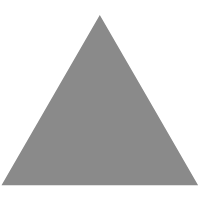
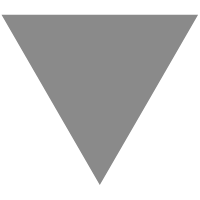
[2210.11399] Transcending Scaling Laws with 0.1% Extra Compute
source link: https://arxiv.org/abs/2210.11399
Go to the source link to view the article. You can view the picture content, updated content and better typesetting reading experience. If the link is broken, please click the button below to view the snapshot at that time.
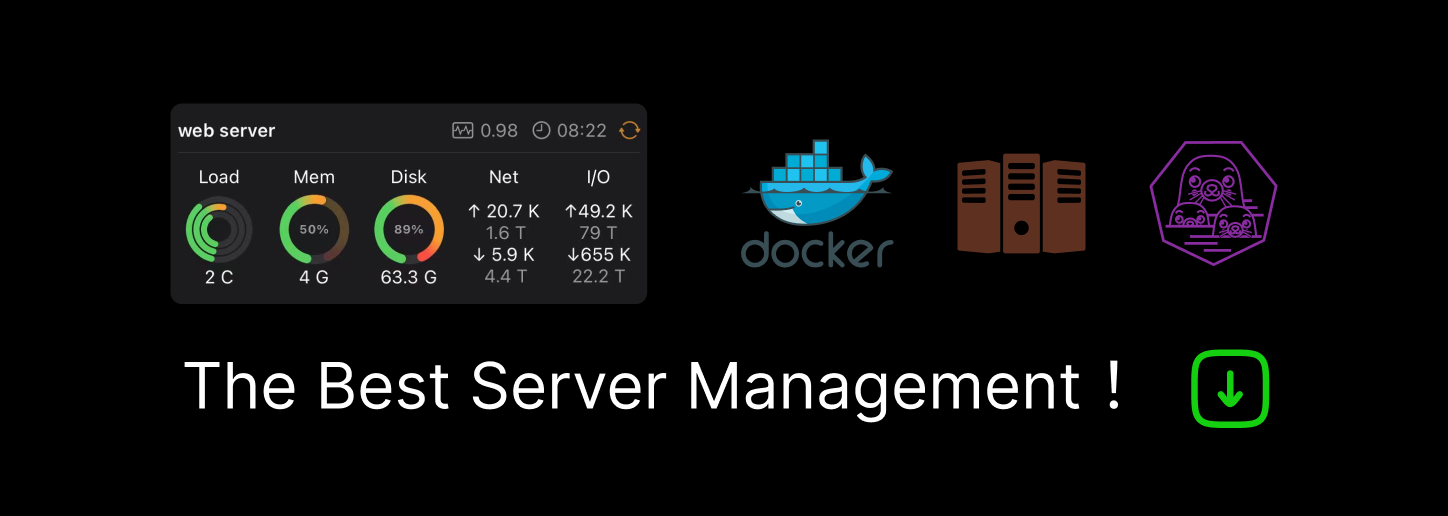
[Submitted on 20 Oct 2022 (v1), last revised 16 Nov 2022 (this version, v2)]
Transcending Scaling Laws with 0.1% Extra Compute
Scaling language models improves performance but comes with significant computational costs. This paper proposes UL2R, a method that substantially improves existing language models and their scaling curves with a relatively tiny amount of extra compute. The key idea is to continue training a state-of-the-art large language model (e.g., PaLM) on a few more steps with UL2's mixture-of-denoiser objective. We show that, with almost negligible extra computational costs and no new sources of data, we are able to substantially improve the scaling properties of large language models on downstream metrics. In this paper, we continue training PaLM with UL2R, introducing a new set of models at 8B, 62B, and 540B scale which we call U-PaLM. Impressively, at 540B scale, we show an approximately 2x computational savings rate where U-PaLM achieves the same performance as the final PaLM 540B model at around half its computational budget (i.e., saving \sim4.4 million TPUv4 hours). We further show that this improved scaling curve leads to 'emergent abilities' on challenging BIG-Bench tasks -- for instance, U-PaLM does much better than PaLM on some tasks or demonstrates better quality at much smaller scale (62B as opposed to 540B). Overall, we show that U-PaLM outperforms PaLM on many few-shot setups, i.e., English NLP tasks (e.g., commonsense reasoning, question answering), reasoning tasks with chain-of-thought (e.g., GSM8K), multilingual tasks (MGSM, TydiQA), MMLU and challenging BIG-Bench tasks. Finally, we provide qualitative examples showing the new capabilities of U-PaLM for single and multi-span infilling.
Comments: | V2 has updated references/related work |
Subjects: | Computation and Language (cs.CL); Artificial Intelligence (cs.AI); Machine Learning (cs.LG) |
Cite as: | arXiv:2210.11399 [cs.CL] |
(or arXiv:2210.11399v2 [cs.CL] for this version) | |
https://doi.org/10.48550/arXiv.2210.11399 |
Recommend
About Joyk
Aggregate valuable and interesting links.
Joyk means Joy of geeK