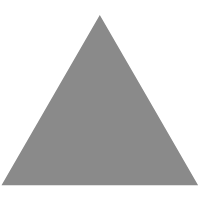
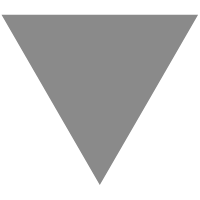
[2203.05093] Sampling from the Sherrington-Kirkpatrick Gibbs measure via algorit...
source link: https://arxiv.org/abs/2203.05093
Go to the source link to view the article. You can view the picture content, updated content and better typesetting reading experience. If the link is broken, please click the button below to view the snapshot at that time.
Mathematics > Probability
[Submitted on 10 Mar 2022]
Sampling from the Sherrington-Kirkpatrick Gibbs measure via algorithmic stochastic localization
We consider the Sherrington-Kirkpatrick model of spin glasses at high-temperature and no external field, and study the problem of sampling from the Gibbs distribution \mu in polynomial time. We prove that, for any inverse temperature \beta<1/2, there exists an algorithm with complexity O(n^2) that samples from a distribution \mu^{alg} which is close in normalized Wasserstein distance to \mu. Namely, there exists a coupling of \mu and \mu^{alg} such that if (x,x^{alg})\in\{-1,+1\}^n\times \{-1,+1\}^n is a pair drawn from this coupling, then n^{-1}\mathbb E\{||x-x^{alg}||_2^2\}=o_n(1). The best previous results, by Bauerschmidt and Bodineau and by Eldan, Koehler, and Zeitouni, implied efficient algorithms to approximately sample (under a stronger metric) for \beta<1/4.
We complement this result with a negative one, by introducing a suitable "stability" property for sampling algorithms, which is verified by many standard techniques. We prove that no stable algorithm can approximately sample for \beta>1, even under the normalized Wasserstein metric.
Our sampling method is based on an algorithmic implementation of stochastic localization, which progressively tilts the measure \mu towards a single configuration, together with an approximate message passing algorithm that is used to approximate the mean of the tilted measure.
Comments: | 41 pages |
Subjects: | Probability (math.PR); Disordered Systems and Neural Networks (cond-mat.dis-nn); Data Structures and Algorithms (cs.DS) |
Cite as: | arXiv:2203.05093 [math.PR] |
(or arXiv:2203.05093v1 [math.PR] for this version) | |
https://doi.org/10.48550/arXiv.2203.05093 |
Recommend
About Joyk
Aggregate valuable and interesting links.
Joyk means Joy of geeK