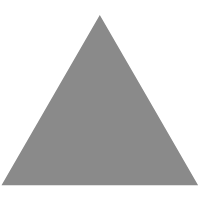
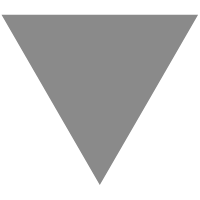
Python 大数据量文本文件高效解析方案代码实现 - 授客
source link: https://www.cnblogs.com/shouke/p/16975025.html
Go to the source link to view the article. You can view the picture content, updated content and better typesetting reading experience. If the link is broken, please click the button below to view the snapshot at that time.
大数据量文本文件高效解析方案代码实现
Python 3.6.2
Win 10 内存 8G,CPU I5 1.6 GHz
这个作品来源于一个日志解析工具的开发,这个开发过程中遇到的一个痛点,就是日志文件多,日志数据量大,解析耗时长。在这种情况下,寻思一种高效解析数据解析方案。
解决方案描述
1、采用多线程读取文件
2、采用按块读取文件替代按行读取文件
由于日志文件都是文本文件,需要读取其中每一行进行解析,所以一开始会很自然想到采用按行读取,后面发现合理配置下,按块读取,会比按行读取更高效。
按块读取来的问题就是,可能导致完整的数据行分散在不同数据块中,那怎么解决这个问题呢?解答如下:
将数据块按换行符\n
切分得到日志行列表,列表第一个元素可能是一个完整的日志行,也可能是上一个数据块末尾日志行的组成部分,列表最后一个元素可能是不完整的日志行(即下一个数据块开头日志行的组成部分),也可能是空字符串(日志块中的日志行数据全部是完整的),根据这个规律,得出以下公式,通过该公式,可以得到一个新的数据块,对该数据块二次切分,可以得到数据完整的日志行
上一个日志块首部日志行 +\n + 尾部日志行 + 下一个数据块首部日志行 + \n + 尾部日志行 + ...
3、将数据解析操作拆分为可并行解析部分和不可并行解析部分
数据解析往往涉及一些不可并行的操作,比如数据求和,最值统计等,如果不进行拆分,并行解析时势必需要添加互斥锁,避免数据覆盖,这样就会大大降低执行的效率,特别是不可并行操作占比较大的情况下。
对数据解析操作进行拆分后,可并行解析操作部分不用加锁。考虑到Python GIL的问题,不可并行解析部分替换为单进程解析。
4、采用多进程解析替代多线程解析
采用多进程解析替代多线程解析,可以避开Python GIL全局解释锁带来的执行效率问题,从而提高解析效率。
5、采用队列实现“协同”效果
引入队列机制,实现一边读取日志,一边进行数据解析:
- 日志读取线程将日志块存储到队列,解析进程从队列获取已读取日志块,执行可并行解析操作
- 并行解析操作进程将解析后的结果存储到另一个队列,另一个解析进程从队列获取数据,执行不可并行解析操作。
#!/usr/bin/env python
# -*- coding:utf-8 -*-
import re
import time
from datetime import datetime
from joblib import Parallel, delayed, parallel_backend
from collections import deque
from multiprocessing import cpu_count
import threading
class LogParser(object):
def __init__(self, chunk_size=1024*1024*10, process_num_for_log_parsing=cpu_count()):
self.log_unparsed_queue = deque() # 用于存储未解析日志
self.log_line_parsed_queue = deque() # 用于存储已解析日志行
self.is_all_files_read = False # 标识是否已读取所有日志文件
self.process_num_for_log_parsing = process_num_for_log_parsing # 并发解析日志文件进程数
self.chunk_size = chunk_size # 每次读取日志的日志块大小
self.files_read_list = [] # 存放已读取日志文件
self.log_parsing_finished = False # 标识是否完成日志解析
def read_in_chunks(self, filePath, chunk_size=1024*1024):
"""
惰性函数(生成器),用于逐块读取文件。
默认区块大小:1M
"""
with open(filePath, 'r', encoding='utf-8') as f:
while True:
chunk_data = f.read(chunk_size)
if not chunk_data:
break
yield chunk_data
def read_log_file(self, logfile_path):
'''
读取日志文件
这里假设日志文件都是文本文件,按块读取后,可按换行符进行二次切分,以便获取行日志
'''
temp_list = [] # 二次切分后,头,尾行日志可能是不完整的,所以需要将日志块头尾行日志相连接,进行拼接
for chunk in self.read_in_chunks(logfile_path, self.chunk_size):
log_chunk = chunk.split('\n')
temp_list.extend([log_chunk[0], '\n'])
temp_list.append(log_chunk[-1])
