be sure to check out some of the other episodes that are featuring really interesting figures from the artificial intelligence world. We’ve had Sam Altman, the CEO of another industrial research lab, Open AI, brilliant thinkers like Faith Alee, Gary Marcus, Joanna Bryson, Jürgen Schmidhuber, Stuart Russell, Andrew Ng, Danny Lange, and so many more. So please do go off and listen to those conversations as well. To stay in touch, subscribe to my podcast or my [email protected]. Five star reviews go a very long way. I would love one from you. This podcast was produced by Marija Gavrilov and Fred Casella. Ilan Goodman is our assistant producer, Bojan Sabioncello, the Sound Editor. Thanks to Premium Exponential View members, Raphael Kaufman, Gianni Decamelli, and Elizabeth Ling for their help with this discussion. Exponential View is a production of E to the Pi Eye Plus One Limited.
Recommend
-
68
GitHub is where people build software. More than 27 million people use GitHub to discover, fork, and contribute to over 80 million projects.
-
44
A list of fundamental web development resources that will increase your understanding of the Web, and progress your career.
-
4
In this #mtpcon London+EMEA keynote, entrepreneur, investor, and author of
-
10
In Conversation with Azeem Azhar, Author, The Exponential Age For all the excitement about the explosive pace of progress in AI and technology that many readers of this blog will share, there’s an undeniable feeling of un...
-
1
-
3
Will Large Language Models Reshape Our Economies? You have 1 free article left this month. ...
-
3
What Can the Copernican Revolution Teach Us about the Future of AI? You have 1 free article left this month. ...
-
5
Sam Altman, Geoffrey Hinton, Demis Hassabis And Others Warn Against Risk Of Extinction Due To AI In A New Open Letter News
-
3
DeepMind made its AI name in games. Now it’s playing with the foundations of computing...
-
4
A Founder’s Exponential Toolset with Azeem Azhar & James Currier | The NFX PodcastIn this enlightening conversation, Azeem Azhar, a former VC-backed Founder and author of ‘The Exponential Age’, shares his insights on th...
About Joyk
Aggregate valuable and interesting links.
Joyk means Joy of geeK