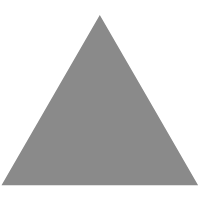
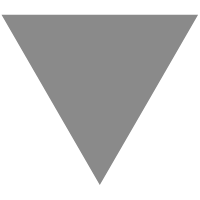
[2303.11156] Can AI-Generated Text be Reliably Detected?
source link: https://arxiv.org/abs/2303.11156
Go to the source link to view the article. You can view the picture content, updated content and better typesetting reading experience. If the link is broken, please click the button below to view the snapshot at that time.
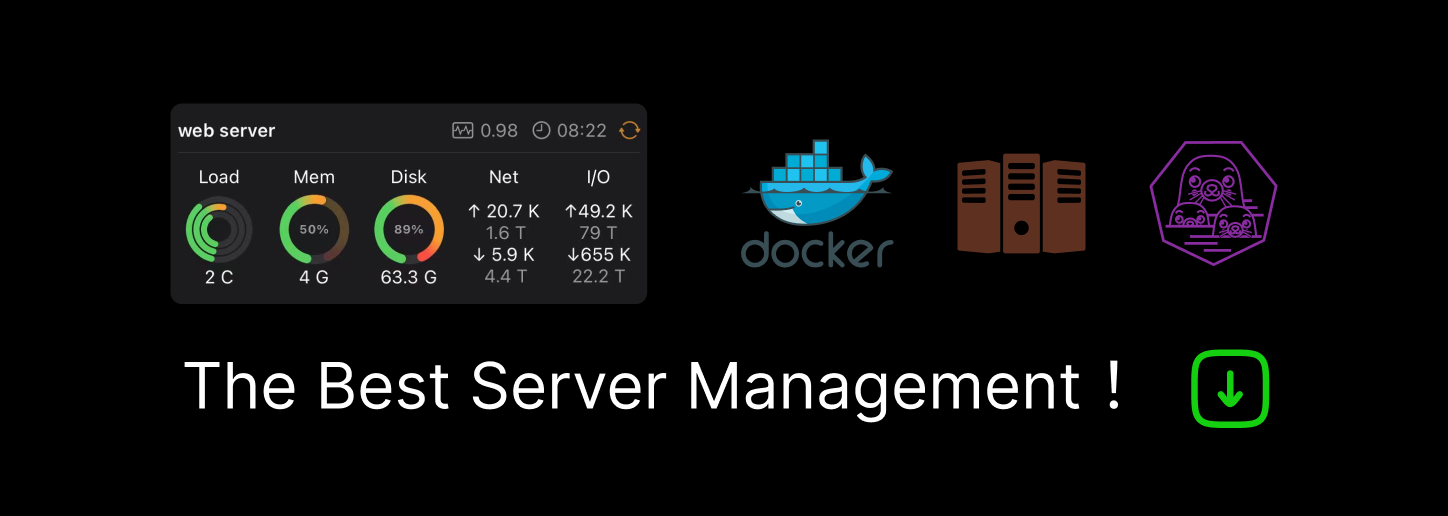
[Submitted on 17 Mar 2023]
Can AI-Generated Text be Reliably Detected?
The rapid progress of Large Language Models (LLMs) has made them capable of performing astonishingly well on various tasks including document completion and question answering. The unregulated use of these models, however, can potentially lead to malicious consequences such as plagiarism, generating fake news, spamming, etc. Therefore, reliable detection of AI-generated text can be critical to ensure the responsible use of LLMs. Recent works attempt to tackle this problem either using certain model signatures present in the generated text outputs or by applying watermarking techniques that imprint specific patterns onto them. In this paper, both empirically and theoretically, we show that these detectors are not reliable in practical scenarios. Empirically, we show that paraphrasing attacks, where a light paraphraser is applied on top of the generative text model, can break a whole range of detectors, including the ones using the watermarking schemes as well as neural network-based detectors and zero-shot classifiers. We then provide a theoretical impossibility result indicating that for a sufficiently good language model, even the best-possible detector can only perform marginally better than a random classifier. Finally, we show that even LLMs protected by watermarking schemes can be vulnerable against spoofing attacks where adversarial humans can infer hidden watermarking signatures and add them to their generated text to be detected as text generated by the LLMs, potentially causing reputational damages to their developers. We believe these results can open an honest conversation in the community regarding the ethical and reliable use of AI-generated text.
Subjects: | Computation and Language (cs.CL); Artificial Intelligence (cs.AI); Machine Learning (cs.LG) |
Cite as: | arXiv:2303.11156 [cs.CL] |
(or arXiv:2303.11156v1 [cs.CL] for this version) | |
https://doi.org/10.48550/arXiv.2303.11156 |
Recommend
About Joyk
Aggregate valuable and interesting links.
Joyk means Joy of geeK