self.log_unparsed_queue.append(log_chunk[1:-1])
self.log_unparsed_queue.append(''.join(temp_list).split('\n'))
self.files_read_list.remove(logfile_path)
def start_processes_for_log_parsing(self):
'''启动日志解析进程'''
with parallel_backend("multiprocessing", n_jobs=self.process_num_for_log_parsing):
Parallel(require='sharedmem')(delayed(self.parse_logs)() for i in range(self.process_num_for_log_parsing))
self.log_parsing_finished = True
def parse_logs(self):
'''解析日志'''
method_url_re_pattern = re.compile('(HEAD|POST|GET)\s+([^\s]+?)\s+',re.DOTALL)
url_time_taken_extractor = re.compile('HTTP/1\.1.+\|(.+)\|\d+\|', re.DOTALL)
while self.log_unparsed_queue or self.files_read_list:
if not self.log_unparsed_queue:
continue
log_line_list = self.log_unparsed_queue.popleft()
for log_line in log_line_list:
#### do something with log_line
if not log_line.strip():
continue
res = method_url_re_pattern.findall(log_line)
if not res:
print('日志未匹配到请求URL,已忽略:\n%s' % log_line)
continue
method = res[0][0]
url = res[0][1].split('?')[0] # 去掉了 ?及后面的url参数
# 提取耗时
res = url_time_taken_extractor.findall(log_line)
if res:
time_taken = float(res[0])
else:
print('未从日志提取到请求耗时,已忽略日志:\n%s' % log_line)
continue
# 存储解析后的日志信息
self.log_line_parsed_queue.append({'method': method,
'url': url,
'time_taken': time_taken,
})
def collect_statistics(self):
'''收集统计数据'''
def _collect_statistics():
while self.log_line_parsed_queue or not self.log_parsing_finished:
if not self.log_line_parsed_queue:
continue
log_info = self.log_line_parsed_queue.popleft()
# do something with log_info
with parallel_backend("multiprocessing", n_jobs=1):
Parallel()(delayed(_collect_statistics)() for i in range(1))
def run(self, file_path_list):
# 多线程读取日志文件
for file_path in file_path_list:
thread = threading.Thread(target=self.read_log_file,
name="read_log_file",
args=(file_path,))
thread.start()
self.files_read_list.append(file_path)
# 启动日志解析进程
thread = threading.Thread(target=self.start_processes_for_log_parsing, name="start_processes_for_log_parsing")
thread.start()
# 启动日志统计数据收集进程
thread = threading.Thread(target=self.collect_statistics, name="collect_statistics")
thread.start()
start = datetime.now()
while threading.active_count() > 1:
print('程序正在努力解析日志...')
time.sleep(0.5)
end = datetime.now()
print('解析完成', 'start', start, 'end', end, '耗时', end - start)
if __name__ == "__main__":
log_parser = LogParser()
log_parser.run(['access.log', 'access2.log'])
需要合理的配置单次读取文件数据块的大小,不能过大,或者过小,否则都可能会导致数据读取速度变慢。笔者实践环境下,发现10M~15M每次是一个比较高效的配置。
Recommend
About Joyk
Aggregate valuable and interesting links.
Joyk means Joy of geeK